March 14, 2023 feature
This article has been reviewed according to Science X's editorial process and policies. Editors have highlighted the following attributes while ensuring the content's credibility:
fact-checked
peer-reviewed publication
trusted source
proofread
Quantum chemistry simulations on a quantum computer

In a new report now featured on the cover page of and published in Science Advances, Hans Hon Sang Chan and a research team in materials, chemistry and quantum photonics at the University of Oxford generated exactly emulated quantum computers with up to 36 qubits to explore resource-frugal algorithms and model two- and three-dimensional atoms with single and paired particles.
Chemistry modeling is a natural attribute for quantum computers, although existing methods are impractical to develop near-perfect qubits. In this work, quantum chemists explored a range of tasks from ground state preparation and energy estimation to scattering and ionization dynamics of electrons, to assess a variety of methods in the split-operative simulation in order to emulate the quantum chemistry of a few molecules of interest. The grid-based method performed exceptionally to make way for a less error-prone quantum computing era.
Quantum computing via a real-space grid approach
Quantum chemists envision quantum computers to be transformative tools for chemistry prediction and exploration. While conventional computers are useful to explore quantum molecular dynamics to predict reaction outcomes and experimental observables, the hardware costs and time duration can scale exponentially with the number of simulated particles. In this work, Chan and colleagues studied the underlying characteristics of accelerating chemical dynamics simulations on early versions of quantum computers based on a real-space grid approach.
These early versions of quantum computers had a limited number of error-corrected qubits. The team encoded features such as particle symmetry to offer optimal resource scaling for complex and interesting molecules during the study.
Most quantum computers are noise-burdened and costly. The researchers therefore took a different approach by deploying classical computing resources to emulate small, but noise-free quantum computers to thereby simulate quantum molecular dynamics within them—to directly examine the costs and performance measures. While they did not rehash pre-existing classical-grid techniques to perform grid-based simulations, they conducted emulations of real, noise-free quantum machines for chemically relevant quantum dynamics instead.
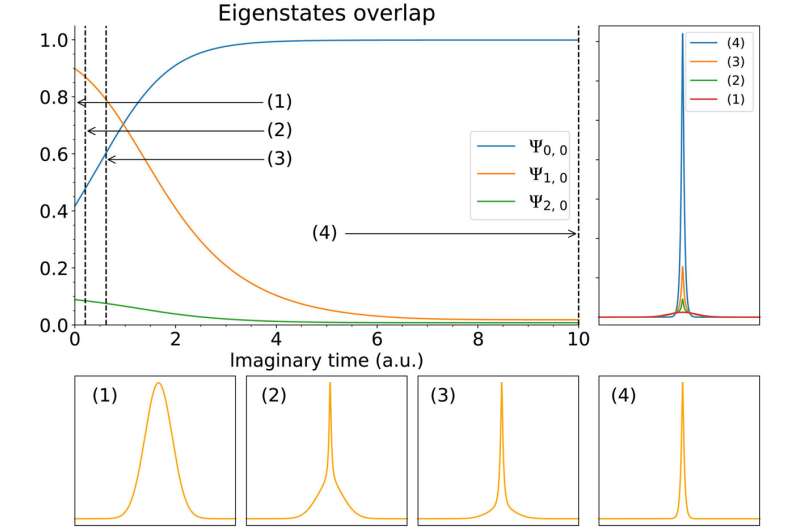
A new approach for quantum chemistry
The costs of mimicry limited the quantum computer-emulations to modest-sized versions containing 36 perfect qubits. The team used the experimental setup to explore several informative scenarios for 2D and 3D simulations of one- and two-electron systems. They selected two key areas of interest in chemistry, and estimated the requisite quantum resources to simulate the dynamics of strong external fields, followed by simulations of particle scattering dynamics.
During the first experiment, the team applied an external field suddenly with resulting dipole oscillation and ionization of a single bound electron. They envision efforts in this direction to encompass topics such as photochemistry and laser excitation. Physicists and quantum chemists consider coherent quantum regulation of small molecules to be one of the "holy grails" of chemical science. For example, the process can allow scientists to study ammonia in the context of hydrogen atom removal to explore its potential in modern agriculture.
In the second scenario, the team examined electron-molecule scattering relevant in spectroscopy, astrochemistry and manufacturing processes, since the processes of collision and scattering are highly dynamic and difficult for classical modeling.
