This article has been reviewed according to Science X's editorial process and policies. Editors have highlighted the following attributes while ensuring the content's credibility:
fact-checked
peer-reviewed publication
trusted source
proofread
Machine learning could help find Martian caves for future human explorers
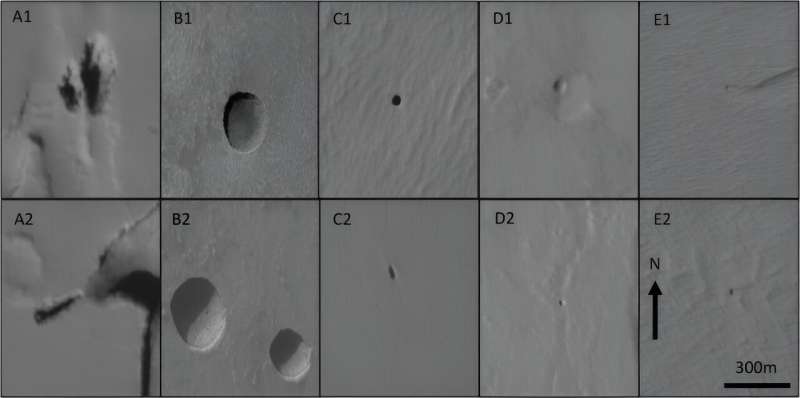
The surface of Mars is hostile and unforgiving. But put a few meters of regolith between you and the Martian sky, and the place becomes a little more habitable. Cave entrances from collapsed lava tubes could be some of the most interesting places to explore on Mars, since not only would they provide shelter for future human explorers, but they could also be a great place to find biosignatures of microbial life on Mars.
But cave entrances are difficult to spot, especially from orbit, as they blend in with the dusty background. A new machine learning algorithm has been developed to quickly scan images of the Martian surface, searching for potential cave entrances.
Researchers Thomas Watson and James Baldini from Durham University in the U.K. used a convolutional neural network (CNN), trained to identify potential cave entrances (PCEs) from images of the Martian surface to locate new potential caves. It was able to identify 61 new cave entrances from images in four different regions on Mars.
Previously, most detections of Martian PCEs have come from a manual review of visible satellite imagery, with images taken by the Mars Reconnaissance Orbiter's (MRO) Context Camera (CTX) and High-Resolution Imaging Science Experiment (HiRISE) cameras. A database from that manual review called the Mars Global Candidate Cave Catalogue (MGC3) contains the coordinates and brief descriptions of more than 1,000 identified PCEs on Mars.
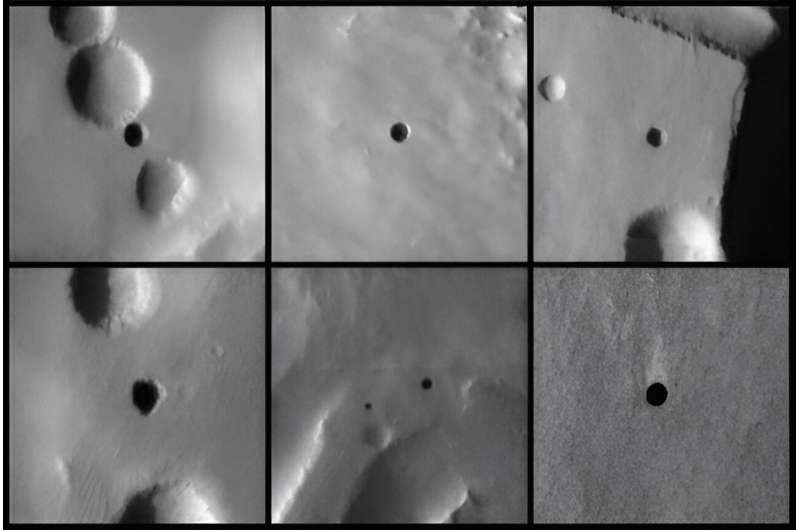
"Manual review of satellite imagery for Martian cave detection is far from efficient on a planet-wide scale," wrote Watson and Baldini in their paper, published in the journal Icarus, "due to the time constraints associated with reviewing such a large dataset. Machine learning presents an intriguing solution to this problem, reducing the dataset to only include imagery computationally determined to contain a PCE."
The caves on Mars are created by lava tubes, which were formed by flowing lava on ancient Mars. As the outside of the flowing lava cooled and solidified into a ceiling and walls, the interior stayed molten and kept flowing. Eventually, the lava flowed out of the tube in a downslope direction, leaving the tube intact and open.
Sometimes these lava tubes are apparent from linear pit chains on the surface—many of which are likely connected linearly underground. But more commonly, they are found by locating a "skylight" or collapsed lava tube ceiling in an orbital image. That skylight provides an entrance to the underground cave.
