June 23, 2022 report
Using machine learning to narrow down the possibilities for a better quantum tunneling interface
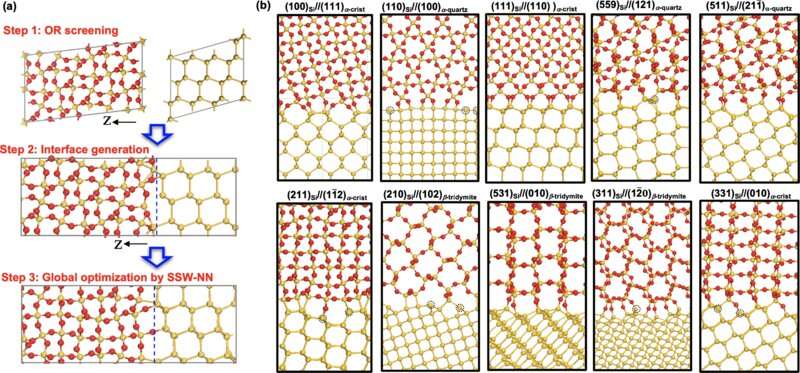
A pair of researchers at Fudan University in China has used machine learning to narrow the list of possible improved tunneling interface configurations for use in transistors. They have published their results in Physical Review Letters.
Over the past several decades, engineers have worked to uphold Moore's law, faithfully doubling the number of transistors that could be placed on an integrated circuit roughly every two years. But such efforts are in jeopardy due to the laws of physics—most particularly, those related to quantum tunneling that degrade performance. More specifically, the material that is used to separate gates on chips (interfaces) from channels has become so thin that charge carriers can wiggle their way through via quantum tunneling. In this new effort, the researchers sought stable configurations that would minimize such tunneling, thereby allowing Moore's law to continue, at least for a while.
The work involved studying how tunneling is impacted by the structure of a given interface. The researchers discovered that the configuration of the material that made up the interface played a major role in the degree of quantum tunneling. They then used a machine learning application to study approximately 2,500 structures as possible candidate interface configuration replacements. They found 40 configurations that appeared likely to provide a better option than those that are currently in use. Of those, they found that just 10 were energetically stable. Testing of the 10 candidates showed that just two were able to suppress tunneling. They suggest the two configurations could be used in integrated circuit design and production to allow for more transistors on a chip, which in practice allows for the creation of smaller devices.
The researchers next plan to refocus their efforts to see if other transistor materials might be more suitable for use in the next generation of integrated circuits.
More information: Ye-Fei Li et al, Smallest Stable Si/SiO2 Interface that Suppresses Quantum Tunneling from Machine-Learning-Based Global Search, Physical Review Letters (2022). DOI: 10.1103/PhysRevLett.128.226102
Journal information: Physical Review Letters
© 2022 Science X Network