This article has been reviewed according to Science X's editorial process and policies. Editors have highlighted the following attributes while ensuring the content's credibility:
fact-checked
trusted source
proofread
Enhancing rice biomass estimation with UAV-based models
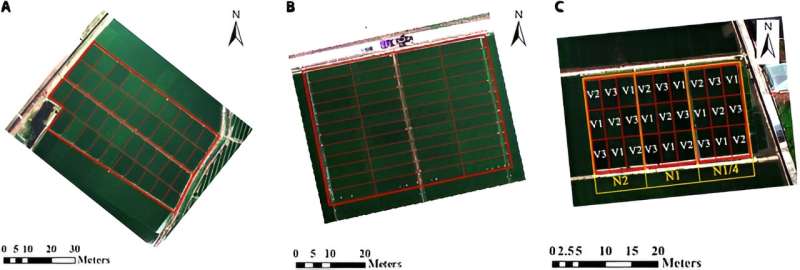
Aboveground biomass (AGB) of rice, vital for carbon pool and yield estimation, is traditionally measured through labor-intensive manual sampling. Recent advancements employ remote sensing, particularly unmanned aerial vehicles (UAVs), to derive vegetation indices (VIs) from plant interactions with the electromagnetic spectrum.
However, these methods face challenges: the non-linear VI-biomass relationship leads to saturation at high biomass levels, and sensitivity loss during later growth stages. Additionally, varying effectiveness across rice cultivars and computational demands of advanced machine learning models add complexity.
In May 2023, Plant Phenomics published a research article titled "Estimation of Rice Aboveground Biomass by UAV Imagery with Photosynthetic Accumulation Models," proposing a new model integrating VI and canopy height data from UAVs for more accurate and general biomass estimation throughout the rice growth season, addressing existing gaps in AGB monitoring.
In this study, initial findings revealed weak correlations between VI and AGB for the entire growing season, and limited accuracy in height models. However, the Photosynthetic Accumulation Model (PAM), combining NDVI and canopy height, significantly improved AGB estimation (R2 = 0.95, RMSE = 136.81 g/m2). Additionally, a Simplified Photosynthetic Accumulation Model (SPAM) was developed, requiring fewer observations while maintaining an R2 above 0.8.
Verification of these methods showed consistent accuracy in canopy height estimation across three years using consistent equipment and flight parameters. Remote sensing proved effective in capturing canopy height, correlating well with actual plant heights (R2 = 0.88, RMSE = 0.05 m). LAI estimation was examined using nine VIs, revealing variability in R2 and RMSE across years and indices.
The H × VI model outperformed individual VI models, reducing saturation and hysteresis effects. In biomass estimation, PAM utilized two different VIs, showing that any two from a set of six (NDVI, EVI2, WDRVI, NDRE, OSAVI, and GNDVI) yielded stable results over two years. The correlation between PAM and AGB was significantly positive, with R2 exceeding 0.8 in a two-year experiment.
SPAM's effectiveness was slightly lower than PAM but still outperformed traditional VI and height models. It demonstrated an improvement in estimation accuracy and a decrease in required observation frequency. Model transferability was tested with data collected at a different location in 2022, confirming PAM and SPAM's robustness and generalization ability. These models maintained better linear relationships with AGB across various rice cultivars throughout the growing season.
In conclusion, the study presents a reliable and efficient method for UAV-based estimation of rice AGB over the entire growing season. It offers a quantitative tool for evaluating rice growth and holds potential for large-scale field management and breeding. Future research aims to enhance model generality through multi-year experiments and broader rice cultivar sampling.
More information: Kaili Yang et al, Estimation of Rice Aboveground Biomass by UAV Imagery with Photosynthetic Accumulation Models, Plant Phenomics (2023). DOI: 10.34133/plantphenomics.0056
Provided by NanJing Agricultural University