Comparing machine learning models for earthquake detection
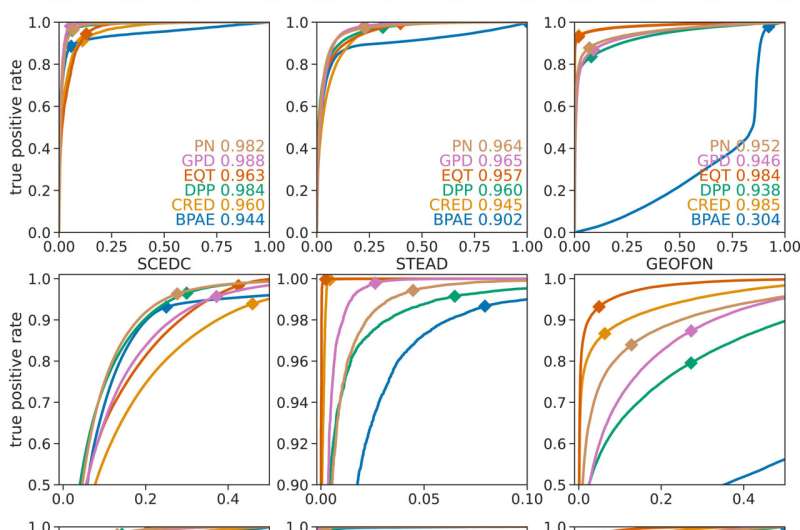
Machine learning is transforming data-heavy fields across the sciences, and seismology is no exception. Several machine learning methods have emerged for earthquake detection, phase identification, and phase picking. However, choosing which method to use is still a challenge because it's not always clear how these deep learning models will respond to data that differ from the data sets they were trained on.
To provide some insight, Münchmeyer et al. compared six deep learning models (and one classical picking model) to find out which perform best across various data sets. The team looked at BasicPhaseAE, CNN-RNN Earthquake Detector (CRED), DeepPhasePick (DPP), Earthquake Transformer (EQTransformer), Generalized Phase Detection (GPD), and PhaseNet. They evaluated each model's performance on three common tasks: event detection, phase identification, and onset time picking.
The researchers found that when the models were trained and evaluated using data sets with identical characteristics, EQTransformer performed best on all three tasks, followed closely by PhaseNet and GPD. For event detection, CRED showed excellent performance as well.
However, the authors note that in the real world, researchers often have data sets with different characteristics than a model's training data. Therefore, the team also evaluated model performance with a cross-domain setup, testing each model with data sets it had not been trained on. The models still worked relatively well, the researchers found, provided that the distance, either regional or teleseismic, was similar for the training and testing data sets.
The team built this benchmark on the SeisBench platform to allow for the addition of new data sets or machine learning methods in the future. Eventually, these or other new deep learning models could be useful for early detection and warning systems for earthquakes. However, further research is needed to evaluate performance for real-time identification of earthquake arrivals before those applications can be realized, according to the authors.
More information: Jannes Münchmeyer et al, Which Picker Fits My Data? A Quantitative Evaluation of Deep Learning Based Seismic Pickers, Journal of Geophysical Research: Solid Earth (2022). DOI: 10.1029/2021JB023499
Journal information: Journal of Geophysical Research: Solid Earth
Provided by American Geophysical Union
This story is republished courtesy of AGU Blogs (http://blogs.agu.org), a community of Earth and space science blogs, hosted by the American Geophysical Union. Read the original story here.