This article has been reviewed according to Science X's editorial process and policies. Editors have highlighted the following attributes while ensuring the content's credibility:
fact-checked
trusted source
proofread
Innovative hybrid models enhance soil moisture forecasting
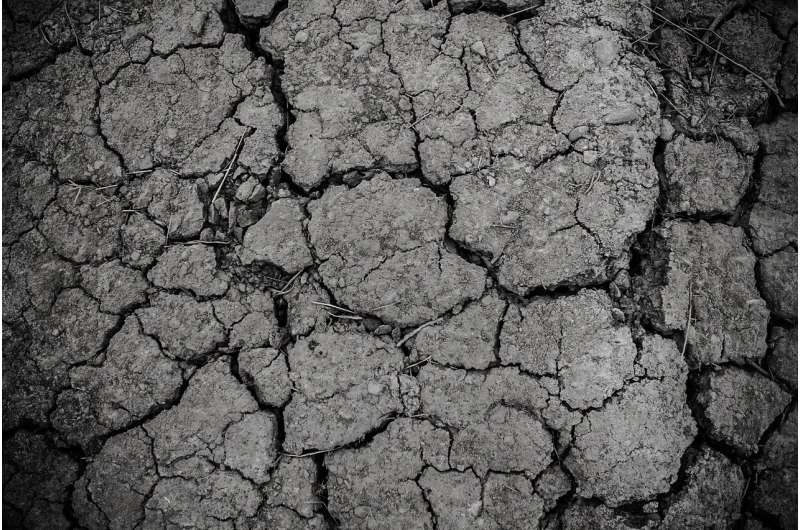
Soil moisture intricately influences climate and hydrological systems by modulating water and energy exchange processes. Accurate predictions of soil moisture are not only instrumental in drought monitoring but are crucial for effective water resource management.
Recognizing existing challenges in traditional physics-based models, characterized by uncertainties in parameters and limited representation of land-surface processes, Prof. Yongjiu Dai of Sun Yat-sen University and his team introduce attention-based hybrid models in their paper recently published in Advances in Atmospheric Sciences, fusing the strengths of physics-based models and deep learning capabilities.
Prof. Dai explained, "Our research seeks to overcome the limitations of pure deep learning models by leveraging the untapped potential of physics-based models at different forecast time scales. This innovative approach aims to adaptively extract important spatiotemporal patterns, improving the precision of soil moisture predictions in diverse regions."
Furthermore, the research addresses the shortcomings of previous hybrid models that predominantly relied on deep learning models to extract features from physics-based model outputs. "To the best of our knowledge, this is the first study taking both the attention mechanism and ensemble methods to integrate physics-based and deep learning models for soil moisture forecasting," said Prof. Dai.
Their study shows that the ensemble hybrid model outperforms current models for long-term predictions, and the attention hybrid model excels in drought predictions.
"While hybrid models have demonstrated promise in various hydrological forecasting areas, our research integrates physics-based and deep learning models explicitly for soil moisture predictions and balance pros and cons of different hybrid schemes," said Lu Li, a co-author of the study. "This innovative approach, combined with ensemble methods, aims to offer a unified model with enhanced predictability, reducing errors from individual models."
The research not only advances the understanding of hydrological processes but also provides a roadmap for the future of soil moisture predictions. Integrating physics-based and deep-learning models promises more accurate and regionally adaptive multi-step-ahead soil moisture forecasts.
More information: Lu Li et al, Enhancing Deep Learning Soil Moisture Forecasting Models by Integrating Physics-based Models, Advances in Atmospheric Sciences (2024). DOI: 10.1007/s00376-023-3181-8
Provided by Chinese Academy of Sciences