This article has been reviewed according to Science X's editorial process and policies. Editors have highlighted the following attributes while ensuring the content's credibility:
fact-checked
peer-reviewed publication
trusted source
proofread
How AI tools can help assess verbal eyewitness statements
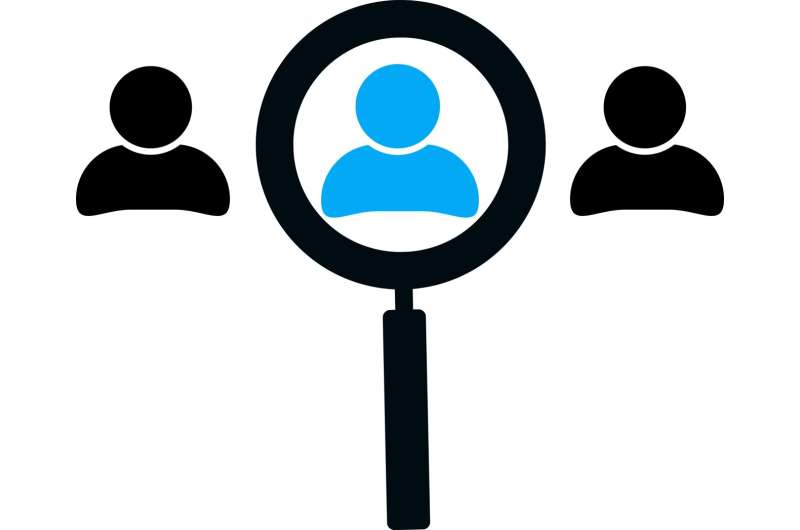
In a new paper in Psychological Science, researchers from the Quattrone Center for the Fair Administration of Justice at the University of Pennsylvania Carey Law School demonstrate how artificial intelligence (AI) can improve the accuracy of the criminal adjudication process.
The paper, "Assessing Verbal Eyewitness Confidence Statements Using Natural Language Processing," outlines how the researchers trained a large language model (LLM) to parse eyewitness confidence statements in an effort to help reduce misidentifications, one of the largest known contributors to wrongful convictions.
"Of the over 3,000 exonerations recorded to date by the National Registry of Exonerations, more than 900 are due to eyewitness misidentifications," says Paul Heaton, Academic Director of the Quattrone Center, Professor of Law, and co-author of the paper. "To help address this problem, we developed a new tool to enable attorneys and police investigators to better distinguish accurate identifications from faulty ones."
After an eyewitness selects a suspect from a lineup, best practices instruct police officers to ask how confident they are in their identification. Prior research demonstrates that witnesses who are highly confident at the time of the identification from an unbiased lineup tend to be correct much more frequently than those who express uncertainty. But in the field, police typically make a subjective judgment as to whether any particular witness was confident, which can introduce error into the process.
The AI developed by the researchers offers a neutral method for assessing such statements free of contextual bias, leveraging information from thousands of prior eyewitness decisions.
"Imagine if a witness makes an identification from a lineup and then states they are 'fairly certain' the person they selected is the person who committed the crime," says Rachel Leigh Greenspan, Assistant Professor of Criminal Justice and Legal Studies at the University of Mississippi and co-author of the paper.
"How might an officer or attorney interpret this confidence statement? Has this witness made a highly confident identification or not? Our LLM offers an objective, reproducible way to make these judgements, reducing the ambiguity of verbal confidence statements."
The goal of this model is to interpret the intended meaning of a witness' confidence statement when given in their own words. The LLM reads and classifies eyewitness confidence statements as high (75–100%), medium (26–74%), or low confidence (0–25%). To do this, researchers trained the model using a sample of witnesses who explained their confidence both in their own words and using numbers. They used the witness-provided numeric translations to identify the "ground-truth" of how confident witnesses actually are in their identification.
The researchers then tested the LLM's performance on data previously unseen by the model. Across a variety of lineup scenarios, the model correctly classified witness' level of confidence 71% of the time and performed similarly to trained human coders.
The tool's judgments also mapped to accuracy—for example, in one scenario analyzed by the authors, eyewitnesses making statements classified as high confidence by the LLM were correct nearly 90% of the time, versus only about a 50% success rate for low confidence identifications. The tool is freely available online for use by researchers and practitioners.
"These results have implications for how empirical scientists collect confidence data in the lab and how police interpret eyewitness confidence statements in the field," adds Alex Lyman, Penn graduate and Ph.D. student at Brigham Young University.
More information: Rachel Leigh Greenspan et al, Assessing Verbal Eyewitness Confidence Statements Using Natural Language Processing, Psychological Science (2024). DOI: 10.1177/09567976241229028
Journal information: Psychological Science
Provided by University of Pennsylvania