Numerical model pinpoints source of precursor to seismic signals
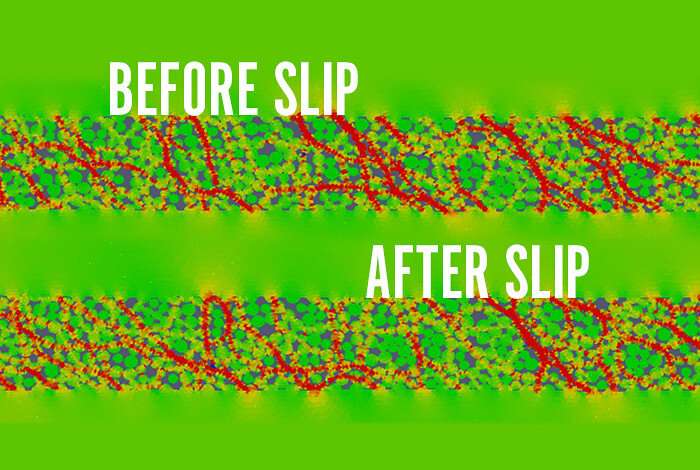
Numerical simulations have pinpointed the source of acoustic signals emitted by stressed faults in laboratory earthquake machines. The work further unpacks the physics driving geologic faults, knowledge that could one day enable accurately predicting earthquakes.
"Previous machine-learning studies found that the acoustic signals detected from an earthquake fault can be used to predict when the next earthquake will occur," said Ke Gao, a computational geophysicist in the Geophysics group at Los Alamos National Laboratory. "This new modeling work shows us that the collapse of stress chains inside the earthquake gouge emits that signal in the lab, pointing to mechanisms that may also be important in Earth." Gao is lead author of the paper, "From Stress Chains to Acoustic Emission," published today in Physical Review Letters and selected as the "Editors' Suggestion."
Stress chains are bridges composed of grains that transmit stresses from one side of a fault block to the other.
Gao works on a Los Alamos team that has identified the predictive acoustic signal in data from both laboratory quakes and megathrust regions in North America, South America and New Zealand. The signal accurately indicates the state of stress in the fault, no matter when the signal is read.
"Using the numerical model that we developed at Los Alamos, we examine and connect the dynamics in a granular system of fault gouge to signals detected on passive remote monitors," Gao said. Fault gouge is the ground-up, gravelly rock material created by the stresses and movements of a fault.
To investigate the cause of acoustic signals, the team conducted a series of numerical simulations on supercomputers using the Los Alamos-developed code HOSS (Hybrid Optimization Software Suite). This novel numerical tool is a hybrid methodology—the combined finite-discrete element method. It merges techniques developed under discrete element methods, to describe grain-to-grain interactions; and under finite element methods, to describe stresses as a function of deformation within the grains and wave propagation away from the granular system. The simulations accurately mimic the dynamics of earthquake fault evolution, such as how the materials inside the gouge grind and collide with each other, and how the stress chains form and evolve over time via interactions between adjacent gouge materials.
Los Alamos has funded a multi-million-dollar, multi-year program consisting of experiments, numerical modeling, and machine-learning efforts to develop and test a highly novel approach to probe the earthquake cycle and, in particular, to detect and locate stressed faults that are approaching failure.
More information: Ke Gao et al. From Stress Chains to Acoustic Emission, Physical Review Letters, 26-July, 2019, Volume: 123, EID: 048003, DOI: 10.1103/PhysRevLett.123.048003
Journal information: Physical Review Letters
Provided by Los Alamos National Laboratory