This article has been reviewed according to Science X's editorial process and policies. Editors have highlighted the following attributes while ensuring the content's credibility:
fact-checked
proofread
Reactor physics research: Advanced neural networks reveal new potential in solving K-eigenvalue problems
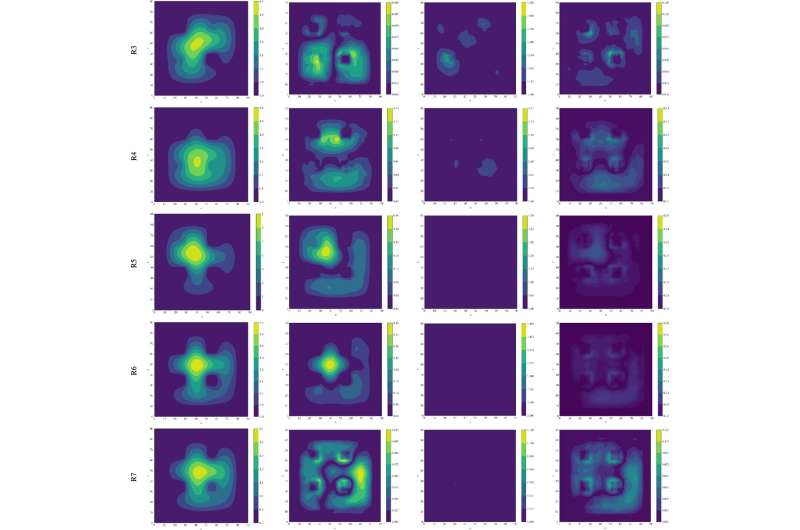
In a new study of reactor physics published in the journal Nuclear Science and Techniques, researchers from Sichuan University, Shanghai Jiao Tong University, have introduced two innovative neural networks to address the longstanding challenges associated with K-eigenvalue problems in neutron diffusion theory. These problems, which are fundamental in the nuclear engineering realm, are pivotal for the simulation and analysis of nuclear reactors.
This study introduced two pioneering neural networks, the Generalized Inverse Power Method Neural Network (GIPMNN) and its advanced version, the Physics-Constrained GIPMNN (PC-GIPMNN), to address challenges in reactor physics. While the GIPMNN utilizes the inverse power method to iteratively pinpoint the lowest eigenvalue and the associated eigenvector, the PC-GIPMNN elevates this approach by seamlessly incorporating conservative interface conditions.
This advancement proves crucial when tackling interface challenges inherent in reactors with varied fuel assemblies. Notably, in a side-by-side performance evaluations across intricate spatial geometries, PC-GIPMNN consistently surpassed both its counterpart GIPMNN and others. This study opted for a data-independent approach, focusing purely on mathematical and numerical solutions, thereby eliminating potential biases.
These findings herald a new era in nuclear reactor physics, paving the way for enhanced understanding and more streamlined simulations. The adaptability of the introduced neural networks hints at their potential use in other scientific arenas grappling with interface challenges. In essence, the study spotlights the revolutionary promise of neural networks in reactor physics. Future endeavors will indubitably refine these networks and probe their effectiveness in increasingly intricate scenarios.
More information: Qi-Hong Yang et al, Physics-constrained neural network for solving discontinuous interface K-eigenvalue problem with application to reactor physics, Nuclear Science and Techniques (2023). DOI: 10.1007/s41365-023-01313-0
Provided by TranSpread