Make deep learning faster and simpler
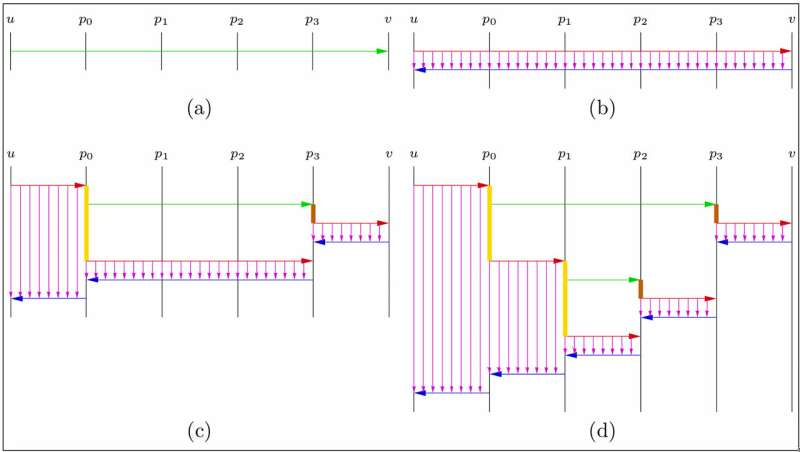
Artificial intelligence systems based on deep learning are changing the electronic devices that surround us.
The results of this deep learning is something seen each time a computer understands our speech, we search for a picture of a friend or we see an appropriately placed ad. But the deep learning itself requires enormous clusters of computers and weeklong runs.
"Methods developed by our international team will reduce this burden," said Jeffrey Mark Siskind, professor of electrical and computer engineering in Purdue's College of Engineering. "Our methods allow individuals with more modest computers to do the kinds of deep learning that used to require multimillion dollar clusters, and allow programmers to write programs in hours which used to require months."
Deep learning uses a particular kind of calculus at its heart: a clever technique, called automatic differentiation (AD) in the reverse accumulation mode, for efficiently calculating how adjustments to a large number of controls will affect a result.
"Sophisticated software systems and gigantic computer clusters have been built to perform this particular calculation," said Barak Pearlmutter, professor of computer science at Maynooth University in Ireland, and the other principal of this collaboration. "These systems underlie much of the AI in society: speech recognition, internet search, image understanding, face recognition, machine translation and the placement of advertisements."
One major limitation on these deep learning systems is that they support this particular AD calculation very rigidly.
"These systems only work on very restricted kinds of computer programs: ones that consume numbers on their input, perform the same numeric operations on them regardless of their values, and output the resulting numbers," Siskind said.
The researchers said another limitation is that the AD operation requires a great deal of computer memory. These restrictions limit the size and sophistication of the deep learning systems that can be built. For example, they make it difficult to build a deep learning system that performs a variable amount of computation depending on the difficulty of the particular input, one that tries to anticipate the actions of an intelligent adaptive user, or one that produces as its output a computer program.
Siskind said the collaboration is aimed at lifting these restrictions.
A series of innovations allows not just reverse-mode AD, but other modes of AD, to be used efficiently; for these operations to be cascaded, and applied not just to rigid computations but also to arbitrary computer programs; for increasing the efficiency of these processes; and for greatly reducing the amount of required computer memory.
"Usually these sorts of gains come at the price of increasing the burden on computer programmers," Siskind said. "Here, the techniques developed allow this increased flexibility and efficiency while greatly reducing the work that computer programmers building AI systems will need to do."
For example, a technique called "checkpoint reverse AD" for reducing the memory requirements was previously known, but could only be applied in limited settings, was very cumbersome, and required a great deal of extra work from the computer programmers building the deep learning systems.
One method developed by the team allows the reduction of memory requirements to apply to any computer program, and requires no extra work from the computer programmers building the AI systems.
"The massive reduction in RAM required for training AI systems should allow more sophisticated systems to be built, and should allow machine learning to be performed on smaller machines – smart phones instead of enormous computer clusters," Siskind said.
As a whole, this technology has the potential to make it much easier to build sophisticated deep-learning-based AI systems.
"These theoretical advances are being built into a highly efficient full-featured implementation which runs on both CPUs and GPUs and supports a wide range of standard components used to build deep-learning models," Siskind said.
Provided by Purdue University