Algorithm could identify disease-associated genes
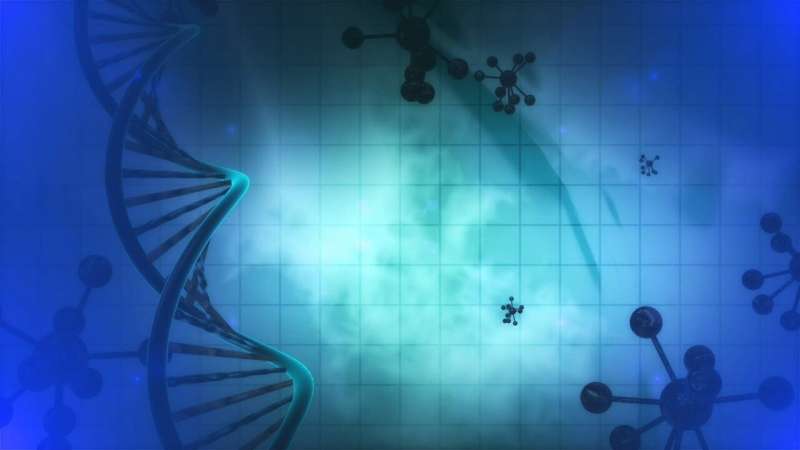
ITMO University's bioinformatics researchers have developed an algorithm that helps to assess the influence of genes on processes in the human body, including the development of disease. The research was published in BMC Bioinformatics.
Diseases or predisposition to hair loss, obesity or bad eyesight can be associated with specific genes. In order to affect them and influence a person's condition, it's necessary to identify the relevant part of the genome from among many suspects. What's more, for the purposes of determining whether there's a connection between a gene and a condition, it's important to know how genes interact among themselves.
"All in all, a human has over 20,000 genes. By comparing the genes of patients relevant conditions with the genes of healthy people, we can see the differences in activity and manifestation between the samples. Based on this information, a common graph is created that shows the interconnections between all genes, and every gene is assigned a weight factor. Usually, scientists continue to work only with the most active genes, making a special subgraph of them. However, by breaking these genes away from the 'common background," we lose the opportunity to assess the correlation of every gene with the others and the diagnoses we study," explains Alexey Sergushichev, assistant professor at ITMO.
Instead of focusing only on one system of genes with the highest weight factor, bioinformatics researchers from ITMO University have proposed a new method in which hundreds of thousands of subgraphs are generated with the use of data on the whole genome. The new algorithm, which is based on a Markov chain Monte Carlo method, makes it possible to calculate the probability of a connection between every sample with the condition in question and analyse a sample's composition with regard to the interactions between every gene.
"Imagine that you are trying to assemble a ship in a bottle. You can use a pair of tweezers, or you can just shake the bottle. When the pieces fall in place as we want them to, we fix the system in this condition and continue shaking. If we don't like what we get, we start all over. Sooner or later, we get something resembling a ship. Our program is somewhat similar. We remove one gene from a set. If the number of active genes increases, it means we did right, and we save the result. If not—we continue. In several steps, the weight factor can start growing rapidly. This way, the algorithm produces lots of graphs," explains Nikita Alexeev, a senior researcher and participant of the ITMO Fellowship and Professorship program.
With such a sample group, scientists can identify the genes that appear there more often than others. If a gene appears in 90% of such subgraphs, then the scientists can be 90% sure of its connection with the condition in question.
The project's authors note that in the future, the algorithm can be represented as a program with a slider that will allow users to produce results with various levels of confidence for various purposes.
"For example, the lower the confidence level, the more genes are shown, and vice versa. If we need to identify only the genes that we are confident in, we would set the confidence level at about 99%," concludes Nikita Alexeev.
More information: Nikita Alexeev et al. Markov chain Monte Carlo for active module identification problem, BMC Bioinformatics (2020). DOI: 10.1186/s12859-020-03572-9
Provided by ITMO University