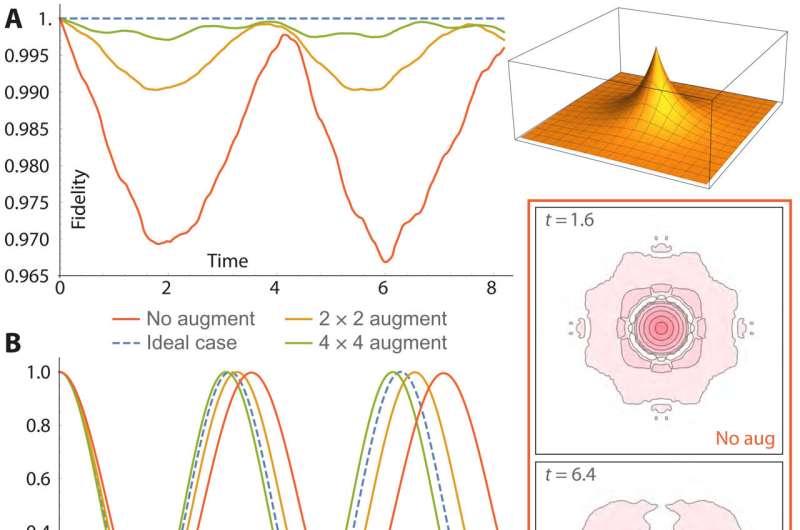
Wave simulation
The scientists used the split-operator quantum Fourier transform (SO-QFT) Hamiltonian simulation approach to perform wave packet operations and presented a range of results that applied to the grid-based method for 2-to-3D systems using single and paired particles. Based on the outcomes, they assessed the quantum resources required for simulations, in order to present suitable quantum hardware architecture.
The numerical outcomes led to emulating quantum processors by implementing them via open-source tools such as QuEST, QuEST-link and pyQuEST. They explored the number of qubits and estimated the duration of execution to achieve simulations of given accuracy, and studied a scenario of a sampling-based method to estimate the system's energy, which proved to be highly sensitive to imperfections. They estimated the accompanying quantum resource costs and indicated the hardware layout for a suitable quantum computer.
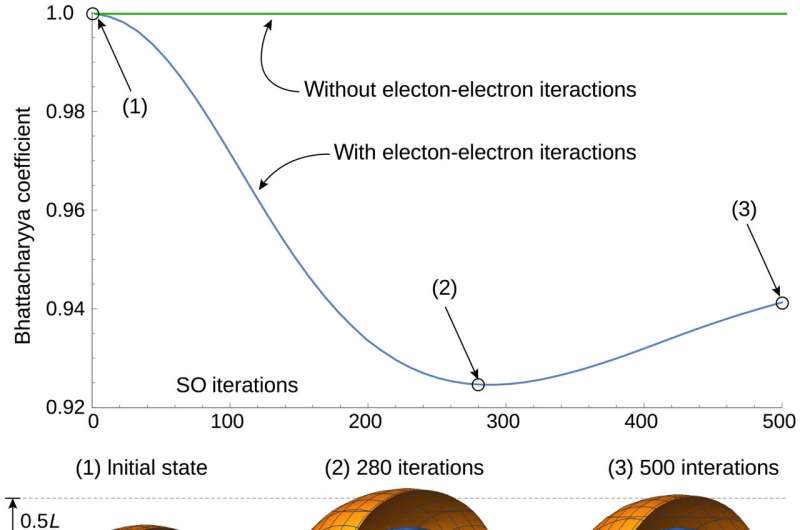
Methods and 3D simulations
The research team explored single-ancilla iterative phase estimation (IPE) measurements to project out excited states. The IPE circuits are interesting and important for near-term quantum computing in practice. The results served for energy estimation and the team conducted alternative approaches to prepare real-space ground states on a quantum computer based on probabilistic imaginary time evolution (PITE) and simulated the ground state of 2D hydrogen, while detailing drawbacks of the method. They carried out two scenarios of quantum dynamics simulations that relied on two scenarios 1) ionization by a strong external field, and 2) dependence on electron-electron scattering.
