November 19, 2008 feature
Professor Finally Publishes Controversial Brain Theory
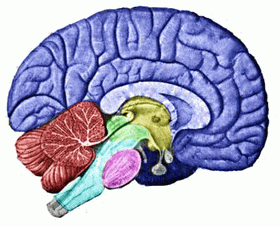
(PhysOrg.com) -- In the late '90s, Asim Roy, a professor of information systems at Arizona State University, began to write a paper on a new brain theory. Now, 10 years later and after several rejections and resubmissions, the paper “Connectionism, Controllers, and a Brain Theory” has finally been published in the November issue of IEEE Transactions on Systems, Man, and Cybernetics – Part A: Systems and Humans.
However, Roy’s controversial ideas on how the brain works and learns probably won’t immediately win over many of his colleagues, who have spent decades teaching robots and artificial intelligence (AI) systems how to think using the classic connectionist theory of the brain. Connectionists propose that the brain consists of an interacting network of neurons and cells, and that it solves problems based on how these components are connected. In this theory, there are no separate controllers for higher level brain functions, but all control is local and distributed fairly equally among all the parts.
In his paper, Roy argues for a controller theory of the brain. In this view, there are some parts of the brain that control other parts, making it a hierarchical system. In the controller theory, which fits with the so-called computational theory, the brain learns lots of rules and uses them in a top-down processing method to operate. In 1997, IBM’s Deep Blue computer, which famously defeated world chess champion Garry Kasparov, operated based on countless rules entered by its programmers.
Despite the success of the rule-based AI system in chess, no AI system has come close to learning and interacting with the world at the human level, using either the connectionist approach or the computational approach. Although the human brain may not serve as the best model for AI systems, a human-like machine should, by its very nature, be patterned after the human brain.
Brains without external babysitters
In his paper, Roy shows that the connectionist theory actually is controller-based, using a logical argument and neurological evidence. He explains that some of the simplest connectionist systems use controllers to execute operations, and, since more complex connectionist systems are based on simpler ones, these too use controllers. If Roy’s logic correctly describes how the brain functions, it could help AI researchers overcome some inherent limitations in connectionist algorithms.
“Connectionism can never create autonomous learning machines, and that’s where its flaw is,” Roy told PhysOrg.com. “Connectionism requires human babysitting of their learning algorithms, and that’s not very brain-like. We don’t guide and control the learning inside our head. Wish we could tweak our brain from outside, but we can’t.”
In his argument, Roy uses examples of a human using a TV remote control or driving a car to demonstrate a general controller-based system. In these systems, the human is the controller, whether changing the TV channels or accelerating the vehicle, while the TV and car are the subservient systems.
In response, connectionists have argued that such systems are not controller-based, but connectionist – or, more specifically, that these are feedback systems, where the components are codependent on each other. In the examples, the TV screen displays the show on that channel, which the human sees and decides whether or not to change the channel again. Or, the car’s speedometer registers 25 mph, which the driver sees and decides whether to accelerate or slow down. This feedback is essential for the human to act, connectionists argue, making the notion of a single controller in the system meaningless.
However, Roy’s response is that the controller doesn’t necessarily need feedback to control the TV or car. The human can act completely arbitrarily without feedback, such as by closing his eyes, and still continuing to change channels and press the accelerator. The key, Roy emphasizes, is that the controller has the ability to act in an arbitrary mode.
Self-supervision
He then examines a simple connectionist learning method called the back-propagation algorithm. This method consists of an interconnected network (for example, of neurons) but also uses an external supervisor when the network makes an error. The supervisor determines which neurons made the error, and then these neurons alter their inputs in an attempt to reduce the system error and come closer to the desired output.
In this algorithm, connectionists see the supervisory abilities as distributed throughout the entire learning system, since the system uses a feed-forward approach to respond to an error in a pre-defined way. But Roy argues that there is a distinct supervisor that has the ability to act in an arbitrary manner, completely neglecting the types of errors the network generates. Therefore, he sees the supervisor as the controller.
Roy acknowledges that only neuroscience – not information science – can determine if connectionist algorithms such as the back-propagation algorithm actually exist in the brain. But if they do, he says that they must rely on controllers.
He highlights evidence from a variety of neuroscience studies that support the existence of controllers in the brain. For example, past research has proposed the existence of control centers of the brain, such as the prefrontal portion of the cerebral cortex. Studies of dopamine and other neural transmitters, as well as the presence of “neurogenesis” (cell birth) in adults, are also compatible with a controller-based brain theory.
Roy clarifies that there is not necessarily a single executive controller in the brain, but rather that multiple distributed controllers could be responsible for different subsystems of the brain. After all, he says, if the connectionist theory is correct and the brain is a network of changing connections, there must be some control mechanism to determine how all those connections are made.
“The controller theory actually takes down a big chunk of connectionism, although not all of it,” Roy said. “The parallel computing idea, within a network of neurons, is still valid. But the controller theory allows one to design and train neural networks in a completely different way than connectionism. So it almost becomes a new science, and we are looking forward to a new generation of human-like learning algorithms.”
Resistance to a new science
Roy’s theory undermines the roots of connectionism, and that’s why his ideas have experienced a tremendous amount of resistance from the cognitive science community. For the past 15 years, Roy has engaged researchers in public debates, in which it’s usually him arguing against a dozen or so connectionist researchers. Roy says he wasn’t surprised at the resistance, though.
