February 21, 2013 feature
To dial, perchance to group: Statistical analysis reveals clustered telephony patterns

(Phys.org)—Whether cellular calls, texting, instant messaging, there's more to communications than content: every exchange leaves behind an electronic trace that can be measured and studied. Recently, researchers led by Prof. Wei-Xing Zhou at East China University of Science and Technology and by Prof. H. Eugene Stanley at University of Boston studied intercall durations of the 100,000 most active cell phone users of a Chinese mobile phone operator. They found that these durations form three clusters – robot-based callers, telecom fraud and telephone sales – that follow a power-law distribution, but also found that calling patterns of individual users formed a fourth cluster that followed a Weibull distribution. The researchers conclude that their findings may enable a more detailed analysis of the huge body of data contained in the logs of massive numbers of users.
Dr. Zhi-Qiang Jiang discusses the challenges he and his colleagues – Prof. H. Eugene Stanley, Prof. Wei-Xing Zhou, Prof. Boris Podobnik, Wen-Jie Xie, and Ming-Xia Li – faced in conducting their study. "In our sample, there are 4,635,536 individuals that have nonempty intercall durations – that is, each has at least two calls," Jiang tells Phys.org, adding that for theoretical and practical reasons, it is not optimal to investigate individuals with low calling frequencies. Therefore, the team focused on the 100,000 most active users. While previous studies were primarily interested in collective behaviors, Jiang's research studied the individual level. "Examining the intercall duration distributions of many randomly chosen individuals showed that power-law and Weibull distributions are two suitable candidates. In order to test our conjecture, we had to design a rigorous statistical method – and this the first challenge we encountered."
Confirmation that intercall durations follow a power-law distribution with an exponential cutoff at the population level was relatively simple, Jiang continues. "Moreover, since this result is consistent with previous studies, the statistical test was also relatively simple. However," he notes, "we did not use maximum likelihood estimation." Maximum likelihood estimation (MLE) is a method of estimating the parameters of a statistical model. In addition, when applied to a dataset and given a statistical model, MLE provides estimates for the model's parameters. "Instead, we used the simple least-squares regression method because performing MLE on a sample of 100,000 individuals with numerous durations was beyond our computer's capacity."
By determining intercall duration distributions, the team was able to classify them into two groups: one with power-law intercall duration distributions and the other with Weibull distributions. (A Weibull distribution is a flexible measurement that details the continuous probability distribution associated with the lifetime characteristics of a member of a population.) "We looked at different properties of individuals' calling patterns," Jiang illustrates, "and found many differences. For instance, it's natural to investigate the data from the perspective of complex networks – and the simplest way is to check the out-degree distributions." The degree of a graph or network node is the number of connections it has to other nodes; the degree distribution is the probability distribution of these degrees over the entire network – and in a directed network, in- and out-degree refers to a node's inbound or outbound links, respectively. In this paper, the out-degree describes the number of different callees (call recipients) for a specified cell phone user. During and after the classification of the four calling patterns, the researchers examined the behaviors of the individuals in the three groups with a power-law duration distribution – robot-bases calls, telecom fraud and telephone sales – in greater detail. For example, Jiang notes, they checked the time series of call occurrence times, adding "It was another challenge to find a suitable method for further classifying the phone users."
Jiang describes the process of classifying the clusters based on statistical analysis. Because individuals in Cluster 1 were characterized by a high frequency of call initiation (out-going call mean percentage 0.99), a small number of call recipients (average number 22), and an allocation of almost all out-going calls to only one call recipient (average communication diversity value 0.015), the researchers inferred them that they were robot-based callers. By comparison, individuals in Cluster 3 characterized by a high frequency of call initiation (mean out-going call percentage 0.94), a larger number of call recipients (average number callees 2083), and an even distribution of out-going calls among all callees (average communication diversity value 0.98), they inferred them to be telecom frauds and telephone sales.