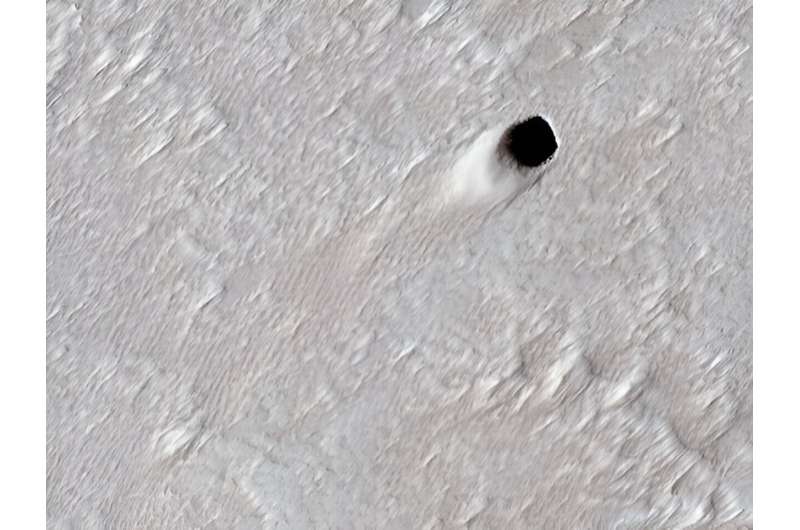
Lava tubes can be found on Earth, the moon, and Mars. Even though Earth is larger than Mars, some incredibly large lava tubes have been found on Mars, bigger than those found on Earth. On Earth, lava tubes are usually only as large as 14–15 meters (46–49 ft) wide—and typically much narrower. In 2020, the HiRISE (High-Resolution Imaging Science Experiment) camera on NASA's Mars Reconnaissance Orbiter (MRO) took a picture of a collapsed lava tube ceiling that is pit crater is 50 m (150 ft) across. The subsurface lava tube cave is likely larger than that.
Regular neural networks are commonly used for natural language processing and speech recognition. But CNNs or ConvNets are more often utilized for classification and computer vision tasks. CNNs can recognize patterns in images and provide image classification and object recognition to projects both large and small. For example, in a previous study, CNNs were trained to recognize Martian surface features, such as craters, and achieved accuracies of more than 90%.
Watson and Baldini created and trained their CNN model, called CaveFinder by having it look at images from the MGC3 catalog from the Tharsis and Elysium regions on Mars, which has the highest concentrations of volcanoes.
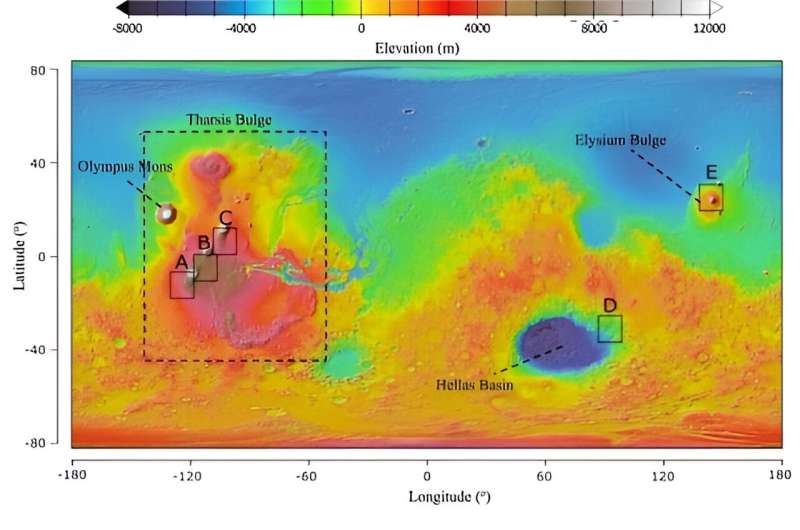
After the training period, CaveFinder achieved a test accuracy of 77%. It found four PCEs that Watson and Baldini highlighted for having specific qualities that make them interesting for further research, including one PCE nicknamed Marvin, which was the largest PCE identified, as well as another they called Emily, whose low altitude could enable surveyance by drone.
Additionally, CaveFinder identified twelve regions that appear to have multiple PCEs, which the authors say be a great place to explore multiple caves with a future mission, due to the proximity and abundance of PCEs.
But the researchers say that CaveFinder needs more work before being used on a large, planet-wide database. It had a large number of false positives, and it appears to have a limited ability to identify "lone small cave types, such as skylights and pinholes."
"CaveFinder is still not considered appropriate for detection on a planet-wide scale, due to the high number of false positive outputs requiring manual assessment," Watson and Baldini wrote. "However, it could prove effective in smaller regions perhaps already known to contain PCEs."
For future tests, they plan to increase the size of the training dataset used. Other ideas for improving CaveFinder's accuracy is to use thermal imagery alongside the visible data. Higher resolution images from a future Mars orbiter would also be beneficial for CaveFinder's increased detection and accuracy.
"Overall, this survey's findings indicate that, with these additions, machine learning has a great potential to advance remote cave detection, which is key to future Martian exploration," the researchers concluded.
More information: Thomas H. Watson et al, Martian cave detection via machine learning coupled with visible light imagery, Icarus (2024). DOI: 10.1016/j.icarus.2024.115952
Journal information: Icarus
Provided by Universe Today