“I was attempting to take down their whole body of science,” he explained. “So I would probably have behaved the same way if I were in their shoes.”
One reason why it was so difficult for researchers to accept his theory is that, in cognitive science, terms are not always defined in a very strict way like in other sciences, he explained. He says he ran into many circular arguments with reviewers regarding his paper, and that’s why much of the paper is dedicated to defining what a controller is.
“It’s not that connectionism does not use controllers,” Roy says. “They do, but they use them at the level of individual neurons and call the whole thing as distributed control. And that kind of control is fine with them. They visualize each neuron as ‘deciding’ on its own how to adjust connection strengths during learning, but have a hard time accepting the notion that some neurons in the brain could be sending instructions to some other neurons and tell them what to do. That is, until I showed them that that is exactly what they are doing in their systems, and that there is also growing neuroscience evidence for signals coming from elsewhere in the brain.”
However, Roy’s controller theory may not be quite as at odds with some connectionist’s perspectives as he supposes. Psychology Professor James McClelland at Stanford University, whose early work Roy cites in his paper, thinks that modern connectionist thought allows for some controlling parts of the brain, though not to the extent as in Roy’s model.
“Roy appears to be using a quote from our 1986 book [see below] to mischaracterize our position,” McClelland said. “Work shortly after the publication of our 1986 book began to address the issue of cognitive control. I still favor the view that control is an emergent function of neural populations distributed over several brain areas, but there is no doubt that some parts of the system (most notably, left lateral inferior prefrontal cortex) play a special role in control. Roy's position appears more modular than ours, but I don't think there's anyone who disputes the idea that there are mechanisms that exert some degree of control over cognition.”
Neuroscientist Walter J. Freeman of the University of California at Berkeley also said that he agreed with the notion that there are controllers or guidance systems within the brain. Freeman, who has taught brain sciences at Berkeley since 1959, has developed a model of the brain’s intentional system. The model involves a control loop that predicts future states, future sensory input and future plans of action. The spatiotemporal pattern that implements this plan is transmitted by cortical neurons into the brain stem and spinal cord, using feedback from various parts of the brain. So guidance, control and monitoring of actions play an important part in Freeman’s model.
Autonomous learning machines
No matter exactly where or what the brain controllers are, Roy hopes that his theory will enable research on new kinds of learning algorithms. Currently, restrictions such as local and memoryless learning have limited AI designers, but these concepts are derived directly from that idea that control is local, not high-level. Possibly, a controller-based theory could lead to the development of truly autonomous learning systems, and a next generation of intelligent robots.
“The controller theory gives us much more freedom in creating brain-like learning systems,” he said. “The science is currently stuck, and we have not made any significant progress towards creating robots that can learn on their own like humans.”
The sentiment that the “science is stuck” is becoming common to AI researchers. In July 2007, the National Science Foundation (NSF) hosted a workshop on the “Future Challenges for the Science and Engineering of Learning.” The NSF’s summary of the “Open Questions in Both Biological and Machine Learning” [see below] from the workshop emphasizes the limitations in current approaches to machine learning, especially when compared with biological learners’ ability to learn autonomously under their own self-supervision:
“Virtually all current approaches to machine learning typically require a human supervisor to design the learning architecture, select the training examples, design the form of the representation of the training examples, choose the learning algorithm, set the learning parameters, decide when to stop learning, and choose the way in which the performance of the learning algorithm is evaluated. This strong dependence on human supervision is greatly retarding the development and ubiquitous deployment of autonomous artificial learning systems. Although we are beginning to understand some of the learning systems used by brains, many aspects of autonomous learning have not yet been identified.”
Roy sees the NSF’s call for a new science as an open door for a new theory, and he plans to work hard to ensure that his colleagues realize the potential of the controller model. Next April, he will present a four-hour workshop on autonomous machine learning, having been invited by the Program Committee of the International Joint Conference on Neural Networks (IJCNN).
“At this time, the plan is to show this community that it is feasible to construct machines that can learn on their own like humans,” he said. “I did a similar workshop last week at ANNIE (Artificial Neural Networks in Engineering), and I had people come up to me and say that they would like to automate their learning algorithms in a similar way. So, at this point, the focus is to build a worldwide team of researchers to collaborate on this new science and move aggressively towards building human-like robots (software and hardware) that can learn on their own. The applications of these systems would be limited only by imagination.”
More information: Roy, Asim. “Connectionism, Controllers, and a Brain Theory.” IEEE Transactions on Systems, Man, and Cybernetics – Part A: Systems and Humans, Vol. 38, No. 6, November 2008.
Rumelhart, D. E. and J. L. McClelland, Eds., Parallel Distributed Processing: Explorations in Microstructure of Cognition, vol. 1. Cambridge, MA: MIT Press, 1986, pp. 318–362.
NSF’s summary of the “Open Questions in Both Biological and Machine Learning” www.cnl.salk.edu/Media/NSFWorkshopReport.v4.pdf
ANNIE Conference Web site annie.mst.edu/annie_2008/ANNIE2008.html
Copyright 2008 PhysOrg.com.
All rights reserved. This material may not be published, broadcast, rewritten or redistributed in whole or part without the express written permission of PhysOrg.com.