Optimizing e-mobility use for everyday life
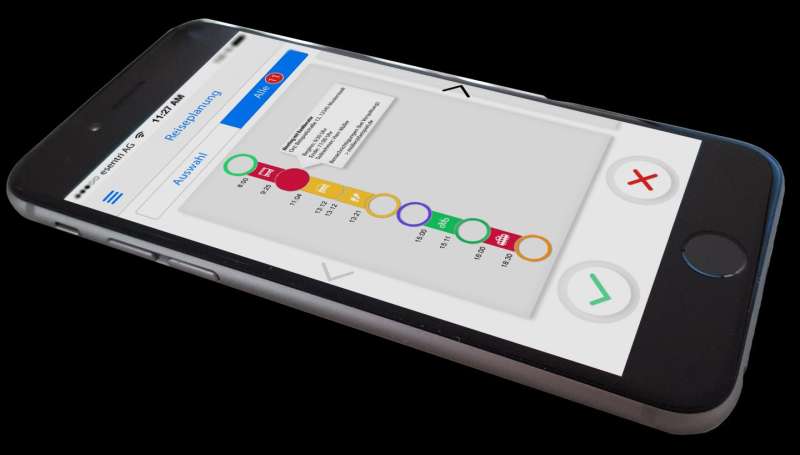
People choose different transportation methods for work commutes, leisure activities and running errands. Each activity in daily life might require a different form of mobility, and sometimes it makes sense to link some appointments. In the future, the optimum solution could be suggested by a mobility app. A prototype of an electronic assistant for mobility customers and other tools for providers of mobility services were recently presented by the BiE project.
Mobility behavior is changing. Attractive public passenger transport services or flexible services such as car sharing are gaining importance. The BiE project (Evaluation of Integrated E-Mobility) focuses on better harmonizing such services and integrating them into daily life, determining where e-mobility fits in, and developing corresponding prototypes.
The results include an app that provides personal mobility assistance. Populated with data specifying individual preferences and mobility needs, it is able to make specific suggestions for personal daily mobility behavior. The assistant not only integrates e-mobility, but all mobility types, such as local and long-distance public transportation services, private motor transport, cycling, walking, and any combination of them. The user's upcoming appointments over the day are matched with the current road traffic and public transportation states so that any possible leeway can be used for travel planning. Such assistance could enable individual users to optimally coordinate their daily routine with their mobility needs, to receive updated suggestions for planning the necessary travel in case of unexpected events, and, as a side effect, to keep their families and appointment partners updated.
The question for the BiE project was how big the influence of such an assistance feature could be on the mobility needs of an entire region. The Institute for Transport Studies of KIT investigated this question in the Stuttgart region. Calculation models were used to determine the effects. The result was that this assistance feature can significantly reduce the average number and duration of trips as well as the distances to be covered. For half of the use cases, the assistance feature offered advantageous alternatives. The use of electric vehicles for private transport could provide an attractive mobility solution for regions such as the greater Stuttgart area.
For fleet operators, BiE concurrently developed a prediction model for the use of e-vehicles that factors in customer preferences, driving behavior, and vehicle consumption characteristics. Thanks to machine learning, the app incorporates a reliable prediction of the distance the user wants to cover, and the car in the fleet that is sufficiently charged and has an adequate distance to empty. Building on this data, the daily planning of the fleet bases can be optimized based on booking offers.
BiE is developing another consulting system that will help organizations such as municipalities, companies or neighborhood communities to deploy new vehicle fleets. Various input parameters such as organization size, number of persons, and mobility behavior allow the system to predict reliably whether and under which conditions the introduction of e-mobility makes sense. The output is a mobility architecture recommendation tailored to the individual needs, including statements on the dimension of investments, infrastructures, utilization, and economic efficiency of e-mobility solutions for almost any environment.
The overall objective of the BiE project is to design an evaluation system for the integration of e-mobility based on key indicators. Possible use scenarios will be simulated and validated prior to their implementation using novel concepts. The underlying networked mobility models were developed by the Institute of Product Engineering at KIT. With these models, individual recommendations adapted to each situation and based on a holistic system of key indicators became possible. Thus, the BiE partners can identify an appropriate infrastructure of charging stations for vehicle fleets, detect cooperation options of various service providers, or analyze traffic flows.
Provided by Karlsruhe Institute of Technology