Study of Maglev train suspensions paves way for simpler industrial fault detection through AI
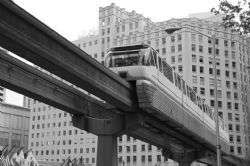
A new approach of industrial fault detection based on Artificial Intelligence has been studied on the electro-magnetic suspension of floating Maglev trains and could be applied to other safety-critical control systems, scientists believe.
Researchers analysed control system reliability using Maglev trains - a transport method that uses magnetic levitation to move vehicles without touching the ground, reducing friction and allowing higher speeds.
The results show that fault detection in industrial systems based on Artificial Intelligence methods could be less complex and of significantly lower computational cost than current technologies. It has strong potential to replace multiple estimators used in fault detection and isolation schemes in various industrial applications.
The research by Dr Argyrios Zolotas, from the School of Engineering, University of Lincoln, UK, together with Dr Konstantinos Michail, Dr Kyriakos Deliparaschos and Prof Spyridon Tzafestas from Cyprus University of Technology and National Technical University Athens, has been published in the academic journal IEEE Transactions on Control Systems Technology.
Typically, Fault Detection and Isolation (FDI) units for multiple sensor faults require a bank of estimators, such as Kalman Filters, that use the information from the remaining healthy sensors to construct the lost signal.
The results of this latest study show that a single artificial neural network-based estimator scheme achieves the same performance as the bank-based fault detector but with substantially lower computational cost. Artificial neural networks are computer-based algorithms used in machine learning which mimic the way neurons are structured in the human brain.
Dr Zolotas, Deputy Director of Research in the School of Engineering at the University of Lincoln, said: "Reliability is important in control systems and in particular to safety-critical systems. In modern system applications such as in Unmanned Aerial Vehicles, control methods that guarantee control reliability usually increase the computational cost of the already limited system resources.
"In this study, we analysed system design simplification for reliability in industrial applications, specifically in relation to sensor and actuator selection. The proposed fault detection scheme uses an Artificial Intelligence approach for developing a low computational fault detection unit that reduces the complexity and computational power of a bank-based equivalent."
The proposed framework was tested on an electro-magnetic suspension system used on Maglev trains to support the mass of the vehicle and passengers as well as to ensure proper ride quality during journeys.
A few simple modifications in the proposed architecture allowed the replacement of the bank of estimators with a typical Neural Network. The results show that this new approach has strong potential to replace multiple estimators used in FDI schemes in industrial applications.
More information: "AI-Based Actuator/Sensor Fault Detection With Low Computational Cost for Industrial Applications." IEEE Transactions on Control Systems Technology. DOI: 10.1109/TCST.2015.2422794
Provided by University of Lincoln