Machine learning algorithms help scientists explore Mars
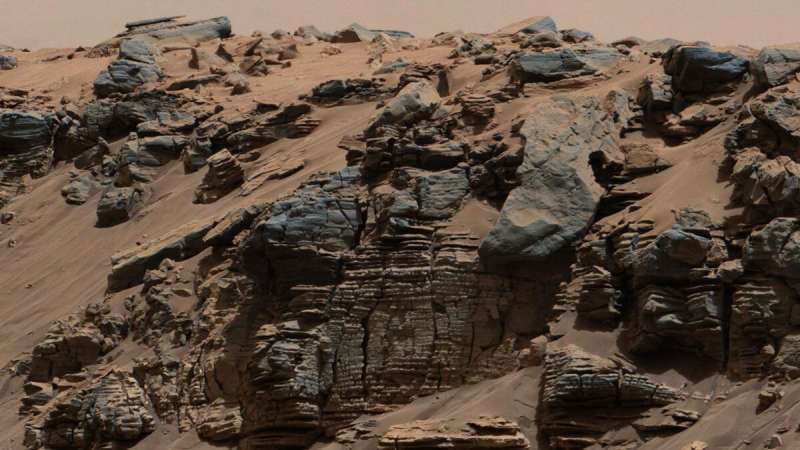
NASA's Curiosity rover has been exploring the Red Planet's surface for nearly a decade, with its main mission being to determine whether Mars was once habitable. While the rover's investigations have indeed confirmed that Mars was once a watery world filled with potentially life-sustaining chemistry, there's still much to learn. Curiosity's mountains of data offer an opportunity to use machine learning algorithms to investigate the planet's surface in even more detail.
A new article in Earth and Space Science focuses on the data collected by Curiosity's Chemistry and Camera (ChemCam) instrument package. ChemCam combines two instruments: a laser-induced breakdown spectrometer (LIBS) and a remote micro-imager (RMI) for high-resolution imaging. ChemCam's LIBS instrument works by blasting rock samples with powerful laser pulses, which leads the material to evolve into a microplasma with excited atoms and ions that emit characteristic light while decaying. Because each element emits a unique light spectrum as it decays, ChemCam can collect the light in three different spectrometers, allowing scientists to determine the chemical makeup of each sample.
Since Curiosity landed in Gale crater in 2012, ChemCam has collected more than 800,000 individual spectra from more than 2,500 samples. Yet using machine learning to investigate ChemCam's data remains challenging because of the lack of training data sets from Mars, according to Rammelkamp and colleagues.
In their new paper, the authors used an unsupervised machine learning algorithm to classify ChemCam's data from the first 2,756 sols (about 7 years) of Curiosity's exploration of Gale crater. Since the rover landed, it has traversed several distinct geological regions. The algorithm sorted ChemCam's data into six distinct groups of chemical compositions, which include high and low silicon dioxide, felsic (high aluminum oxide and alkalis), high and low calcium oxide, and high total iron oxides.
The results reveal transitions between the geologically different regions along Curiosity's traverse since leaving the landing site. The results were obtained using only LIBS data, and no training data were required. Furthermore, the six distinct groups serve as training data for supervised machine learning algorithms, which was shown to be exemplary with a so-called random forest classifier.
Machine learning algorithms like this one can serve as a powerful tool to map Mars's surface on a large scale, according to the authors. This ability will become increasingly useful as more rovers explore the Red Planet, such as NASA's Perseverance rover, which landed in February 2021 (and has already been collecting its own LIBS data from Jezero crater with ChemCam's successor instrument, SuperCam), and China's Zhurong rover, which landed in May.
More information: Kristin Rammelkamp et al, Clustering Supported Classification of ChemCam Data From Gale Crater, Mars, Earth and Space Science (2021). DOI: 10.1029/2021EA001903
Provided by Eos
This story is republished courtesy of Eos, hosted by the American Geophysical Union. Read the original story here.