Underground noise reveals fracture pathways needed for energy production
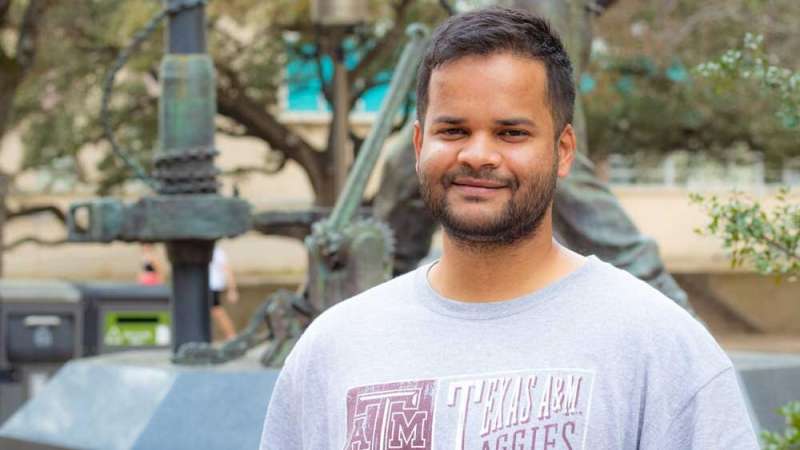
Both oil production and geothermal energy need fluids to move through fracture channels within subsurface rocks. Yet accurately mapping and measuring fractures created for fluid flow is challenging, because what happens underground goes unseen.
Seismic surveys, created when sound bounces against subsurface features, can produce reflective imaging, but these are not detailed pictures and are difficult to decipher by anyone untrained in geosciences.
Texas A&M University graduate researcher Aditya Chakravarty believes a different sound source will create clearer and more accurate pictures, at least when it comes to depicting fractures. Under the supervision of his mentor, Siddharth Misra of the Harold Vance Department of Petroleum Engineering, Chakravarty is using machine-learning algorithms to focus on the passive sounds made by rocks as they crack and break underground so the fractures can be accurately assessed and mapped.
The research is supported by a grant awarded to Misra from the Department of Energy (DOE) and continues work Chakravarty did as an intern for the Lawrence Berkeley National Lab during the summer of 2020. Both the current research and the internship are associated with the Enhanced Geothermal Systems Collaborative project (EGS Collab) created by the DOE Geothermal Technologies Office.
While it may seem strange for a student getting his doctoral degree in petroleum engineering to participate in a project advancing geothermal methods so they may one day power millions of homes, Chakravarty disagrees.
"Petroleum engineers have a very solid grasp of underlying geothermal energy concepts, like drilling completions, fluid flows and so on," he said. "I found out most of the leading people in geothermal projects previously worked as petroleum engineers or reservoir engineers."
Fluid flows have been studied in enough detail to have well-defined equations available to predict their behavior, but fluid flow travel in underground reservoirs can be tricky. Many reservoir rocks are so tightly-grained that fluids can't flow through them unless they are cracked or fractured. Most of these rocks are also highly heterogeneous, meaning of all different types, so the fracture shapes, sizes and lengths can be quite varied and, consequently, difficult to predict. Getting oil to flow out of a tightly compacted shale reservoir or water to flow down through hot rock to become an upward vent of steam depends on better visualization of the actual fracture channels underground.
"To understand and be sure that we create the right fractures, we need to be able to image and characterize them properly," Chakravarty said. "For example, we may pump in water to create steam, but the fluid will just get lost below ground if we don't have fractures in the right places to guide it back to the geothermal plant."
Chakravarty is working with data collected by the EGS Collab from the Sanford Underground Research Facility at Homestake Mine in South Dakota, where injection, fracture and production tests were performed, monitored and recorded at depths of over 4,800 feet. His role is to apply blind, or unsupervised, machine-learning methods to these underground data measurements to better understand what the signatures say about the state of the fractures in the subsurface.
There are two broad types of machine-learning algorithms: supervised and unsupervised. Supervised machine learning is somewhat like taking an exam where all the questions were covered somewhere within a memorized textbook: everything is known, it just has to be identified. Unsupervised learning deals with things that are not known but could be relevant. The algorithms must filter data through a general understanding of a subject and extract what seems important from what probably isn't.
Because of the complexities of understanding the unseen fractures underground, unsupervised learning is perfect for this job. The algorithms sift through the data collected by the EGS Collab measuring equipment and sort out what they find based on guidance from Chakravarty.
"With clever processing, pieces of the puzzle appeared from the random underground noise and started telling a tightly coherent story," said Chakravarty. "For me, the petroleum engineering student who has limited exposure to seismology, it's an epiphany."
Chakravarty might not be adept at seismology, but he does have a bachelor's and master's degree in geoscience and a master's in petroleum engineering. He came to Texas A&M to finish his petroleum engineering education and, after working for Misra on a machine-learning project based on using passive sounds to characterize cracks in rock, found a deep appreciation for the science. Chakravarty was able to get a coveted internship with Lawrence Berkeley to further his interests in machine learning and it introduced him to the EGS Collab project.
He is proud of his affiliation with the geothermal project and advocates the advantages of a broad-based education.
"I have this rather interdisciplinary approach, which involves geophysics and petrophysics, as well as the core engineering concepts," Chakravarty said. "This gives me an appreciation of how all these different disciplines come together and make sense of what's going on."
Provided by Texas A&M University