Fruit fly brains inform search engines of the future
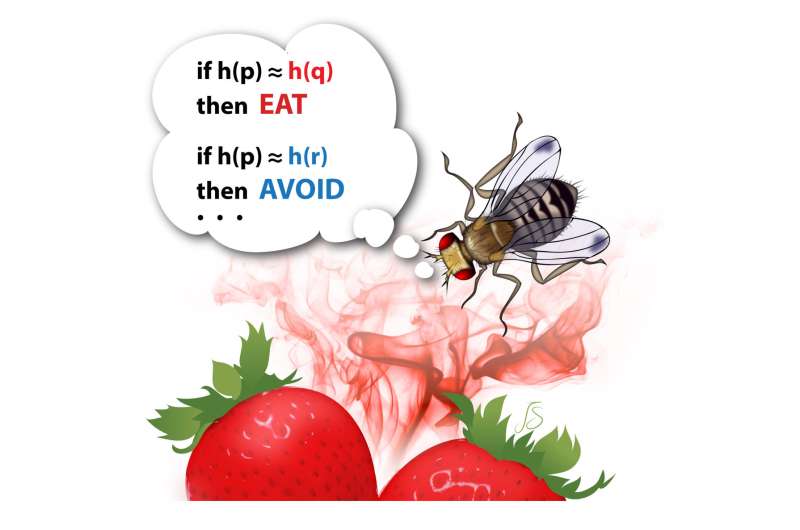
Every day, websites you visit and smartphone apps that you use are crunching huge sets of data to find things that resemble each other: products that are similar to your past purchases; songs that are similar to tunes you've liked; faces that are similar to people you've identified in photos. All these tasks are known as similarity searches, and the ability to perform these massive matching games well—and fast—has been an ongoing challenge for computer scientists.
Now, Salk and University of California San Diego scientists have discovered that the fruit fly brain has an elegant and efficient method of performing similarity searches. For flies, it helps them identify odors that are most similar to those they've encountered before, so they know how to behave in response to the odor, such as to approach or avoid it. New details on the fly's computational approach to smelly similarity searches, described in the journal Science on November 9, 2017, could inform computer algorithms of the future.
"This is a problem that pretty much every technology company with any kind of information retrieval system has to solve, so it's been something that computer scientists have studied for years," says Saket Navlakha, assistant professor in Salk's Integrative Biology Laboratory and lead author of the new paper. "Now, we have this new approach to similarity searches thanks to the fly."
The way most computerized data systems categorize items—from songs to images—to optimize similarity searches is by reducing the amount of information associated with each item. These systems assign short "hashes" to each item so that similar items are more likely to be assigned the same or a similar hash compared to two very different items. (Hashes are a kind of digital shorthand, the way a bitly is a shorter version of a URL.) Assigning hashes in this way is called "locality-sensitive hashing" to computer scientists. When searching for similar items, a program looks through the hashes, rather than the original items, to find similarities quickly.
Navlakha was chatting with colleague Charles Stevens, a professor in Salk's Molecular Neurobiology Laboratory and a coauthor of the new work, who had studied fly olfaction, when the former realized that flies—and all animals—are constantly faced with similarity searches as well. So he started combing the literature on the brain circuitry behind fly olfaction to work out just how flies identify similar smells.
"In the natural world, you're not going to encounter exactly the same odor every time; there's going to be some noise and fluctuation," Navlakha explains. "But if you smell something that you've previously associated with a behavior, you need to be able to identify that similarity and recall that behavior." So if a fruit fly knows that the smell of a rotting banana means mealtime, it needs to respond the same way when it encounters a very similar smell, even if it never experienced that exact smell before.
Navlakha and his collaborators' review of the literature revealed that when fruit flies first sense an odor, 50 neurons fire in a combination that's unique to that smell. But rather than hashing that information by reducing the number of hashes associated with the odor, as computer programs would, flies do the opposite—they expand the dimension. The 50 initial neurons lead to 2,000 neurons, spreading out the input so that each smell has an even more distinct fingerprint among those 2,000 neurons. The brain then stores only the 5 percent of those 2,000 neurons with the top activity as the "hash" for that odor. The whole paradigm helps the brain notice similarities better than it would compared to reducing the dimension, Navlakha says.
"Say you have a bunch of people clustered by their relationships, and they're bunched into a crowded room," he explains. "Then take the same people and relationships, but have them spread out on a football field. It will be much easier to see the structure of relationships and draw boundaries between groups in the expanded space relative to the crowded space."
While Navlakha and his collaborators did not reveal the actual mechanism by which flies are storing odor information—that was already available in the literature—they are the first to analyze how this process maximizes speed and efficiency for similarity searches. When they applied the process to three standard datasets computer scientists use to test search algorithms, they found that the fly approach improved performance. This approach, they think, may inform computer programs someday.
"Pieces of this approach had been used in the past by computer scientists, but evolution put it together in a very unique way," says Navlakha.
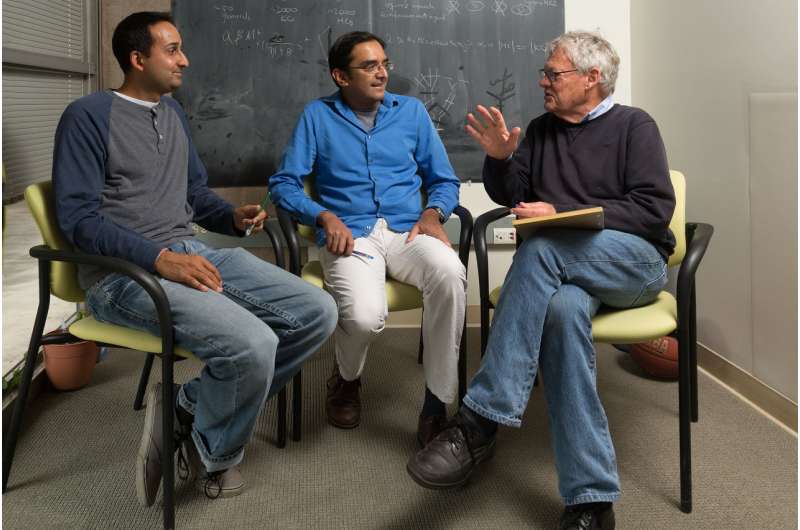
Navlakha's collaborators say that the study is among the first to make such concrete parallels between neural circuits in the brain and information processing algorithms used in computer science.
"For the past 20 years I've been interested in random projections [a core component of locality-sensitive hashing for similarity search] as they apply to algorithms running on computers," says Sanjoy Dasgupta, a professor of computer science and engineering at the UCSD Jacobs School of Engineering and first author of the new paper. "It never occurred to me that similar operations may be at work in nature."
"A dream shared by neurobiologists and computer scientists is to understand how the brain computes well enough that we can adapt its methods to improve machine computation," adds Stevens. "Our paper provides a proof of principle that this dream may become reality."
More information: S. Dasgupta at University of California, San Diego in La Jolla, CA el al., "A neural algorithm for a fundamental computing problem," Science (2017). science.sciencemag.org/cgi/doi … 1126/science.aam9868
Journal information: Science
Provided by Salk Institute