Can artificial intelligence tell a polar bear from a can opener?
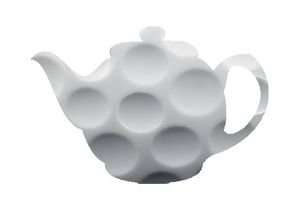
How smart is the form of artificial intelligence known as deep learning computer networks, and how closely do these machines mimic the human brain? They have improved greatly in recent years, but still have a long way to go, a team of UCLA cognitive psychologists reports in the journal PLOS Computational Biology.
Supporters have expressed enthusiasm for the use of these networks to do many individual tasks, and even jobs, traditionally performed by people. However, results of the five experiments in this study showed that it's easy to fool the networks, and the networks' method of identifying objects using computer vision differs substantially from human vision.
"The machines have severe limitations that we need to understand," said Philip Kellman, a UCLA distinguished professor of psychology and a senior author of the study. "We're saying, 'Wait, not so fast.'"
Machine vision, he said, has drawbacks. In the first experiment, the psychologists showed one of the best deep learning networks, called VGG-19, color images of animals and objects. The images had been altered. For example, the surface of a golf ball was displayed on a teapot; zebra stripes were placed on a camel; and the pattern of a blue and red argyle sock was shown on an elephant. VGG-19 ranked its top choices and chose the correct item as its first choice for only five of 40 objects.
"We can fool these artificial systems pretty easily," said co-author Hongjing Lu, a UCLA professor of psychology. "Their learning mechanisms are much less sophisticated than the human mind."
VGG-19 thought there was a 0 percent chance that the elephant was an elephant and only a 0.41 percent chance the teapot was a teapot. Its first choice for the teapot was a golf ball, which shows that the artificial intelligence network looks at the texture of an object more so than its shape, said lead author Nicholas Baker, a UCLA psychology graduate student.
"It's absolutely reasonable for the golf ball to come up, but alarming that the teapot doesn't come up anywhere among the choices," Kellman said. "It's not picking up shape."
Humans identify objects primarily from their shape, Kellman said. The researchers suspected the computer networks were using a different method.
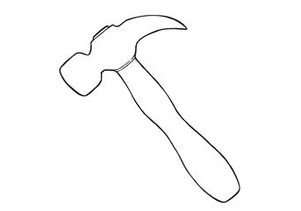
In the second experiment, the psychologists showed images of glass figurines to VGG-19 and to a second deep learning network, called AlexNet. VGG-19 performed better on all the experiments in which both networks were tested. Both networks were trained to recognize objects using an image database called ImageNet.
However, both networks did poorly, unable to identify the glass figurines. Neither VGG-19 nor AlexNet correctly identified the figurines as their first choices. An elephant figurine was ranked with almost a 0 percent chance of being an elephant by both networks. Most of the top responses were puzzling to the researchers, such as VGG-19's choice of "website" for "goose" and "can opener" for "polar bear." On average, AlexNet ranked the correct answer 328th out of 1,000 choices.
"The machines make very different errors from humans," Lu said.
In the third experiment, the researchers showed 40 drawings outlined in black, with images in white, to both VGG-19 and AlexNet. These first three experiments were meant to discover whether the devices identified objects by their shape.
The networks again did a poor job of identifying such items as a butterfly, an airplane and a banana.
The goal of the experiments was not to trick the networks, but to learn whether they identify objects in a similar way to humans, or in a different manner, said co-author Gennady Erlikhman, a UCLA postdoctoral scholar in psychology.
In the fourth experiment, the researchers showed both networks 40 images, this time in solid black.
With the black images, the networks did better, producing the correct object label among their top five choices for about 50 percent of the objects. VGG-19, for example, ranked an abacus with a 99.99 percent chance of being an abacus and a cannon with a 61 percent chance of being a cannon. In contrast, VGG-19 and AlexNet each thought there was less than a 1 percent chance that a white hammer (outlined in black) was a hammer.
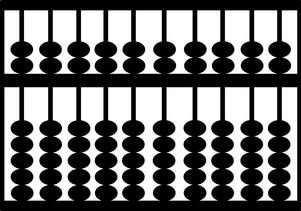
The researchers think the networks did much better with the black objects because the items lack what Kellman calls "internal contours"—edges that confuse the machines.
In experiment five, the researchers scrambled the images to make them more difficult to recognize, but they preserved pieces of the objects. The researchers selected six images the VGG-19 network got right originally, and scrambled them. Humans found these hard to recognize. VGG-19 got five of the six images right, and was close on the sixth.
As part of the fifth experiment, the researchers tested UCLA undergraduate students, in addition to VGG-19. Ten students were shown objects in black silhouettes—some scrambled to be difficult to recognize and some unscrambled, some objects for just one second, and some for as long as the students wanted to view them. The students correctly identified 92 percent of the unscrambled objects and 23 percent of the scrambled ones with just one second to view them. When the students could see the silhouettes for as long as they wanted, they correctly identified 97 percent of the unscrambled objects and 37 percent of the scrambled objects.
What conclusions do the psychologists draw?
Humans see the entire object, while the artificial intelligence networks identify fragments of the object.
"This study shows these systems get the right answer in the images they were trained on without considering shape," Kellman said. "For humans, overall shape is primary for object recognition, and identifying images by overall shape doesn't seem to be in these deep learning systems at all."
There are dozens of deep learning machines, and the researchers think their findings apply broadly to these devices.
More information: Nicholas Baker et al. Deep convolutional networks do not classify based on global object shape, PLOS Computational Biology (2018). DOI: 10.1371/journal.pcbi.1006613
Journal information: PLoS Computational Biology
Provided by University of California, Los Angeles