Artificial intelligence and molecule machine join forces to generalize automated chemistry
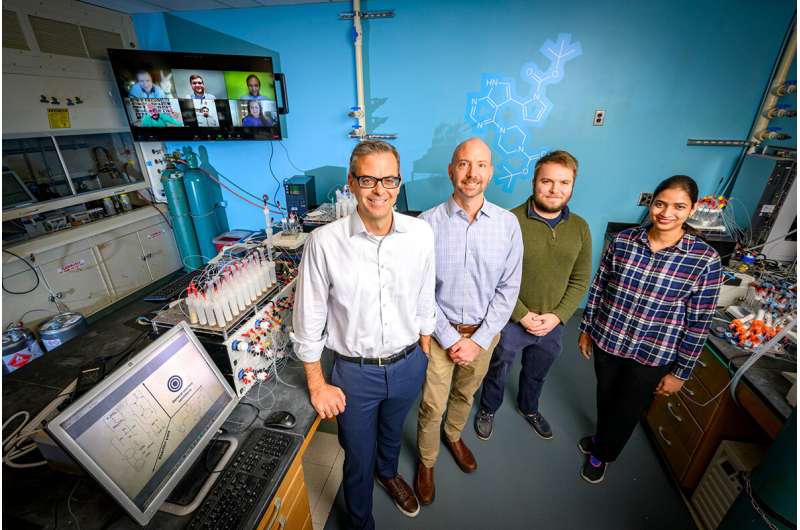
Artificial intelligence, "building-block" chemistry and a molecule-making machine teamed up to find the best general reaction conditions for synthesizing chemicals important to biomedical and materials research—a finding that could speed innovation and drug discovery as well as make complex chemistry automated and accessible.
With the machine-generated optimized conditions, researchers at the University of Illinois Urbana-Champaign and collaborators in Poland and Canada doubled the average yield of a special, hard-to-optimize type of reaction linking carbon atoms together in pharmaceutically important molecules. The researchers say their system provides a platform that also could be used to find general conditions for other classes of reactions and solutions for similarly complex problems. They reported their findings in the journal Science.
"Generality is critical for automation, and thus making molecular innovation accessible even to nonchemists," said study co-leader Dr. Martin D. Burke, an Illinois professor of chemistry and of the Carle Illinois College of Medicine, as well as a medical doctor. "The challenge is the haystack of possible reaction conditions is astronomical, and the needle is hidden somewhere inside. By leveraging the power of artificial intelligence and building-block chemistry to create a feedback loop, we were able to shrink the haystack. And we found the needle."
Automated synthesis machines for proteins and nucleic acids such as DNA have revolutionized research and chemical manufacturing in those fields, but many chemicals of importance for pharmaceutical, clinical, manufacturing and materials applications are small molecules with complex structures, the researchers say.
Burke's group has pioneered the development of simple chemical building blocks for small molecules. His lab also developed an automated molecule-making machine that snaps together the buildings blocks to create a wide range of possible structures.
However, general reaction conditions to make the automated process broadly applicable have remained elusive.
"Traditionally, chemists customize the reaction conditions for each product they are trying to make," Burke said. "The problem is that this is a slow and very specialist-dependent process, and very hard to automate because the machine would have to be optimized every time. What we really want are conditions that work almost every time, no matter what two things you're trying to snap together."
An automated approach with generalized conditions could help standardize how some products are made, addressing the problem of reproducibility, said Illinois postdoctoral researcher Vandana Rathore, a co-first author of the study.
Burke's group teamed up with a group led by Bartosz A. Grzybowski at the Polish Academy of Sciences' Institute for Organic Chemistry, as well as the group of Alán Aspuru-Guzik at the University of Toronto, both leaders in using artificial intelligence and machine learning to improve chemical synthesis. The team integrated AI with the molecule machine to provide real-time feedback to the machine-learning system.
"To distinguish good and bad you need to know something about the bad, but people only publish the successes," Grzybowski said. Published studies reflect conditions that are popular or convenient, rather than the best, so a systematic approach that included diverse data and negative results was necessary, he said.
First, the team ran the entire matrix of possible combinations using the building-block chemistry through an algorithm to group together similar reactions. Then, the AI sent instructions, inputted to a machine in the Molecule Maker Lab located in the Beckman Institute for Advanced Science and Technology at Illinois, to produce representative reactions from each cluster. The information from those reactions fed back into the model; the AI learned from the data and ordered more experiments from the molecule machine.
"We were looking to see two things: an increase in yield and a decrease in uncertainty, for a broad spectrum of reactions," said Grzybowski, who now is at Ulsan Institute of Science and Technology in South Korea. "This loop continued without us having to intervene until the problem was solved. Figuring out the generalized conditions for protein-synthesis machines took 30 years. This took us two months."
The process identified conditions that doubled the average yield of a challenging class of reactions, called heteroaryl Suzuki-Miyaura coupling, crucial for many biological and materials-relevant compounds.
"There are all kinds of building block combinations that we didn't even study in our AI training, but because the AI had explored such a diverse space, it found good results even in those initially unexplored areas," said Illinois graduate student Nicholas H. Angello, a co-first author of the study.
The machine-learning process described in the paper also could be applied to other broad areas of chemistry to find the best reaction conditions for other types of small molecules or even larger organic polymers, the researchers say.
"There are so many different materials classes that we want to know, target and discover for different functional properties. The extension possibility of this approach to other similar reaction chemistry, other types of carbon-carbon links, is exciting," said study co-author Charles M. Schroeder, an Illinois professor of materials science and engineering and chemical and biomolecular engineering, and a Beckman Institute affiliate.
More information: Nicholas H. Angello et al, Closed-loop optimization of general reaction conditions for heteroaryl Suzuki-Miyaura coupling, Science (2022). DOI: 10.1126/science.adc8743
Journal information: Science
Provided by University of Illinois at Urbana-Champaign