Machine learning techniques enable models from partial image data
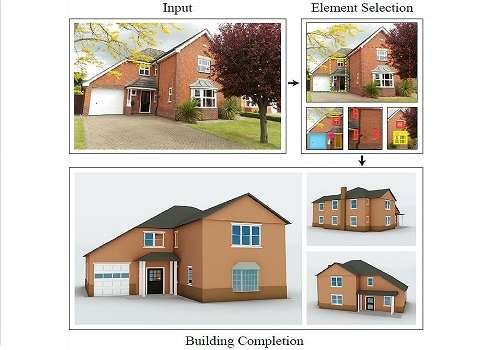
Virtual three-dimensional models of buildings and cities are used in an increasing number of situations, including systems such as driving and flying simulators and first responder training, internet maps, games, movies and even architectural and engineering walkthroughs. In each of these virtual environments, small buildings or dwellings can make up a huge proportion of the objects encountered and the character of these buildings sets the look, identity and locale for the simulation. However, with thousands of such buildings in the background, developers of virtual cities can have difficulty populating their world models with realistic buildings in addition to the specific locations that form the focus of the simulation.
By integrating machine learning techniques, Lubin Fan and Peter Wonka from King Abdullah University of Science and Technology's Visual Computing Center have developed a method to automatically complete and generate three-dimensional building models characteristic of a given area by using partial images.
"Machine learning allows us to take data for existing buildings and learn the 'look' of those buildings, which then allows us to synthesize new buildings," said Wonka. "This technique is used widely in visual computing for objects such as humans and airplanes, but buildings and their structural variations are more difficult to learn. The core of our work was to come up with a meaningful set of features, parameters and their relationships that can describe buildings generically."
Thanks to initiatives such as Google Streetview, enormous volumes of data on residential buildings are available. Wonka and Fan's modelling scheme takes such datasets and extracts key external features of each building, such as the observable footprint, size of the garage, roof style and the window-to-wall ratio.
"The scheme then 'learns' a probabilistic graphical model to encode the relationships between these features," noted Wonka. "A user can then sample specific features or fix observed features and compute the unobserved structure. Finally, there is an optimization step that translates building features into 3-D building models."
Once trained, the model can generate a large variety of buildings, and by setting hard constraints, plausible complete models can be created even from partial observations. This allows designers and developers to automatically and simply generate many buildings with the character of the particular area or style.
"Our probabilistic model for exteriors of residential buildings could also help architects more easily generate building prototypes or generate plausible 3-D reconstructions from a limited set of photographs," Wonka said.
More information: A probabilistic model for exterior of residential buildings. ACM Transactions on Graphics, DOI: 10.1145/2910578