Deep learning for glaucoma detection
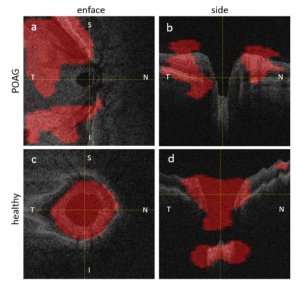
Glaucoma is the second leading cause of blindness in the world, impacting approximately 2.7 million people in the U.S alone. It is a complex set of diseases and, if left untreated, can lead to blindness. It's a particularly large issue in Australia, where only 50 percent of all people who have it are actually diagnosed and receive the treatment they need.
As part of a team of scientists from IBM and New York University, my colleagues and I are looking at new ways AI could be used to help ophthalmologists and optometrists further utilize eye images, and potentially help to speed the process for detecting glaucoma in images. In a recent paper, we detail a new deep learning framework that detects glaucoma directly from raw optical coherence tomographic (OCT) imaging, a method which uses light waves to take cross-section pictures of the retina. This method achieved an accuracy rate of 94 percent, without any additional segmentation or scrubbing of the data, which is usually time-consuming.
Currently, glaucoma is diagnosed using a variety of tests, such as intraocular pressure measurements and visual field tests, as well as fundus and OCT imaging. OCT provides an efficient way to visualize and quantify structures in the eye, namely the retinal nerve fibre layer (RNFL), which changes with progression of the disease.
Although this approach works well, it requires an additional process to quantify the RNFL in OCT images. These techniques also typically clean up the input data in a variety of ways, such as flipping all eyes into the same orientation (left or right) in order to reduce variability in the data to improve the performance of the classifiers. Our approach removes these additional steps, indicating that these potentially time-consuming stages are not required for the detection of glaucoma.
Ultimately, when normalised by a false positive rate, in a cohort of 624 subjects (217 healthy and 432 glaucoma patients), our new approach, founded in deep learning, correctly detects glaucomatous eyes in 94 percent of cases, while previously mentioned techniques only found this in 86 percent of cases. We believe this improved accuracy is a result of eliminating errors in the automated segmentation of structures in images as well as the inclusion of regions of the image that are not currently utilised clinically for this purpose.
Additionally, contrary to the current trend in AI research that uses larger and deeper networks, the network we used was a small 5-layer network because medical data is not as easily accessible due its confidential nature. This data scarcity makes the use of large networks impractical in many medical applications. Even in research, we are sometimes seeing that "less is more," and the training of these algorithms on smaller networks allows them to run with greater efficiency.
This is just one facet of our research in applying AI for the eye. In a recently announced new collaboration, IBM Research and George & Matilda (G&M) will leverage G&M's robust data set of anonymous clinical data and imaging studies to explore methods to use deep learning models and imaging analytics to support clinicians in the identification and detection of eye disease—including glaucoma—in images. Researchers will also look to investigate the potential biomarkers of glaucoma, which could help in better understanding disease progression.
More information: Stefan Maetschke et al. A feature agnostic approach for glaucoma detection in OCT volumes. arXiv:1807.04855 [cs.CV]. arxiv.org/abs/1807.04855v1
Provided by IBM
This story is republished courtesy of IBM Research. Read the original story here.