Financial crashes, pandemics, Texas snow: How math could predict 'black swan' events
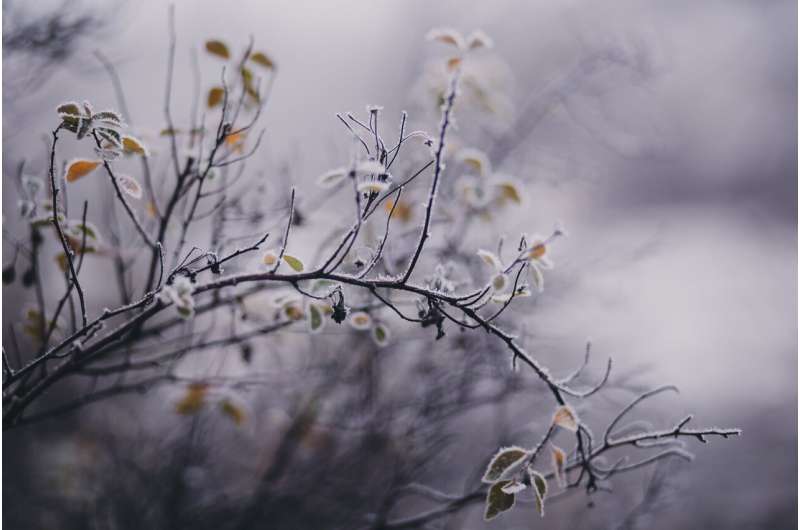
What if mathematicians could have seen COVID-19 coming, or could predict the next outbreak? Is it possible that numbers, manipulated by statistics, might warn of future market fluctuations and environmental disasters, or herald vast shifts in finance, trade, and employment?
It's hard to predict the details of an individual extreme event, but patterns emerge when many such occurrences are studied collectively. A key to understanding some of these patterns is the theory of heavy-tailed distributions, a genre of statistics that deals with exceptional "black swan" events. In a new study, Rockefeller's Joel E. Cohen and colleagues at Columbia University and Cornell University demonstrate that it is possible to predict the patterns of heavy-tailed events with established mathematical techniques.
The discovery raises the prospect that mathematical modeling may one day help scientists anticipate and manage a wide range of extreme occurrences—"from daily precipitation to microbial evolution, from cortical oscillations in the human brain to global pandemics," Cohen says. "Many extreme events are not described by standard curves. We are unprepared for extremes socially, institutionally and, too often, scientifically."
Predicting the (seemingly) unpredictable
A powerful tool for summarizing statistical variability is Taylor's Law, a simple mathematical formula that relates a population's mean to its variance—a measure of the scatter around the average. Taylor's Law describes how cancer cells and infectious diseases multiply; how crop yields fluctuate; and even how tornado outbreaks vary. It has become a tool in agricultural science worldwide, guiding sampling of insects and efficient pest control.
Scientists have long assumed that Taylor's Law works only when the measured quantities have finite averages and variances, as when measuring the heights of people. There is a finite limit to how tall or how short a human can be. If you measure enough heights of people of the same age, the result will be a roughly bell-shaped curve in which most heights cluster around an average—at the apex of the bell—and a few very short or very tall individuals have heights in the "tails" to the left and right of the curve's center. The more people measured, the more the average heights converge to a central height.
Pandemics, fires, floods, storms, and market fluctuations are different. There is no finite limit to how high the numbers might be and therefore, unlike the classic example of collecting heights, "the more you sample, the more extreme are the biggest events, and your sample's average and variance get larger and larger, marching off toward infinity," Cohen says.
The more earthquakes we experience, the greater the chances of recording one so powerful that it drives up the cumulative average size of all earthquakes, bending the standard bell curve entirely out of shape. The more we expose ourselves to the viruses of non-human animals, the more likely we are to be infected by a novel coronavirus that upends our lives.
Until now, Taylor's Law was thought to have no place in these heavy-tailed systems. It helped plot our paths along the normal circumstances of daily life, but when it came to extreme occurrences like the present pandemic, Taylor's Law seemed irrelevant.
The world of heavy tails
But a few years ago, Cohen and colleagues at Columbia University made a striking discovery—a way of looking at heavy-tailed variables that yields surprisingly orderly connections between the mean and the variance. "It was as if we took all of the pieces of a car, put it in a box, and the car still ran," Cohen says. "This combination of variables gave us the same result regardless of how they were connected."
A collaboration of excited mathematicians culminated in this new study, which collects many more examples of the phenomenon and concludes with mathematical proof that extreme, heavy-tailed events are indeed well described by Taylor's Law.
This does not mean that any individual extreme event can be predicted with a simple mean-to-variance formula. But the research effectively breaks Taylor's Law out of its shell, giving scientists good reason to test whether market fluctuations and natural disasters obey the same Taylor's Law that governs insect populations and the progression of cancerous growths.
Cohen hopes that this work will stimulate further basic research on the mathematics of heavy-tailed distributions and that scientists will use it to better understand the extreme events wherever heavy-tailed distributions lurk. "Advances like these are the mathematical analogue of bioimaging," he says.
"They make it possible to see what was previously invisible."
More information: Joel E. Cohen et al. Heavy-tailed distributions, correlations, kurtosis and Taylor's Law of fluctuation scaling, Proceedings of the Royal Society A: Mathematical, Physical and Engineering Sciences (2020). DOI: 10.1098/rspa.2020.0610
Provided by Rockefeller University