The team next introduced the augmented split-operator (ASO) to optimize the fidelity of the simulation by presenting additional elements to the basic SO-QFT (split-operator quantum Fourier transform) cycle. When operating with strict Coulomb interactions common to all numerical studies as seen in this paper, the ASO method was highly relevant. Using the setup, they simulated the dynamics of a helium atom in 3D. They used the Schrodinger equation to approximate the true electron eigenstates of the helium atom, and the Bhattacharya coefficient to represent electron-electron interactions and time evolution of the helium atom simulation.
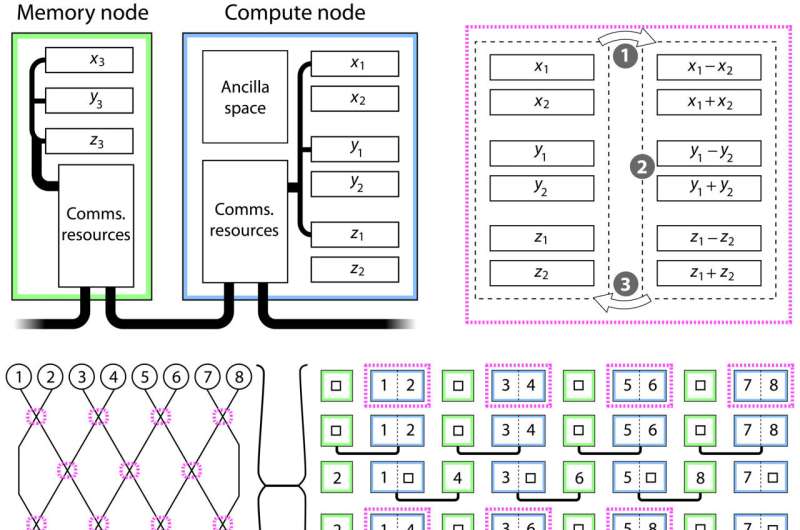
Quantum computing resources and architectures
The quantum chemists studied the resource requirements to undertake quantum modeling beyond the reach of classical algorithms, and aligned quantum architectures suited for such expressions. They estimated the number of qubits necessary to model quantum scenarios of interest for hexafluoro ethane (C2F6) and ammonia (NH3) molecules. The grid-based simulations of C2F6 required about 2250 computational qubits, while the ammonia molecules required less than 450 qubits.
The time cost for simulation also relied on hardware realization. As a result, the most well-understood codes required many hundreds of physical qubits per logical qubit relative to deep algorithms and error-rates, comparable to the best quantum computer prototypes of today. The researchers additionally devised a multicore network architecture to support the theoretical massive-scale qubits.
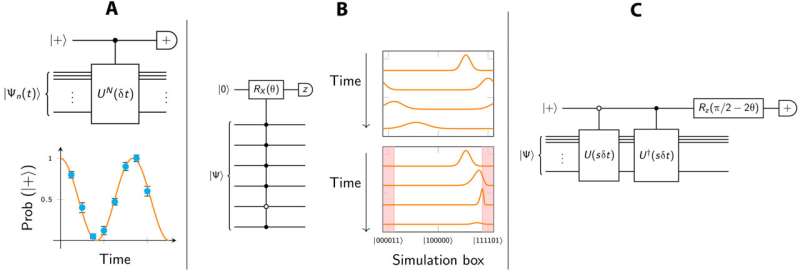
Outlook
In this way, Hans Hon Sang Chan and colleagues explored the split-operator quantum Fourier transform (SO-QFT) approach to emulate exact qubits and test the technologies behind real-space quantum chemistry simulations. They explored several known quantum techniques and introduced a few others to convey key aspects of quantum simulation. The scientists characterized the resources underlying the realization of digital experiments on early fault-tolerant quantum computers.
The outcomes can be a learning/prediction cycle by augmenting physical experiments with machine learning to accelerate chemical discovery. The results can lead to diverse fields of quantum technology, including special relativity to model high energy particles as well as play a role in financial engineering.
More information: Hans Hon Sang Chan et al, Grid-based methods for chemistry simulations on a quantum computer, Science Advances (2023). DOI: 10.1126/sciadv.abo7484
Chris Sparrow et al, Simulating the vibrational quantum dynamics of molecules using photonics, Nature (2018). DOI: 10.1038/s41586-018-0152-9
Journal information: Science Advances , Nature
© 2023 Science X Network