AI can help retailers understand the consumer
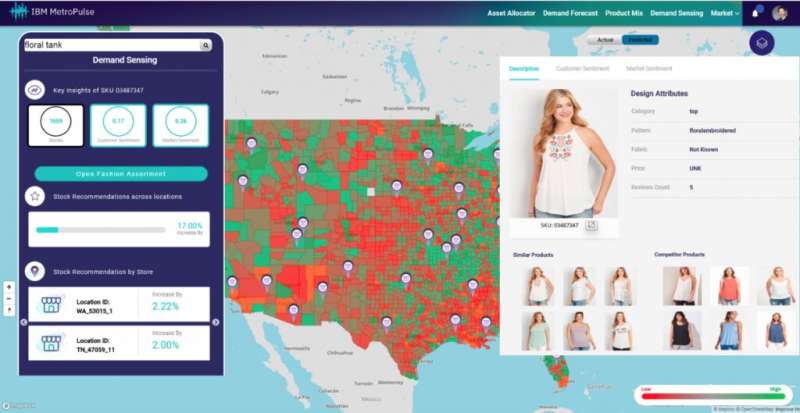
Consumer brands and retailers often struggle to fully understand ever-changing customer needs. That is why you mostly find XL sizes in your favorite fashion store and no M sizes. That is why you have to spend hours looking for the style you saw on Instagram and still not find it. That is why the cost of dead inventory to fashion retailers in the US alone is an estimated to be a whopping USD 50 billion. And that is part of the reason why the US generated 16 million tons of textile waste in 2014.
This is not because of any lack of intention or effort in the industry; rather, it is extremely difficult to understand consumers at scale. Characterizing consumers with broad brush definitions of age, gender and income is not effective given diverse and ever-changing consumer preferences, and retailers now need to look at much finer market segments—even down to single individuals. Increasingly, consumers are driving trends rather than merchants defining them, and this goes hand in hand with much more experimentation and disruption in the market.
To create and sell the "next big thing" in such a dynamic environment, designers, buyers and merchandisers must use their own creativity but also consider, with unprecedented granularity, how consumer preferences are changing and how different design, merchandising and marketing choices will perform. This is where AI and automation come in.
For example, consider a fashion retail buyer. She is responsible for the financial success of the merchandise she selects in any given season, but it's impossible for her to predict the performance of any design 12 months before the target season, or to identify the best promotional interventions to apply in-season. This is because she has very little visibility into how consumer preferences are changing across her stores over time, and how competing products are performing in the market.
Imagine an AI driven system that could analyze the natural language text of millions of online customer reviews and the images of all products in the market to summarize key relationships between location-specific customer sentiment and product features. For instance, how customers are responding to color block pullovers in Kansas City versus in Buffalo, and what attribute is the likely cause of lower customer sentiment for her color block pullovers versus competing color block pullovers. Such information for her in-market products and new planned products would help her dramatically improve her assortment, pricing and markdown, and marketing plans.
Market sentiment computed for visually similar floral tops across three different brands. The dashboard shows consumer preference for this type of product in different parts of the country, and recommended stock interventions at different stores.
Similarly, consider a sales manager for a yogurt brand. With a system that could analyze cross-brand sales of foods across the country to produce high quality predictions of the demand for the spinach artichoke flavored yogurt produced by the company, the sales manager then could negotiate product introductions and planograms with retailers. A majority of such negotiations fail today in the absence of such capability.
In fact, IBM's recent study of more than 1,900 retail and consumer product leaders shows that the adoption of intelligent automation in the retail and consumer products industries is projected to balloon from 40 percent of companies today to more than 80 percent in three years.
Our team at IBM Research – India collaborated with the IBM MetroPulse team to bring such first-of-a-kind, AI-driven capabilities to MetroPulse, an industry platform that brings together voluminous market, external and client datasets. The new capabilities use AI and automation to fuse these structured and unstructured datasets around semantic, visual and location contexts and uncovers fine-grained insights about customer preferences hidden in this fused data. These insights will help consumer brands and retailers make smarter choices about product design, inventory planning, demand forecasting and product assortment that are in tune with dynamic consumer preferences.
The platform has three layers, each with deep industry content:
The data layer, which consists of
- Market data containing up to date, location-specific signals of consumer preferences, product landscape and brand/retailer behavior. Some examples of datasets we curate include online customer reviews and comments, point of sales data and product images. The analysis of these large data sets can give companies clues as to how consumer preferences are changing across brands, retailers, cultures and regions – at the city or neighborhood level.
- Hyper-local third-party data continuously updated, neighborhood-level signals of external factors that influence consumer behavior such as demographics, weather forecasts and history, local events and visitors.
- Private enterprise data containing information about the retailer's own stores, products, merchandising, promotions and sales history. This data is treated with high security and privacy guarantees.
Incorporating such multiple data sets is critical to getting demand sensing and forecasting right, as also noted in Supply Chain Management 2018: In Service of The Customer, Retail Systems Research, Dec 2018 where 60 – 70 percent of respondents see "a lot of value" from considering new data such as sentiment, trade area data and past promotions into demand forecasting.
The knowledge layer, which consists of
- A retail-industry specific digital vocabulary – knowledge graphs that capture industry information in the form of entities, properties and relationships. This layer allows the data in the data layer to be interpreted and analyzed in a standard and meaningful way by the AI systems that ultimately generate insights for end users. For example, consider a fashion taxonomy that captures various fashion terms and the relationships between them (e.g. "peplum" is type of "top"), or a grocery ontology that captures various food types, ingredients, flavors and type hierarchy.
The industry intelligence layer, which consists of
- A variety of AI algorithms and models that can identify and understand the signals buried in the data, turning them into insights and recommendations exposed in a meaningful and standardized way via a dashboard and APIs. These insights and recommendations help business leaders, product designers, merchandisers and other enterprise users to understand and optimize for the behaviors and preferences of their target consumer base. Shopper-facing solutions for personalization and cognitive assistance can also consume these APIs for enhanced consumer engagement at the point of sale. These algorithms leverage the latest AI techniques in multimodal AI, explainable AI and forecasting, and tune them to understand industry-specific knowledge and concepts.
- Multimodal AI brings together visual perception and natural language processing to extract insights from multiple modalities of data. For instance, it may identify fashion objects in an image and relate them to expressions of customer opinion in accompanying reviews; or a semantic similarity model that understands that apple juice is more similar to lemonade than to apple for a consumer, but close to apple juice in terms of flavor and ingredients.
- Explainable AI explains why a model generates a particular output for a given input. As AI models have gotten more complex, it has become nearly impossible for non-data scientists to understand their behavior, making it difficult for them to rely on the predictions from the models. tour techniques bring that explainability and help end users understand the "why." For example, understand the sentiment for different design aspects of summer dresses in the market and explain the contribution of local factors (demographics, weather) and merchandising factors (price, stock, promotion) on the variation of sentiment across the counties of the US.
You can try out these new MetroPulse capabilities with real-world data at the National Retail Federation (NRF) event in New York City in January, 2019, or see here for further details.
Provided by IBM
This story is republished courtesy of IBM Research. Read the original story here.