This article has been reviewed according to Science X's editorial process and policies. Editors have highlighted the following attributes while ensuring the content's credibility:
fact-checked
proofread
Enhancing model performance and data efficiency through standardization and centralization
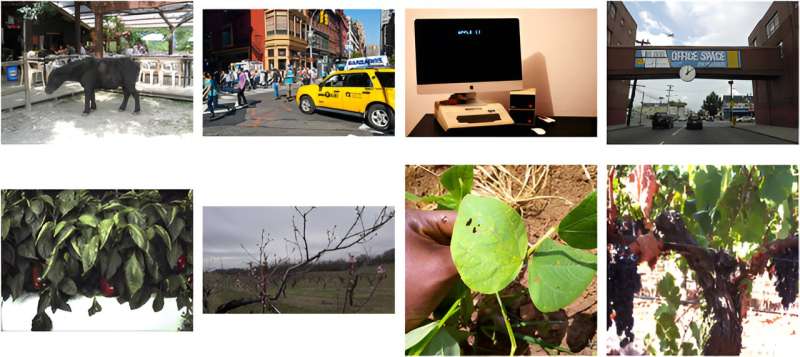
Recent advancements in agricultural computer vision have heavily relied on deep learning models, which, despite their success in general tasks, often lack agricultural-specific fine-tuning. This results in increased training time, resource use, and lower performance due to the reliance on weights from non-agricultural datasets.
Though transfer learning has proven effective in mitigating data gaps, the current research emphasizes the inadequacy of existing pre-trained models in capturing agricultural relevance and the absence of a substantial, agriculture-specific dataset. Challenges include insufficient task-specific data and uncertainties regarding the efficacy of data augmentation in agricultural contexts.
To tackle these issues, exploring alternative pre-trained model strategies and establishing a centralized agricultural dataset is imperative to enhance data efficiency and bolster model performance in agriculture-specific tasks.
In a study published in Plant Phenomics, the researchers created a novel framework for agricultural deep learning by standardizing a wide range of public datasets for three distinct tasks and constructing benchmarks and pre-trained models.
They employed commonly used deep learning methods, yet unexplored in agriculture, to enhance data efficiency and model performance without major alterations to existing pipelines. The research showcased that standard benchmarks enable models to perform comparably or better than existing benchmarks, with these resources made available through AgML (github.com/Project-AgML/AgML).
For object detection, agricultural pre-trained weights substantially outperformed standard baselines, achieving quicker convergence and higher precision, especially for certain fruits. Similarly, in semantic segmentation, models with agricultural pretrained backbones outperformed those with general backbones, indicating swift performance improvements.
These findings underscore that even subtle adjustments to training processes can significantly enhance agricultural deep learning tasks. The study also delved into the efficacy of data augmentations, revealing that spatial augmentations outperformed visual ones, suggesting their potential to enhance model generalizability and performance in diverse conditions.
However, the impact varied across tasks and conditions, highlighting the nuanced nature of augmentation application. Additionally, researchers explored the effects of annotation quality, revealing that models could still perform well even with lower-quality annotations, suggesting a potential for broader data use and annotation strategies.
In summary, this work not only advances the field of agricultural deep learning through a novel set of standardized datasets, benchmarks, and pretrained models but also provides a practical guide for future research. By demonstrating that minor training adjustments can lead to significant improvements, pathways have been opened for more efficient and effective agricultural deep learning, ultimately contributing to the broader goal of advancing agricultural technology and productivity.
More information: Amogh Joshi et al, Standardizing and Centralizing Datasets for Efficient Training of Agricultural Deep Learning Models, Plant Phenomics (2023). DOI: 10.34133/plantphenomics.0084
Provided by TranSpread