Wildfire dataset could help firefighters save lives and property
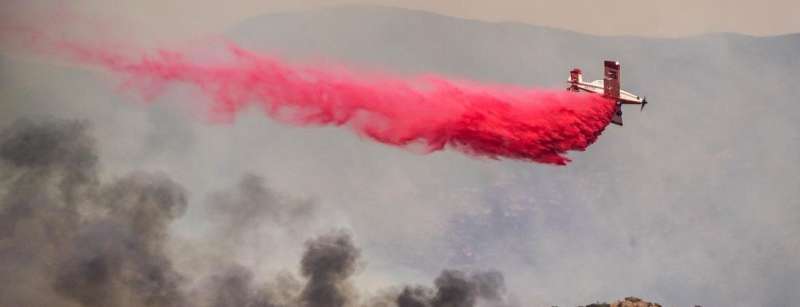
A team at UC Riverside led by computer science assistant professor Ahmed Eldawy is collaborating with researchers at Stanford University and Vanderbilt University to develop a dataset that uses data science to study the spread of wildfires. The dataset can be used to simulate the spread of wildfires to help firefighters plan emergency response and conduct evacuation. It can also help simulate how fires might spread in the near future under the effects of deforestation and climate change, and aid risk assessment and planning of new infrastructure development.
The open-source dataset, named WildfireDB, contains over 17 million data points that capture how fires have spread in the contiguous United States over the last decade. The dataset can be used to train machine learning models to predict the spread of wildfires.
"One of the biggest challenges is to have a detailed and curated dataset that can be used by machine learning algorithms," said Eldawy. "WildfireDB is the first comprehensive and open-source dataset that relates historical fire data with relevant covariates such as weather, vegetation, and topography."
First responders depend on understanding and predicting how a wildfire spreads to save lives and property and to stop the fire from spreading. They need to figure out the best way to allocate limited resources across large areas. Traditionally, fire spread is modeled by tools that use physics-based modeling. This method could be improved with the addition of more variables, but until now, there was no comprehensive, open-source data source that combines fire occurrences with geo-spatial features such as mountains, rivers, towns, fuel levels, vegetation, and weather.
Eldawy, along with UCR doctoral student Samriddhi Singla and undergraduate researcher Vinayak Gajjewar, utilized a novel system called Raptor, which was developed at UCR to process high-resolution satellite data such as vegetation and weather. Using Raptor, they combined historical wildfires with other geospatial features, such as weather, topography, and vegetation, to build a dataset at a scale that included the most of the United States.
WildfireDB has mapped historical fire data in the contiguous United States between 2012 to 2017 with spatial and temporal resolutions that allow researchers to home in on the daily behavior of fire in regions as small as 375-meter square polygons. Each fire occurrence includes type of vegetation, fuel type, and topography. The dataset does not include Alaska or Hawaii.
To use the dataset, researchers or firefighters can select information relevant to their situation from WildfireDB and train machine learning models that can model the spread of wildfires. These trained models can then be used by firefighters or researchers to predict the spread of wildfires in real time.
"Predicting the spread of wildfire in real time will allow firefighters to allocate resources accordingly and minimize loss of life and property" said Singla, the paper's first author.
A visualization of the dataset is available here.
More information: WildfireDB: An Open-Source Dataset Connecting Wildfire Spread with Relevant Determinants. ayanmukhopadhyay.github.io/files/neurips2021.pdf
Provided by University of California - Riverside