Robust and realistic general method for dealing with wind-driven phenomena
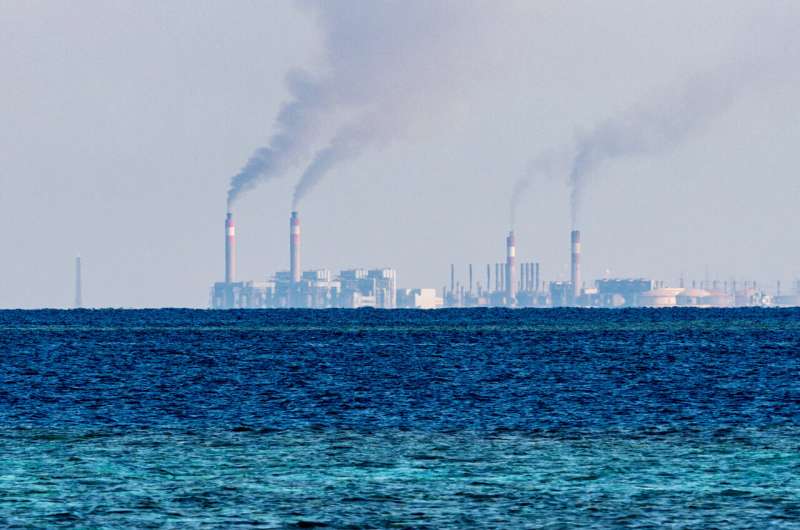
By adapting a flow-following physical framework to the statistical modeling of large spatio–temporal datasets, KAUST researchers have developed a more robust and realistic general method for dealing with wind-driven phenomena. The approach promises to greatly improve the accuracy of pollutant dispersion prediction by incorporating more physically realistic processes into geostatistical modeling.
Geostatistical analyses involve the statistical processing of very large datasets, such as measurements of wind speed at many locations and altitudes over time, to extract an underlying model of how certain parameters behave and are correlated spatially and temporally in the real world. However, the ability of such models to accurately characterize that behavior and predict what happens next largely depends on the model framework used for analysis. A team of KAUST scientists led by Marc Genton has been developing more physically meaningful analytical frameworks that can better model such natural phenomena.
"Many space-time geostatistical models do not necessarily reflect fundamental scientific relationships," explains Mary Salvaña, who worked with Genton and Amanda Lenzi on the research. "There is demand for space–time geostatistical models with a physics basis, as most environmental data obey various fundamental laws of nature. In this study, we took a modeling concept in physics called the Lagrangian framework and formulated it in the language of space–time multivariate geostatistics to develop a suite of data-driven space–time models that are more appropriate for datasets involving transport by media, such as wind."
Wind is a complicated driving phenomenon to incorporate into a practical statistical model. It is asymmetric in its correlation, flowing from one place to another, and also varies by altitude. The Lagrangian framework was developed in the field of fluid dynamics to model flows in a way that is analogous to the underlying physics by following a fluid parcel as it moves through space and time. For Salvaña and her colleagues, the challenge was to ensure that this framework could be validly used with a space–time geostatistics model across multiple variables.
"Our results, which confirmed the validity of the model, showed that failing to account for multiple advections or transport phenomena can lead to poor predictions," says Salvaña.
The team demonstrated their model by applying it to a bivariate pollutant dataset of particulate matter across Saudi Arabia. The results showed that black carbon distributions are much more accurately modeled taking altitude-dependent wind behavior into account.
"Our modeling framework could also be applied to the study of space–time correlation of ocean variables, since water is another transport medium, which could be important for understanding ocean patterns before and after a tropical cyclone," Salvaña says.
The study is published in the Journal of the American Statistical Association.
More information: Mary Lai O. Salvaña et al, Spatio-Temporal Cross-Covariance Functions under the Lagrangian Framework with Multiple Advections, Journal of the American Statistical Association (2022). DOI: 10.1080/01621459.2022.2078330