ARIEL exoplanet mission celebrates machine learning challenge and citizen science launch
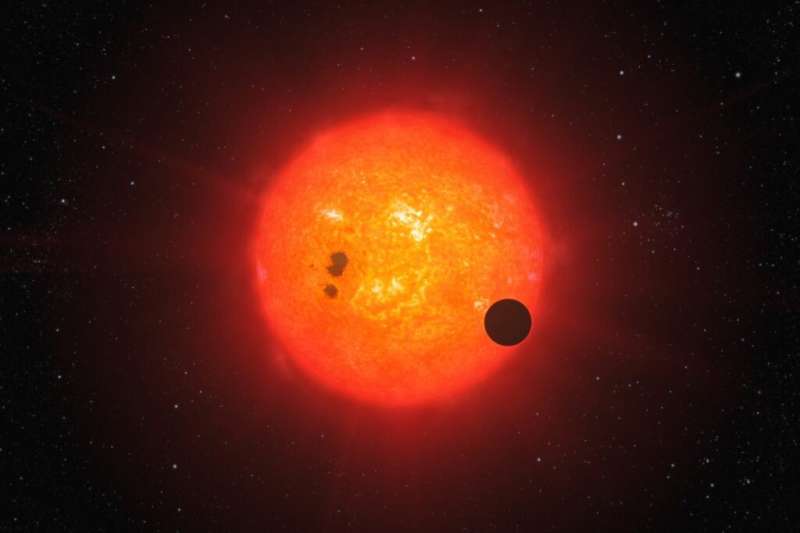
ARIEL, an ESA mission to make the first large-scale survey of exoplanet atmospheres, has announced the winners of its first international Machine Learning Data Challenge and has launched a new project, ExoClocks, aimed at amateur astronomers and citizen scientists.
The winners of the Data Challenge, James Dawson (Team SpaceMeerkat), and Vadim Borisov (Team major_tom), were announced today at the EPSC-DPS Joint Meeting 2019 in Geneva. The pair topped the leader-board for the competition out of 112 registered individuals and teams. The Data Challenge, launched in April, tackled the problem of removing noise from exoplanet observations caused by starspots and by instrumentation.
Nikos Nikolaou of the UCL Centre for Exochemistry Data, who devised the competition, said, "The outcomes of the competition exceeded our expectations, both in terms of the quality of the technical solutions submitted and in the massive numbers of entries for the challenge, which rivaled participation in open machine learning competitions with large monetary prizes."
A dedicated session is being held today at EPSC-DPS 2019 to present the methodologies used by the winning teams to the exoplanet research community, in order to share advancements in computational statistics and machine learning. The five top-ranked teams have additionally been invited to present their solutions at the European Conference on Machine Learning (ECML-PKDD 2019) on Friday. The participation in both conferences aims to develop closer collaborations between exoplanet researchers and the machine learning and statistics communities.
ARIEL has also launched the "ExoClock" project to collect measurements known as "light curves" that show the drop in intensity as a planet transits in front of its host star and blocks some of the light. When ARIEL starts its mission to observe 1000 exoplanets in 2028, it will need to have precise knowledge of the expected transit time of each planet that it observes. Transits can be measured using small and medium-scale telescopes and give key information about the exoplanets, including their size, orbit, mass and density. ExoClock aims to enlist the sizable and active amateur astronomy community around the world to gather large numbers of light curve observations and improve the accuracy of transit timings.
"This is the first open call to join the ExoClock project and we encourage all interested observers to become part of ESA's ARIEL mission. Every transit observation is unique and important. By participating in ExoClock, citizens all over the world can contribute to the success of the ARIEL mission," said Anastasia Kokori, who announced the launch of ExoClock at EPSC-DPS 2019.
The ExoClock platform includes target prioritization and an alert systemto maximize coverage of exoplanet targets and efficient use of resources. Users are given a personalized schedule based on their telescopes and their geographical location. The lightcurves submitted will be analyzed, published and credited on ExoClock website and may become part of scientific publications.
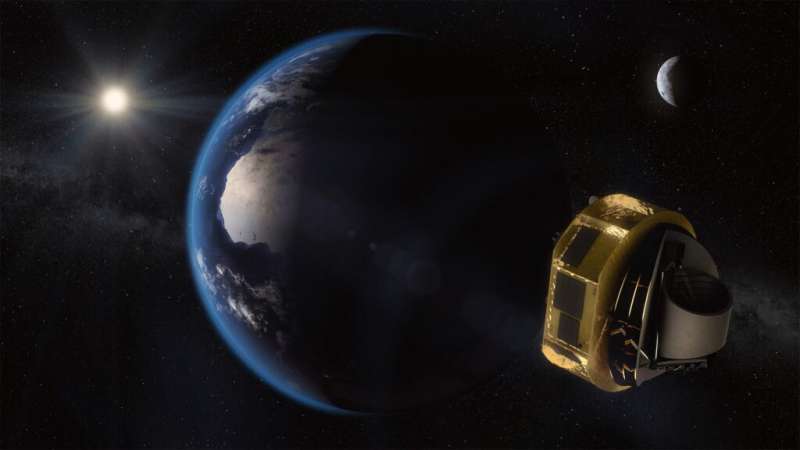
Experienced observers can register directly at exoclock.space and get started. For observers that are new to exoplanet transits, training is provided through the ExoWorlds Spies project (exoworldsspies.com). All online resources are currently available free of charge in English and in Greek.
Giovanna Tinetti, Principal Investigator for the ARIEL mission, said: "ARIEL is a challenging mission that's pushing the boundaries of exoplanet research. The Data Challenges and ExoClock project are enabling us to build a global community of collaborators with a diverse mix of skills and backgrounds. We look forward to working with them over the next few years to develop networks, tools and analysis techniques in preparation for the mission's launch in 2028."
Provided by EuroPlanet