On the other hand, in the group of individual users with a Weibull duration distribution, the average number of callees, the mean percentage of outgoing calls, and the average value of communication diversity were 245, 0.57, and 0.79, respectively.
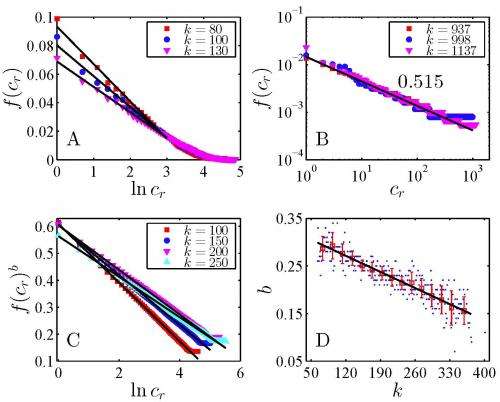
Two other interesting discoveries: The researchers found that they could determine the probability that a user will call the cr-th-most-contact (the recipient most called by an outgoing call r within cluster c) and the probability distribution of burst sizes.
Jiang summarizes the main cr-th-most-contact results by cluster as follows:
- Cluster 1: most of the calls (mean 99.5% and min 94%) are to only one contact
- Cluster 2: the number of outgoing calls to different contacts follows an exponential distribution
- Cluster 3: the number of outgoing calls to different contacts follows a power-law distribution
- Cluster 4: the number of outgoing calls to different contacts follows a stretched exponential distribution
Regarding burst size probability,
- Clusters 1 and 3: the burst size switches from a power-law distribution to an exponential distribution with the increment of time windows
- Cluster 2: the burst size follows a exponential distribution for different time windows
- Cluster 4: the burst size follows a power-law distribution for different time windows
Finally, the researchers see that their findings may enable a more detailed analysis of the huge body of data contained in the logs of massive users. "Our analysis of the massive data of calls enables us to gain insights into the investigation of other massive data sets," Zhou says, "such as stock traders and massively multiplayer online role-playing game users. However," he acknowledges, "the methods used in our paper might not be able to be directly applied to other complex systems. It's very possible that we'll need to further develop new methods and techniques."
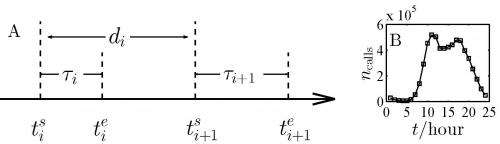
Moving forward, Zhou continues, the researchers plan to perform further investigations on the calling behaviors of individuals and the complexity of the communication networks. "We also plan to investigate the mobility behaviors of individuals to have a better understanding of human mobility patterns. It would also be a very interesting topic to understand the spatiotemporal dynamics of human communication and mobility."
Jiang and his colleagues also believe that their highly-interdisciplinary work represents a significant scientific step forward. "It involves topics that range from complex systems to human dynamics, and also enriches our understanding on the individuals whose activity patterns are dominated by the power-law distribution of inter-event time. Moreover, it proposes a new approach to understanding individual behaviors from the big data contained in the logs of massive users, and provides a framework for constructing models to explain the empirical collective behaviors based on clusters of, rather than all, individuals."
The team also views work as significant in a practical sense. "Mobile phone service providers can use the idea to identify illegal users and to design their sales strategies," Podobnik concludes. "We believe that having better insight into mobile phone dynamics can help mobile operators become even more efficient, and perhaps even help them reduce their costs and more easily deal with spam, which are also part of our studies."
More information: Calling patterns in human communication dynamics, PNAS January 29, 2013 vol. 110 no. 5 1600-1605, doi:10.1073/pnas.1220433110
Journal information: Proceedings of the National Academy of Sciences
Copyright 2013 Phys.org
All rights reserved. This material may not be published, broadcast, rewritten or redistributed in whole or part without the express written permission of Phys.org.