This article has been reviewed according to Science X's editorial process and policies. Editors have highlighted the following attributes while ensuring the content's credibility:
fact-checked
trusted source
proofread
New study is first step in predicting carbon emissions in agriculture
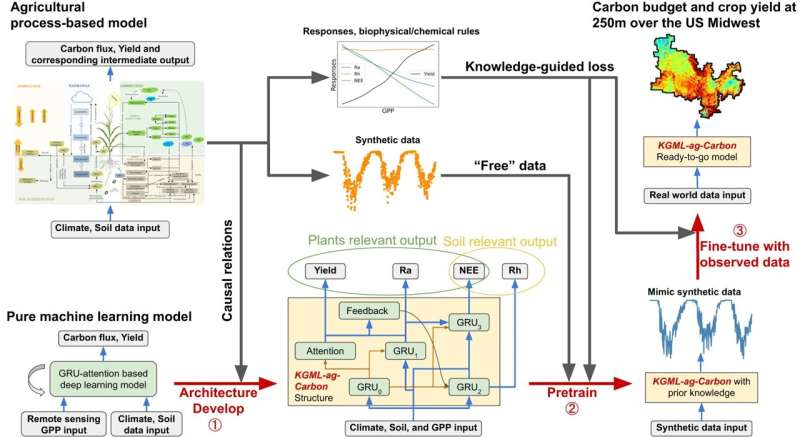
For the first time, researchers at the University of Minnesota Twin Cities (UMN) and the University of Illinois Urbana-Champaign (UIUC) have demonstrated that it is possible to provide accurate, high-resolution predictions of carbon cycles in agroecosystems, which could help mitigate the impacts of climate change.
The study by scholars from the UMN-led National Artificial Intelligence Institute for Climate-Land Interactions, Mitigation, Adaptation, Tradeoffs and Economy (AI-CLIMATE) and UIUC-led Agroecosystem Sustainability Center was recently published in Nature Communications.
The study's findings are a critical first step in developing a credible Measurement, Monitoring, Reporting, and Verification (MMRV) of agricultural emissions that can be used to incentivize the implementation of climate-smart practices while boosting rural economies.
This follows the national strategy, set by the White House, highlighting the need to quantify greenhouse gas emission across sectors with a goal of net-zero emissions by no later than 2050.
Accurate, scalable, and cost-effective monitoring and reporting of greenhouse gas emissions are needed to verify what are called "carbon credits" or permits that offset greenhouse gas emissions. Farmers can be reimbursed for practices that reduce greenhouse gas emissions. Agriculture accounts for about 25 percent of greenhouse gas emissions, but large corporations can be hesitant about purchasing these credits without knowing how much carbon is being stored.
Right now, to accurately gather carbon data, a farmer would need to hire someone to come to their farm, take what is called a soil core (vertical profile of the soil), and send that back to the lab for analysis.
"To gather the amount of data needed at each individual farm, it could cost the farmers time and money that they may not be willing to give," said Licheng Liu, the lead author and a research scientist at the University of Minnesota Department of Bioproducts and Biosystems Engineering.
The emerging field of Knowledge-Guided Machine Learning (KGML), pioneered by researchers at the University of Minnesota, combines the strength of artificial intelligence (AI) and process-based models from physical sciences.
With observations in the United States Corn Belt, the KGML-ag framework significantly surpasses both process-based and pure machine learning models in accuracy, especially with limited data. Remarkably, KGML-ag operates over 10,000 times faster than traditional process-based models, delivering high-resolution and high-frequency predictions cost-effectively.
"These knowledge-guided machine learning (KGML) techniques are fundamentally more powerful than standard machine learning approaches and traditional models used by the scientific community to address environmental problems," said Vipin Kumar, a University of Minnesota Regents Professor and William Norris Endowed Chair in the Department of Computer Science and Engineering.
Instead of taking soil cores at every farm, with KGML-ag, researchers can use the power of satellite remote sensing, computational models, and AI to provide an estimate of carbon in each individual field. This allows for compensation to individual farmers that is fair and accurate. The researchers say this is key to fostering trust in carbon markets and supporting the adoption of sustainable practices.
"KGML-ag combines the most advanced understanding of mechanisms in agriculture with the state-of-the-art AI techniques and thus offers a new powerful lens to monitor and manage our agricultural ecosystems," said Zhenong Jin, the corresponding author for this study and assistant professor in the University of Minnesota Department of Bioproducts and Biosystems Engineering, who co-leads the KGML special interest group in the AI-CLIMATE.
Now, AI-CLIMATE researchers are investigating the potential of the KGML framework for forestry, leveraging its capabilities to address the pressing challenges in sustainable forestry management and the capturing and storing of carbon. The team is also exploring a KGML-based data assimilation approach to make use of the rapidly growing different kinds of satellite data flexibly.
"The KGML is one of the key research topics of the AI-CLIMATE," said Shashi Shekhar, a University of Minnesota ADC Chair and Distinguished McKnight University Professor in the Department of Computer Science and Engineering and the lead researcher of the AI-CLIMATE Institute.
"These initial results demonstrate the immense potential of AI for developing more accurate and cheaper methods for estimating emissions from agriculture. This may lubricate carbon markets and incentivize the adoption of climate-smart practices."
More information: Licheng Liu et al, Knowledge-guided machine learning can improve carbon cycle quantification in agroecosystems, Nature Communications (2024). DOI: 10.1038/s41467-023-43860-5
Provided by University of Minnesota