This article has been reviewed according to Science X's editorial process and policies. Editors have highlighted the following attributes while ensuring the content's credibility:
fact-checked
proofread
Measuring and predicting collision cross section values for unknown compounds
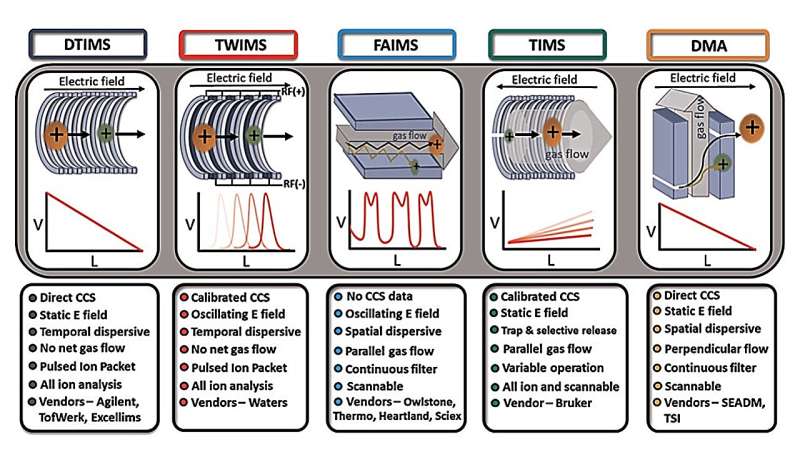
Researchers at Auburn University are seeking ways to identify unknown compounds using collision cross section (CCS) measurements paired with prediction methods including computational models and machine learning. Their study in the Journal of Mass Spectrometry discusses different types of technologies for the determination of CCS values.
Nowadays, there is a high demand for fast and reliable detection of compounds using untargeted analyses. This is especially true for the diagnosis of various diseases. Usually, the detected compounds can have a variety of different structures which will make the detection process challenging. Therefore, they can have different effects on living organisms, which is why research in "omics" (lipidomics, metabolomics, proteomics) has gained importance and is applied in many research areas such as environmental, food, clinical, etc.
Many separation techniques such as chromatography and mass spectrometry have been used to separate these structures from each other. However, the separation of structurally different compounds by these techniques is not always reliable for unknown substances. The reasons are that several structurally different compounds may have the same mass spectra and fragmentation patterns, and may also elute simultaneously in chromatography.
One of the separation techniques that can be used to solve these problems is ion mobility, as it separates the ionized compound based on its collision with a buffer gas under an electric field. Thus, it separates the ions based on their shape, size, and charge. In addition, ion mobility is a fast separation technique (~1 minute) that provides information about the CCS of a compound. CCS measurements are rotationally averaged surface area values that are unique to each compound, giving it a "fingerprint." In addition, CCS measurements are highly reproducible.
Although ion mobility can separate ions very well, it still has some limitations. Accurate experimental CCS determination in untargeted analysis usually requires reference CCS from standards. Since it is challenging to find appropriate standards, it can be difficult to obtain accurate experimental CCS values for different chemical classes. Therefore, theoretical CCS determination can help increase the confidence of experimental CCS measurements in untargeted analysis.
In recent decades, researchers have developed many methods for predicting CCS values based on machine learning and computer models. One of the first methods was the computational approach, which uses the 3D models of a compound. These approaches can be accurate, but they are also prone to larger errors depending on the structure of the compound of interest. They also require high computational power and typically take days to determine CCS values for many compounds in a batch.
On the contrary, the machine learning approach is faster in determining the CCS values and requires minimum computational power compared to the computational approach, and it yields an error of less than 10%. The limitations of the machine learning approach are that it depends on the available experimental CCS databases, the nature of the chemical classes, and the resolving power of the experimental CCS.
For example, if a CCS database constructed primarily with lipids is used to train the machine learning model, the error for the predicted CCS values of a protein compound will likely be higher. Therefore, acquiring and updating experimental databases with CCS from different chemical classes and higher resolving power (~300 and above) can improve the accuracy and error of predicted CCS values in the future through machine learning.
"By using ion mobility spectrometry (IMS), the Hamid Lab determined CCS values for compounds that will provide a foundation for measurements using portable ion mobility spectrometers in the future," said Ahmed M. Hamid, assistant professor in the Department of Chemistry and Biochemistry at Auburn University.
More information: Kimberly Y. Kartowikromo et al, Collision cross section measurement and prediction methods in omics, Journal of Mass Spectrometry (2023). DOI: 10.1002/jms.4973
Provided by Auburn University College of Sciences and Mathematics