Researchers study interactions in molecules using AI
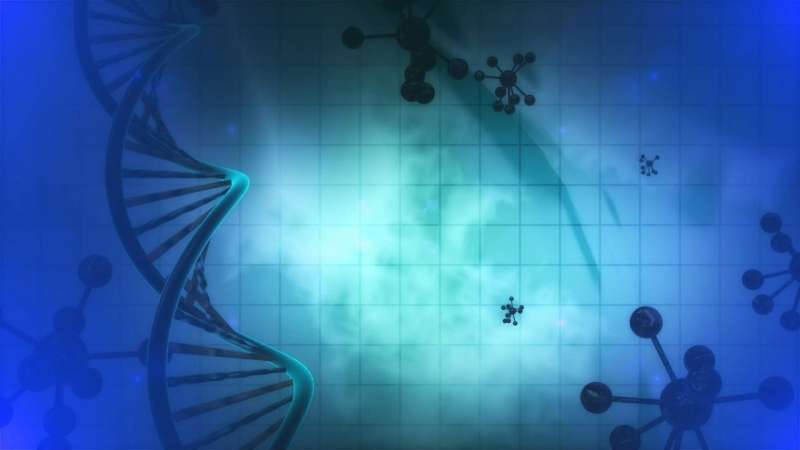
Researchers from the University of Luxembourg, Technische Universität Berlin, and the Fritz Haber Institute of the Max Planck Society have combined machine learning and quantum mechanics to predict the dynamics and atomic interactions in molecules. The new approach allows for a degree of precision and efficiency that has never been achieved before.
Molecular dynamics simulations are used in natural and material science to predict the properties and behavior of different materials. In the past, these simulations were usually based on mechanistic models that are unable to integrate important insights from the quantum mechanics. This work now published in Nature Communications substantially improves the prediction capabilities of modern atomistic modeling in chemistry, biology, and the material sciences.
Exact knowledge about the molecular dynamics of a substance, in other words precise knowledge of the possible states and interactions of single atoms in a molecule, enables us to not only understand many chemical and physical reactions but also to make use of these. "Machine learning techniques have dramatically altered work in many disciplines, but up until now, little use has been made of them in molecular dynamics simulations," says Klaus-Robert Müller, Professor of machine learning at TU Berlin. The problem: Most standard algorithms have been developed with the understanding that the amount of data to be processed is of no relevance. "This doesn't apply, however, for accurate quantum mechanical calculations of a molecule, where every single data point is crucial and the individual calculation for larger molecules can take several weeks or even months. The enormous computational resources required to do this has meant that precise molecular dynamics simulations have not been possible to date," explains Alexandre Tkatchenko, professor of theoretical chemical physics at the University of Luxembourg.
It is precisely this problem which the researchers have now solved by integrating physical laws into machine learning techniques. "The trick consists in not calculating all potential possible states of molecular dynamics with machine learning techniques, but rather only those which do not result from known physical laws or the application of symmetry operations", explains Professor Alexandre Tkatchenko.
On the one hand, the newly developed algorithms use natural mathematical symmetries within molecules. Among the things they recognise are axes of symmetry which do not alter the physical characteristics of the molecule. As a result, these data points need only be calculated once, rather than several times, which greatly reduces the complexity of the calculation. Additionally, the learning techniques use the physical law of the conservation of energy.
Through this innovative approach of allowing the machine learning techniques employed to "incorporate" physical laws before learning to calculate the molecular dynamics, the research team has succeeded in reconciling the two contradicting aspects of high precision and data efficiency. "These special algorithms permit the process to focus on the complex problems of the simulation, rather than using computer performance for the reconstruction of trivial relationships between data points. As such, this research demonstrates the great potential of combining AI and chemistry or other natural sciences", Klaus-Robert Müller says, explaining the significance of the project.
More information: Stefan Chmiela et al. Towards exact molecular dynamics simulations with machine-learned force fields, Nature Communications (2018). DOI: 10.1038/s41467-018-06169-2
Journal information: Nature Communications
Provided by University of Luxembourg