New algorithm improves the way computers interpret readings of the brain’s electrical signals
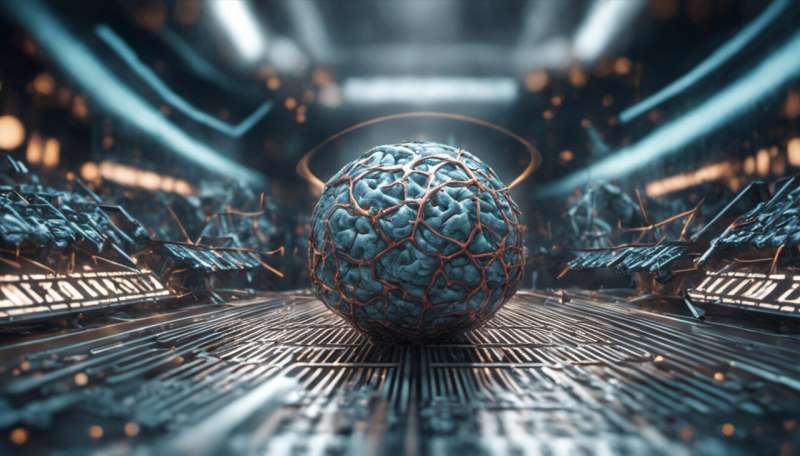
Electroencephalography (EEG) records the electrical signals produced by the brain using an array of electrodes placed on the scalp. Computers use an algorithm called common spatial pattern (CSP) to translate these signals into commands for the control of various devices.
Haiping Lu at the A*STAR Institute for Infocomm Research and co-workers have now developed an improved version of CSP for classifying EEG signals. The new algorithm will facilitate the development of advanced brain–computer interfaces that may one day enable paralyzed patients to control devices such as computers and robotic arms.
CSP distinguishes and interprets commands by estimating the variations between EEG signals, and its accuracy strongly depends on how many signals are provided. As a result, CSP may make an incorrect interpretation when the number of EEG signals is small.
The new CSP algorithm developed by Lu and his colleagues uses two parameters to regularize the estimation of EEG signal variations. One parameter lowers the variations of the estimates, while the other reduces the tendency of the CSP algorithm to bias the estimates towards values from only a small number of samples.
Together, these parameters significantly improve the accuracy of CSP for classifying EEG signals. The researchers optimized the new algorithm even further by aggregating a number of different regularizations.
They evaluated their algorithm in the third Brain–Computer Interface Competition, held in 2005. The competition enables researchers who are developing advanced brain–computer interface technologies to test their methods for processing and classifying EEG signals using publicly available data sets.
The algorithm developed by Lu’s group significantly out-performed four other groups in three sets of experiments with varying testing scenarios, and its superiority was particularly evident when the number of sample EEG signals was small.
Conventional algorithms require 20–90 EEG signals, but the algorithm of Lu and co-workers needs only ten. This significantly reduces the effort required to collect data for brain–computer interfaces, the memory requirements for EEG signal processing applications, and the processing time needed for processing the signals.
“This is a method to improve the accuracy of current brain–computer interfaces,” says Lu. “Our algorithm applies ensemble-based learning in the feature-extraction stage of an EEG-based brain–computer interface, which could be integrated with training data ensembles in the data pre-processing stage. It would be among many other improvements to be tested and used in existing brain–computer interface systems.”
More information: Lu, H., et al. Regularized common spatial pattern with aggregation for EEG classification in small-sample setting. IEEE Transactions of Biomedical Engineering 57, 2936–2946 (2010) dx.doi.org/10.1109/TBME.2010.2082540
Provided by Agency for Science, Technology and Research (A*STAR)