A counterintuitive approach yields big benefits for high-dimensional, small-sized dataset problems
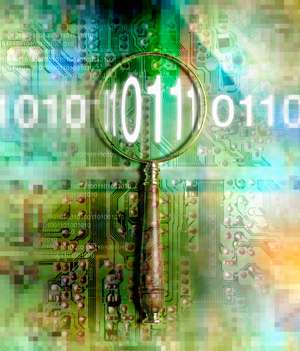
Extracting meaningful information out of clinical datasets can mean the difference between a successful diagnosis and a protracted illness. However datasets can vary widely both in terms of the number of 'features' measured and the number of independent observations taken. Now, A*STAR researchers have developed an approach for targeted feature selection from datasets with small sample sizes, which tackles the so-called class imbalance problem.
The class imbalance problem—when the common, or 'majority class' data, overwhelm the rare, or 'minority class' data—is a significant hurdle in data mining. This is particularly evident for datasets that have lots of features, known as high-dimensional data, or have few samples—both of which are common to gene expression analysis and clinical data.
Feng Yang and colleagues from the A*STAR Institute of High Performance Computing took an unconventional approach to this problem. They began with a common pattern classification method called linear discriminant analysis (LDA). But to make feature selection tractable, the dataset had to be 'regularized'.
"After we analyzed the different forms of regularization," Yang recalls, "we found that one intrinsic difference of the existing forms of regularization is the class emphasis."
Existing regularization methods favored the majority class: "intuitively, the majority class should be given more emphasis weight since it has more samples," acknowledges Yang, "however, our study proved that this is not true in the high-dimensional, small-sized situation with class imbalance."
Indeed, their study showed that when the minority class was more heavily emphasized, that both the classification accuracy and the robustness performance improved.
"From the view of sample distribution in the subspace, minority class emphasis will actually 'squeeze' the samples in the minority class to form a compact 'nucleus' in the subspace of selected features, which would be easier to be classified," Yang explains.
The approach was tested experimentally on five gene microarray datasets, which suffered class imbalance—with the number of samples ranging from 60 to 136 and the number of features from 2,000 to 12,600. By using an incremental approach, Yang and his team were able to significantly reduce the computational load related to feature selection from 4,215 seconds to 49 seconds.
"Due to some practical limitations, such as the very specific case of a rare disease in clinic data, many practical problems will be of high dimensionality, small sample size and class imbalance," Yang notes. "There are still issues that need to be addressed to deal with these kinds of problems."
More information: "Emphasizing minority class in LDA for feature subset selection on high-dimensional small-sized problems." IEEE Transactions on Knowledge and Data Engineering 27, 88–101 (2015). dx.doi.org/10.1109/TKDE.2014.2320732