Researcher develops computational text analysis method made possible regardless of language or domain
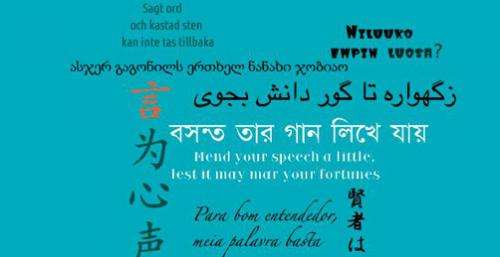
The Internet is awash with text. Databases swell larger and larger by the minute. How can the vast amount of textual data be systematically analysed and managed, as the number of languages, domains, styles and dialects is getting countless? The task is too much for the human brain. Traditional methods for textual analysis run short. What we need are statistical methods, data mining and machine learning.
Mari-Sanna Paukkeri has studied how textual data can be processed and analysed automatically with machine learning methods. In her doctoral dissertation for the Aalto University Department of Information and Computer Science, Paukkeri has developed computational methods for text processing independent of language or domain.
With these methods, textual data sets are mined with algorithms for statistical dependencies and structures, from which specific properties of texts can then be extracted.
"Languages appear to be alike: sequential symbols form words, which build up to sentences. Large masses of text are examined for co-occurrences and structures in the use of language in order to make sense of individual sentences and words," sums up Paukkeri the principle of computational linguistics.
"Precisely these co-occurrences in language enable the computational study of texts regardless of the language or domain."
Unsupervised machine learning extracts relevant information from massive textual data sets
Paukkeri has especially studied the applicability of unsupervised machine learning to natural language processing. The field has traditionally made use of rule-based methods, in which the words and structures to be sought for are manually pre-defined for the data processing models.
"In unsupervised machine learning methods, the data set is not manually pre-processed in any way: the algorithms are left to their own devices to find out what the data is like and what kind of statistical dependencies and structures it holds. The methods are not told whether they are performing correctly or not; they work independently, without manual labour," explains Paukkeri.
Paukkeri finds an analogy for unsupervised machine learning in the way a child learns to use language.
"A child does not dabble in language grammar first, but imitates, experiments and combines fragments."
In Paukkeri's dissertation a method called Likey, co-developed with her colleagues and her supervisor Docent Timo Honkela from Aalto University, is applied to keyphrase and keyword extraction from text documents of 11 different languages.
"Likey finds out how common certain words and pairs, threes and fours of words are in a data set. This way it defines the keywords and phrases for a specific document – solely on the basis of their frequency and context in the text."
An everyday example of very refined computational unsupervised text processing would be Google's translation application. The translations are based on the automatically analysed, enormous amount of text the search engine has in its use.
"Companies also have an awful lot of text tucked away in their databases, usually with only a simple search functionality to utilise them. These databases exceed human management abilities, but with my methods they could be categorised and analysed."
Global companies in particular could benefit from methods with which to process their textual data in all of their working languages around the world.
Also the subjective variation of language use is within the grasp of computational methods. Paukkeri has studied the automatic assessment of difficulty and comprehension of texts aimed both at experts and lay people. Paukkeri's research experiments on medical texts, but the method is, again, independent of the domain.
"A search engine could predict the knowledge level of each user and customise the difficulty of the search results accordingly."
Language-independent text mining could also, according to Paukkeri, contribute to the discovery of language universals, features that are common to all languages. They could be mined from data sets consisting of hundreds or even thousands of languages.
"Who says we cannot apply our knowledge of structural and lexical similarities between languages for machine learning systems? That is what people do as well when learning new languages," ponders Paukkeri.
Provided by Aalto University