Network traffic anomaly detection
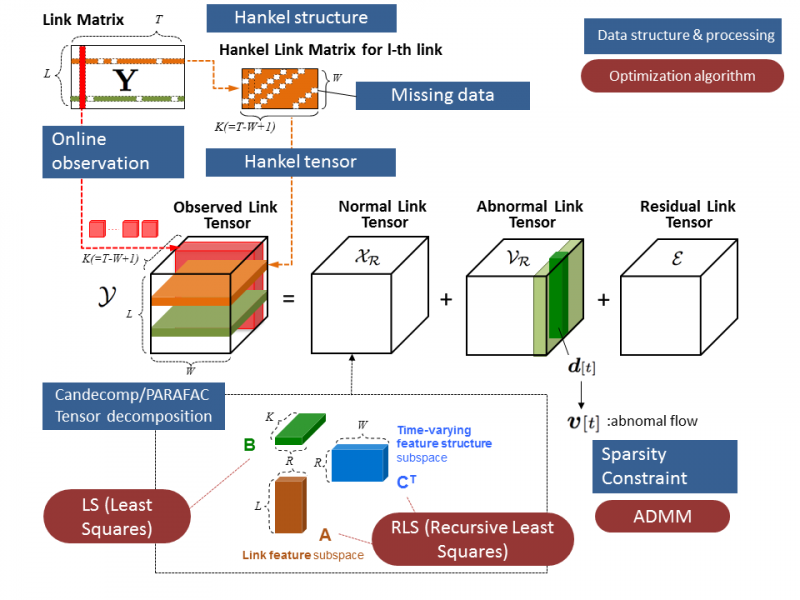
"Diagnosing unusual events (called "anomalies") in a large-scale network like Internet Service Providers and enterprise networks is critical and challenging for both network operators and end users," explain Hiroyuki Kasai from The University of Electro-Communications in Japan, and co-authors Wolfgang Kellerer Martin Kleinsteuber at the Technical University of Munich in Germany in a recent report. In their latest work they devise a computationally efficient and effective algorithm to identify network level anomalies by exploiting the state-of-the-art machine learning algorithms, especially the large-scale higher-order tensor tracking technique.
Kasai, Kellerer and Kleinsteuber describe their system as data flows from origin to destination along courses that cross at various links. Measuring the traffic volume of each flow is incredibly data intensive, so instead the researchers focus on the directly observable but coarse link matrix, for which they then need to identify how they can estimate the unobservable flow matrix for the full network from the link matrix.
The researchers also avoid storage issues for large sets of archive data by developing the algorithm to operate online. They formulate their system with a latent structure of normal flows with noise, and they can then estimate abnormal flows as outlier sparse flows by leveraging sparse modelling.
As the researchers point out in their report, network anomalies can be caused by deliberate malicious operations, or misconfigurations and failures of network equipment, all of which are important to identify. They add, "Extensive numerical evaluations show that the proposed algorithm achieves faster convergence per iteration of model approximation, and better volume anomaly detection performance compared to state-of-the-art algorithms."
More information: Hiroyuki Kasai et al. Network Volume Anomaly Detection and Identification in Large-Scale Networks Based on Online Time-Structured Traffic Tensor Tracking, IEEE Transactions on Network and Service Management (2016). DOI: 10.1109/TNSM.2016.2598788
Provided by University of Electro-Communications