This article has been reviewed according to Science X's editorial process and policies. Editors have highlighted the following attributes while ensuring the content's credibility:
fact-checked
proofread
AI-enabled forecasting model predicts nearly two years of ENSO events
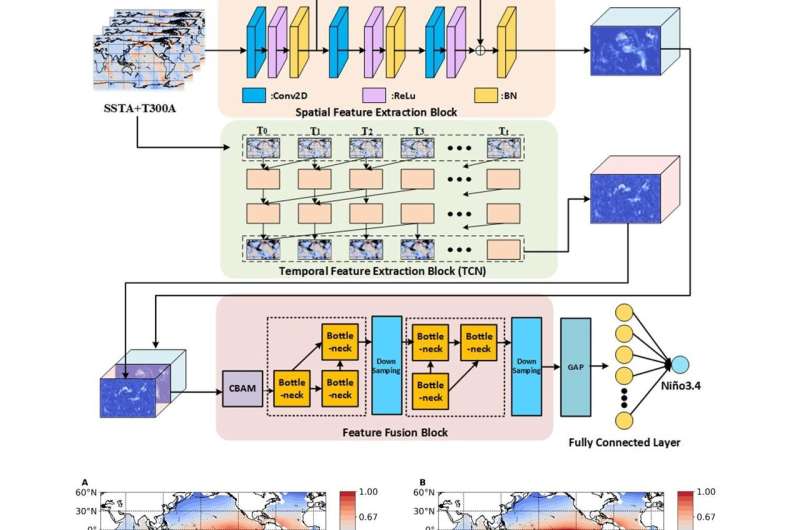
Every few years, changes in how the ocean and atmosphere interact along the West Coast—stretching from southern California to Peru and reaching across the Pacific almost to Fiji and the Solomon Islands—determine climate variability around the globe. To better predict this El Niño-Southern Oscillation (ENSO), an international research team applied artificial intelligence (AI) to develop a model capable of forecasting up to 22 months of this phenomenon.
They published their approach on May 17 in Ocean-Land-Atmosphere Research.
"ENSO dominates Earth's year-to-year climate variability and can often cause severe environmental and socioeconomic impacts globally," said first author Haoyu Wang, a doctoral student in the Chinese Academy of Sciences' Institute of Oceanology's Key Laboratory of Ocean Circulation and Waves. "However, despite continuous ENSO theory and modeling advances, the global heat signature variations preceding ENSO events have not been fully understood, especially for long-lead ENSO forecasts more than 12 months in advance."
This outer prediction of a year is partly limited by what the researchers call a spring persistence barrier, referring to the variability of the spring season as it transitions from the freeze of winter to the steam of summer. As temperatures shift at both the sea surface and in the atmosphere, the data becomes muddy and provides a more garbled indication of what might be expected from the ENSO.
"In this paper, we used AI methods to predict the ENSO phenomenon, achieving an effective prediction length of 22 months for Niño 3.4 and minimal influence from spring prediction barriers," said corresponding author Xiaofeng Li, professor in the Key Laboratory of Ocean Circulation and Waves. Niño 3.4 refers to the middle of the southern Pacific Ocean, halfway between the ENSO's outer borders. It is one of four Niño indices.
"Additionally, we designed an interpretable method to observe the relationship between global sea surface temperature and ocean heat content with ENSO from an AI perspective."
They named their approach the Spatio-Temporal Information Extraction and Fusion (STIEF) model and trained it on historic observations of surface sea temperatures and simulated ocean heat content data. According to Li, it contains two key components: the ability to extract space and time features and the ability to fuse those features together.
The deep learning model extracts the temporal and spatial ocean data characteristics in parallel. It then uses what it learns from those separate data points to understand how they correlate based only on the immediate past data points. This allows the model to avoid the pitfall of assuming a future data point is the result of a gradual lead up, compensating for the rapidly shifting changes of spring persistence barriers.
According to Wang, the team also designed the model to retroactively understand how it processed various data points to make predictions. Typically, the processing is too tangled to extract specific data and follow how the model used it in its forecast. Called the 'black box' issue, researchers can see the input variables and the output forecast, but the process remains a mystery.
"We designed an interpretable approach to solve the 'black box' issue in our AI-based ENSO prediction model," Wang said. "This allows us to observe the correlations between different variables from the perspective of AI, providing new insights for our ocean forecast phenomena theoretical research."
The researchers said they plan to continue improving their model and eventually apply it to all four Niño indices to explore ENSO diversity. The eventual goal is to establish an interpretable AI model that is applicable to various ocean phenomena forecasts.
More information: Haoyu Wang et al, An Interpretable Deep Learning Model for ENSO Forecast, Ocean-Land-Atmosphere Research (2023). DOI: 10.34133/olar.0012
Provided by Ocean-Land-Atmosphere Research (OLAR)