Deep learning and metamaterials make the invisible visible
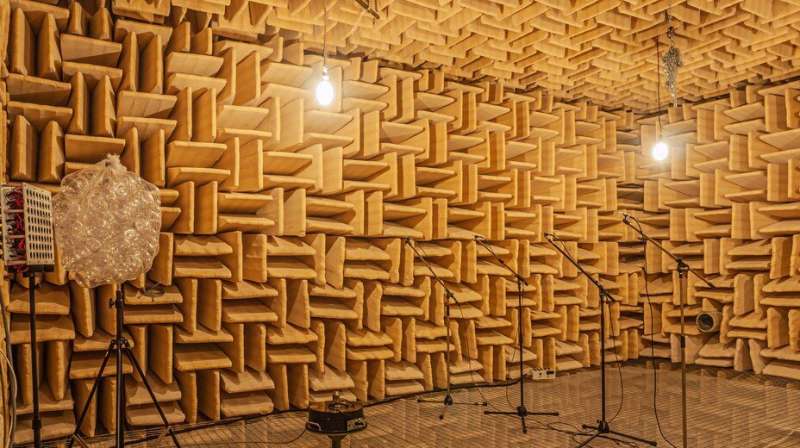
By combining purpose-built materials and neural networks, researchers at EPFL have shown that sound can be used in high-resolution imagery.
Imaging allows us to depict an object through far-field analysis of the light- and sound-waves that it transmits or radiates. The shorter the wave, the higher the image's resolution. However, the level of detail is limited by the size of the wavelength in question—until now. Researchers at EPFL's Laboratory of Wave Engineering have successfully proven that a long, and therefore imprecise, wave (in this case a sound wave) can elicit details that are 30 times smaller than its length. To achieve this, the research team used a combination of metamaterials—specifically-engineered elements—and artificial intelligence. Their research, which has just been published in Physical Review X, is creating exciting new possibilities, particularly in the fields of medical imaging and bioengineering.
The team's groundbreaking idea was to bring together two separate technologies that have previously pushed the boundaries of imaging. One of these is metamaterials: purpose-built elements that can, for example, focus wavelengths precisely. That said, they are known to lose their effectiveness by haphazardly absorbing signals in a way that makes them difficult to decipher. The other is artificial intelligence, or more specifically neural networks that can quickly and efficiently process even the most complex information, although there is a learning curve involved.
To exceed what is known in physics as the diffraction limit, the research team—headed by Romain Fleury—conducted the following experiment: they first created a lattice of 64 miniature speakers, each of which could be activated according to the pixels in an image. Then they used the lattice to reproduce sound images of numerals from zero to nine with extremely precise spatial details; the images of numerals fed into the lattice were drawn from a database of some 70,000 handwritten examples. Across from the lattice the researchers placed a bag containing 39 Helmholtz resonators (10 cm spheres with a hole at one end) that formed a metamaterial. The sound produced by the lattice was transmitted by the metamaterial and captured by four microphones placed several meters away. Algorithms then deciphered the sound recorded by the microphones in order to learn how to recognize and redraw the original numeral images.
An advantageous drawback
The team achieved a nearly 90% success rate with their experiment. "By generating images with a resolution of only a few centimeters—using a sound wave whose length was approximately a meter—we moved well past the diffraction limit," says Romain Fleury. "In addition, the tendency of metamaterials to absorb signals, which had been considered a major disadvantage, turns out to be an advantage when neural networks are involved. We found that they work better when there is a great deal of absorption."
In the field of medical imaging, using long waves to see very small objects could be a major breakthrough. "Long waves mean that doctors can use much lower frequencies, resulting in acoustic imaging methods that are effective even through dense bone tissue. When it comes to imaging that uses electromagnetic waves, long waves are less hazardous to a patient's health. For these types of applications, we wouldn't train neural networks to recognize or reproduce numerals, but rather organic structures," says Fleury.
More information: Bakhtiyar Orazbayev et al. Far-Field Subwavelength Acoustic Imaging by Deep Learning, Physical Review X (2020). DOI: 10.1103/PhysRevX.10.031029
Journal information: Physical Review X
Provided by Ecole Polytechnique Federale de Lausanne