AI researchers develop 'Darwin,' a neuromorphic chip based on spiking neural networks
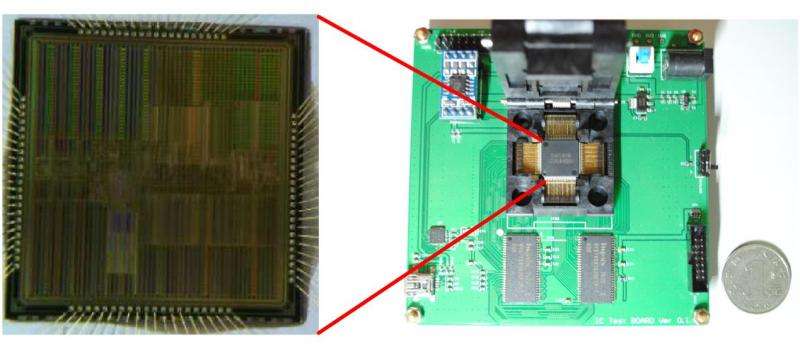
Artificial neural networks (ANNs) are a type of information processing system based on mimicking the principles of biological brains, and have been broadly applied in application domains such as pattern recognition, automatic control, signal processing, decision support systems and artificial intelligence. Spiking neural networks (SNNs) are a type of biologically inspired ANN that perform information processing based on discrete time spikes. They are more biologically realistic than classic ANNs, and can potentially achieve a much better performance-power ratio. Recently, researchers from Zhejiang University and Hangzhou Dianzi University in Hangzhou, China successfully developed the Darwin Neural Processing Unit (NPU), a neuromorphic hardware co-processor based on spiking neural networks, fabricated by standard CMOS technology.
With the rapid development of the "Internet of Things" and intelligent hardware systems, intelligent devices are pervasive in today's society, providing many services and conveniences to people's lives. But they also raise challenges of running complex intelligent algorithms on small devices. Sponsored by the college of Computer science of Zhejiang University, the research group led by Dr. De Ma from Hangzhou Dianzi university and Dr. Xiaolei Zhu from Zhejiang university has developed a co-processor called Darwin. The Darwin NPU aims to provide hardware acceleration of intelligent algorithms for resource-constrained, low-power embedded devices. It has been fabricated through a standard 180nm CMOS process, supporting a maximum of 2048 neurons, more than 4 million synapses and 15 different possible synaptic delays. It is highly configurable, supporting reconfiguration of SNN topology and many parameters of neurons and synapses. Figure 1 shows photos of the die and the prototype development board, which supports input/output in the form of neural spike trains via USB port.
The successful development of Darwin demonstrates the feasibility of real-time execution of spiking neural networks in resource-constrained embedded systems. It supports flexible configuration of a multitude of parameters of the neural network; it can therefore be used to implement different functionalities as configured by the user. Its potential applications include intelligent hardware systems, robotics, brain-computer interfaces, and others. Since it uses spikes for information processing and transmission, similar to biological neural networks, it may be suitable for analysis and processing of biological spiking neural signals, and building brain-computer interface systems by interfacing with animal or human brains. As a prototype application in brain-computer interfaces, Figure 2 describes an example application of recognizing the user's motor imagery intention via real-time decoding of EEG signals, i.e., whether the user is thinking of left or right, and using that information to control the movement direction of a basketball in the virtual environment. Different from conventional EEG signal analysis algorithms, the input and output to Darwin are both neural spikes: The input consists of spike trains that encode EEG signals; after processing by the neural network, the output neuron with the highest firing rate is chosen as the classification result.
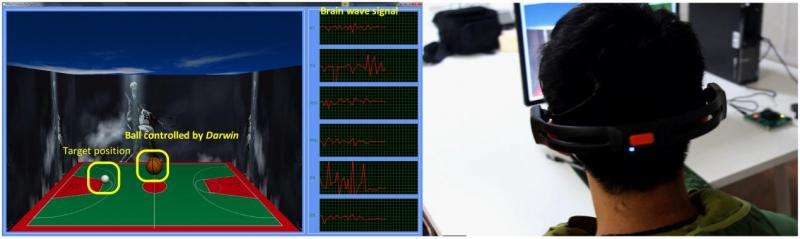
More information: "Darwin: a Neuromorphic Hardware Co-Processor based on Spiking Neural Networks",to be published in SCIENCE CHINA Information Sciences, 2016 No.2 issue: info.scichina.com/sciFe/EN/10.1360/112010-977
Provided by Science China Press