Computer-aided systems may speed up new anticancer drug development
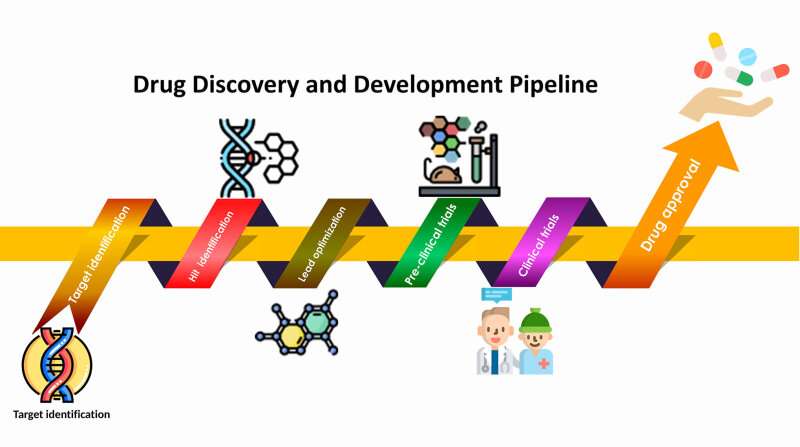
New drug discovery is a complicated, expensive and time-consuming process. Traditional drug development pipeline needs 12 years and $2.7 billion USD on average.
Reducing research costs and speeding up development processes of new drug discovery are challenging issues for the pharmaceutical industry.
Recently, the rapid growth of computational tools, such as computer-aided drug discovery (CADD), has made significant impact on anticancer drug design. CADD enables faster, cheaper and more effective drug design, and provides fruitful insights in the area of cancer therapy.
Researchers from the Shenzhen Institutes of Advanced Technology (SIAT) of the Chinese Academy of Sciences analyzed different subareas of the CADD process with a focus on anticancer drugs. Their study was published in Frontiers in Pharmacology.
The study indicated that computational drug design has promoted the discovery of several new anticancer drugs, which has become a milestone in this area. Gefitinib, Erlotinib, Sorafenib, Lapatinib, Abiraterone and Crizotinib were all approved drugs discovered based on computational drug methods.
With the arrival of artificial intelligence (AI), the design of anticancer drugs in silico has undergone unprecedented changes. State-of-the-art deep learning approaches, such as retro-synthetic routine planning, drug scaffold generation and drug binding affinity predictions have the potential to produce excellent chemical properties needed for new molecules.
"We believe that useful predictions generated by computational models combined with experimental validations could further speed up anticancer drug development," said Dr. Cui Wenqiang, first author of the study.
More information: Wenqiang Cui et al. Discovering Anti-Cancer Drugs via Computational Methods, Frontiers in Pharmacology (2020). DOI: 10.3389/fphar.2020.00733
Provided by Chinese Academy of Sciences