Sentiment analysis for portfolio management
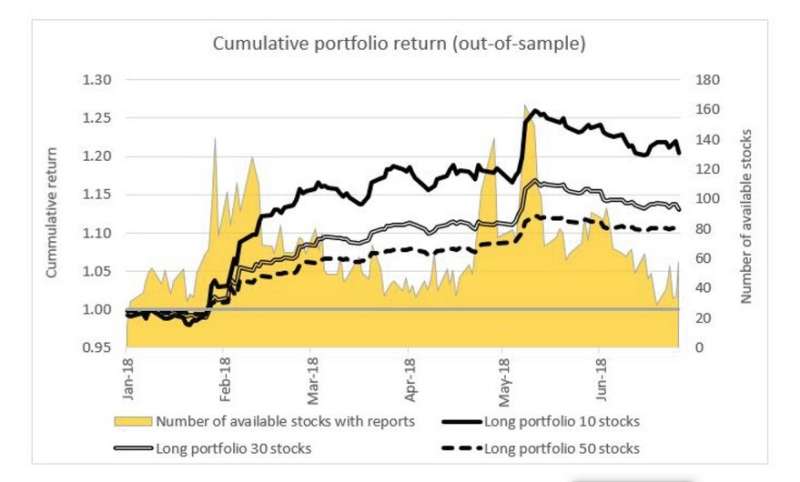
NUS data scientists have developed a deep learning-based text data analytics method to extract sentiment information from analyst reports for investment decisions.
Unstructured text is one of the largest data sources which is used to communicate investor thoughts and opinions in financial markets. Text data analytics transform these unstructured text data into meaningful data that can provide insights, such as stock market trading patterns. There is increasing demand in the financial sector to utilise text information to guide decision makers in making better investment decisions.
Prof Chen Ying from the Department of Mathematics, NUS and her Ph.D. student Mr Hitoshi IWASAKI have developed a text data analytics method for extracting sentiment indices for specific topics from analyst reports of listed companies. This sentiment extraction, which is based on a machine learning approach, is named as deep neural network supervised learning. A key feature of this method is that it analyses the reports at the sentence level rather than individual words. In this way, the original meaning and context can be more accurately identified.
The research team performed the sentiment analysis on more than 110,000 analyst reports written in Japanese for stocks listed on the Tokyo Stock Exchange and the Osaka Exchange. The sentiments are then incorporated into a topic sentiment asset pricing model. Compared to other asset pricing models which either do not incorporate sentiment analysis or have overall sentiments (single aggregated value), their model was shown to have better predictability on expected returns and improved interpretability (contribution of each variable component towards the outcome).
Prof Chen said, "In our study, we found that topics reflecting the subjective opinions of equity analysts have greater predictability on portfolio returns than topics pertaining to objective facts and quantitative measures. This seems to suggest that sentiment analysis could play a significant role in modern portfolio selection."
More information: Hitoshi Iwasaki et al. Topic Sentiment Asset Pricing with DNN Supervised Learning, SSRN Electronic Journal (2018). DOI: 10.2139/ssrn.3228485
Provided by National University of Singapore