Improved statistical methods for high-throughput omics data analysis
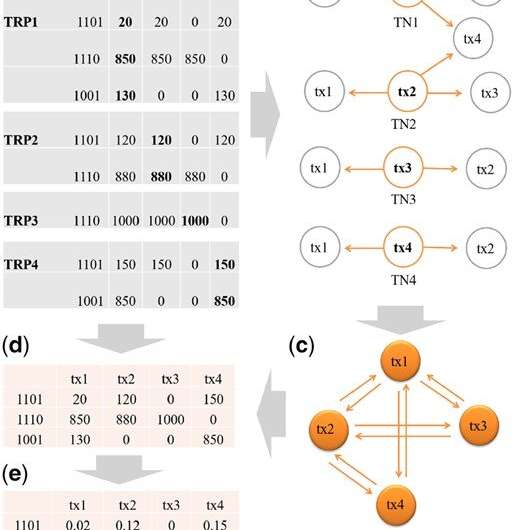
High-throughput omics technology has revolutionized biological and biomedical research and large volumes of omics data have been produced. For this, computational tools to manage and analyze the omics data have been developed and there are big challenges in how to process and interpret the omics data in the best way. Wenjiang Deng has worked to develop novel statistical methodologies and algorithms for omics data analysis, using both simulated and real cancer data to test the methods.
Could you describe some of the results in your thesis?
Yes, in my first study, we identify several genes associated with the survival of high-risk neuroblastoma patients, says Wenjiang Deng, Ph.D. student at the Department of medical epidemiology and biostatistics, MEB. Neuroblastoma is the most common and deadliest cancer in young kids under the age of five. We believe that our findings will provide significant evidence for the treatment and management of patients. Our results can also be meaningful to understand the physiological mechanisms of the disease.
How come you chose to study this particular area?
We are living in the era of "big data," and the high-throughput sequencing data is the predominant "big data" in life science. When I first heard the concept of omics data, I was amazed by its huge volume and the big potential in medical research. Nowadays it is quite easy to produce sequencing data, but we still need efficient and accurate tools to analyze them, so I decided to study the development of algorithms during my time as a Ph.D. student.
What will you do next?
After my defense, I will stay in MEB for a while to wrap up my manuscripts. I will then go to Shenzhen, China, and start working in a biotechnology company which aims to develop new methods for early diagnosis of cancers. I hope that our work there will contribute to the overall health of human beings.
More information: Wenjiang Deng et al, Alternating EM algorithm for a bilinear model in isoform quantification from RNA-seq data, Bioinformatics (2019). DOI: 10.1093/bioinformatics/btz640
Journal information: Bioinformatics
Provided by Karolinska Institutet