Computational chemistry: A faster way to untangle intermolecular interactions
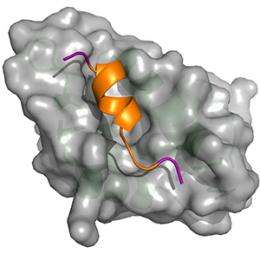
A powerful computational technique used by the pharmaceutical industry to expedite new drug development has just received a performance boost. Chandra Verma and his co-workers at the A*STAR Bioinformatics Institute in Singapore have developed a method for extracting greater information from the simulations that are used to predict how candidate drug molecules will interact with biomolecular targets. The technique could enable drug makers to create highly effective medicines for a broader range of individuals.
Pharmaceutical researchers currently process molecular dynamics simulations using a technique known as 'MM-PBSA' free-energy calculations. These calculations predict how tightly a drug candidate will bind to its target protein inside the body. In general, the more tightly a drug binds to its target, the more effective it will be. "MM-PBSA can be used to rapidly assess the relative binding propensities of a series of molecules to a protein to distil out a select few candidates that can then be tested experimentally," Verma explains. By cutting down on experimental work, companies can save time and money.
Since the MM-PBSA method was first developed in the late 1990s, computer processing power has increased significantly. Researchers can now run simulations that map drug–protein interactions over much longer timeframes. As simulation times have lengthened, the complexity of protein dynamics has become an increasingly important consideration. Proteins are inherently flexible structures with multiple possible conformations, each of which interacts differently with the drug molecule over time. Taking these differences into account provides more reliable results.
Verma and his co-workers' method for analyzing and reporting the results of MM-PBSA calculations, which they named MM-PBSA_segmentation, separately captures the free energies of binding for multiple protein conformations. MM-PBSA_segmentation is based on an algorithm that can extract the binding behavior of individual protein subpopulations from the overall free-energy calculations. For example, using their method on the well-characterized interaction between two proteins called p53 and MDM2, the researchers identified six distinct subpopulations of p53 conformations (see image). They also established each subpopulation's relative size and hence overall importance.
MM-PBSA_segmentation, which is freely available from the team, expands the range of protein conformations accessible for analysis, Verma says. This ability to examine multiple protein conformations could be particularly important for cancer drug development, for example, where protein targets can become mutated and so change their conformation. As these changes can differ among individuals, Verma and his team are currently investigating whether they can use their technique to develop drugs targeted to individual patients.
More information: Zhou, W., Motakis, E., Fuentes, G. & Verma, C. S. Macrostate identification from biomolecular simulations through time series analysis. Journal of Chemical Information and Modeling 52, 2319–2324 (2012). dx.doi.org/10.1021/ci300341v