Modelling method to address pollution monitoring systems' data loss
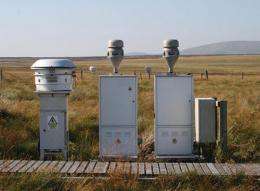
A paper published in the journal Environmental Science: Processes & Impacts by NPL's Richard Brown is featured as the cover story and named as a 'HOT article' on the journal's blog.
The paper concerns the issue of data loss in pollution monitoring systems and tests a modelling method that could help overcome the problem.
There are sometimes gaps in data sets because it is not always feasible to monitor pollutants every day and there is always the chance of an equipment malfunction or bad weather impacting a measurement. These data gaps are especially problematic when the pollutant in question varies with the time of year. One such pollutant is benzo[a]pyrene which is monitored by NPL for Defra as part of the UK PAH Monitoring Network.
This paper explores the effects of losing one month of data on the calculated annual average concentration of benzo[a]pyrene. One month of data was removed from the complete set and then models were used to fill in the gap. The predicted data was then compared to the actual data to see how accurate the modelling techniques are, and to examine the effects that this has on the final average annual concentration figures. The results show that losing data for the month of January could result in an underestimation of concentrations by as much as 16%.
NPL employs a time coverage of 100% in its operation of the UK PAH Monitoring Network. This means that it aims to make measurements every day of the year, and generates a reasonably complete data set. However, the specifications given in the European Commission's Fourth Air Quality Daughter Directive only require sampling every other month or every third month. The project results indicate that for pollutants whose concentrations vary with the seasons, such as benzo[a]pyrene, this is too infrequent to obtain reliable data if current modelling techniques are used to fill in the gaps.
The paper proposes a new modelling method that is quicker and less complex, and can predict the missing data more accurately. In months where conditions are significantly different to the average, for example with lower temperatures than usual, the accuracy needs to be further improved. This should be possible by taking factors such as temperature into account within the model.
This work will help inform the current debate around the revision of air quality monitoring guidelines and legislation, and provide some useful tools to help ensure the reliability of the collected data sets.
Read the full paper: "Data loss from time series of pollutants in ambient air exhibiting seasonality: consequences and strategies for data protection"
Provided by National Physical Laboratory