Machine learning could revolutionize mineral exploration
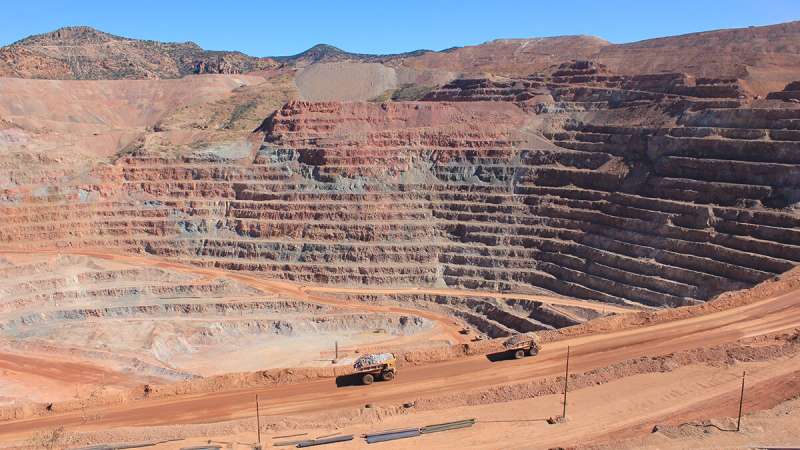
Twenty-first century technologies, including those central to a low-carbon future, rely on rare earth elements and metals. Many of these sought-after minerals reside in porphyry copper deposits that contain hundreds of millions of metric tons of ore. In addition to copper, these deposits are a source of significant quantities of gold, molybdenum, and rhenium. However, the mining industry has identified and mined most of the world's large and accessible porphyry deposits. Despite growing investment in mineral exploration, the rate of discovery for mineral deposits is decreasing.
In a study recently published in the Journal of Geophysical Research: Solid Earth, Zou et al. present two novel machine learning techniques to identify new, deeply buried porphyry copper deposits by characterizing magma fertility. Fertile magma refers to magmas that can form porphyry deposits. Barren magmas, in contrast, are not likely to develop rich ores. The authors aimed to improve traditional geochemical indicators plagued by high false-positive rates.
The authors developed two algorithms, random forest and deep neural network. They formulated the models using a global data set of zircon chemistry, which is used to evaluate the porphyry copper deposits in magma. The authors focused the models on 15 trace elements. They validated the models with independent data sets from two well-characterized porphyry copper deposits in south central British Columbia, Canada, and Tibet, China.
Both models resulted in a classification accuracy of 90% or greater. The random forest model exhibited a false-positive rate of 10%, whereas the deep neural network model had a 15% false-positive rate. In comparison, traditional metrics report false positives at a 23%–66% rate.
Europium, yttrium, neodymium, cerium, and other elements emerged as significant indicators of magma fertility. The models' performances indicate that the algorithms can distinguish between fertile and barren magmas using trace element ratios. Notably, model performance was not affected by regional differences or the geologic setting between the evaluation data sets from Canada and China.
As the demand for rare earth elements, minerals, and metals surges, new techniques are required to discover previously unknown deposits. According to the researchers, the results highlight machine learning's promise as a robust, accurate, and effective approach for identifying and locating porphyry copper resources.
More information: Shaohao Zou et al, Application of Machine Learning to Characterizing Magma Fertility in Porphyry Cu Deposits, Journal of Geophysical Research: Solid Earth (2022). DOI: 10.1029/2022JB024584
Journal information: Journal of Geophysical Research: Solid Earth
Provided by American Geophysical Union
This story is republished courtesy of Eos, hosted by the American Geophysical Union. Read the original story here.