Bioengineers developing better models of the bone, muscles and nerves
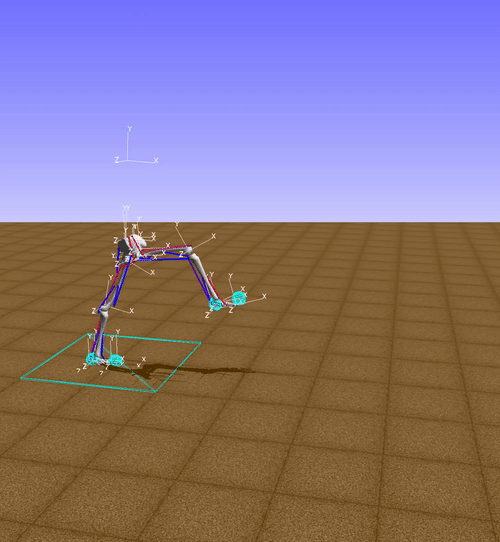
At this moment, computer-generated skeletons are competing in a virtual race, running, hopping and jumping as far as they can before collapsing in an electronic heap. Meanwhile, in the real world, their coaches – teams of machine learning and artificial intelligence enthusiasts – are competing to see who can best train their skeletons to mimic those complex human movements. Perhaps the coaches are doing it for glory or prizes or fun, but the event's creator has a serious end goal: making life better for kids with cerebral palsy.
Łukasz Kidziński, a postdoctoral fellow in bioengineering, dreamed up the contest as a way to better understand how people with cerebral palsy will respond to muscle-relaxing surgery. Often, doctors resort to surgery to improve a patient's gait, but it doesn't always work.
"The key question is how to predict how patients will walk after surgery," said Kidziński. "That's a big question, which is extremely difficult to approach."
Modeling the walk
Kidziński works in the lab of Scott Delp, a professor of bioengineering and of mechanical engineering who has spent decades studying the mechanics of the human body. As part of that work, Delp and his collaborators have collected data on the movements and muscle activity of hundreds of individuals as they walk and run.
With data like that, Delp, Kidziński and their team can build accurate models of how individual muscles and limbs move in response to signals from the brain.
But what they could not do was predict how people relearn to walk after surgery – because, as it turns out, no one is quite sure how the brain controls complex processes like walking, let alone walking through the obstacle course of daily life or relearning how to walk after surgery.
"Whereas we've gotten quite good at building computational models of muscles and joints and bones and how the whole system is connected – how the human machine is built – an open challenge is how your brain orchestrates and controls this complex dynamic system," Delp said.
Machine learning, a variety of artificial intelligence, has reached a point where it could be a useful tool for modeling of the brain's movement control systems, Delp said, but for the most part its practitioners have been interested in self-driving cars, playing complex games like chess or serving up more effective online ads.
"The time was right for a challenge like this," Delp said, in part because some in the machine learning community are looking for more meaningful problems to work on, and because bioengineers stand to gain from understanding more about machine learning. His lab's most successful efforts to model human movement have come from efforts to represent neural control of movement, Delp said, and machine learning is likely a realistic way to think about learning to walk.
The contest
So far, 63 teams have submitted a total of 145 ideas to Kidziński's competition, which is one of five similar contests created for the 2017 Neural Information Processing Systems conference. Kidziński supplies each team with computer models of the human body and the world that body must navigate, including stairs, slippery surfaces and more. In addition to external challenges, teams also face internal ones, such as weak or unreliable muscles. Each team is judged based on how far its simulated human makes it through those obstacles in a fixed amount of time.
Kidziński and Delp hope that more teams will join their competition, and with about two months remaining, they hope that at least a few teams will overcome all the various virtual obstacles thrown in their way. (No one has done so yet – the top teams have for the most part conquered walking, but none has attempted the more athletic maneuvers.) The challenge, Kidziński said, is "very computationally expensive."
In the long run, Kidziński said he hopes the work may benefit more than just kids with cerebral palsy. For example, it may help others design better-calibrated devices to assist with walking or carrying loads, and similar ideas could be used to find better baseball pitches or sprinting techniques.
But, Kidziński said, he and his collaborators have already created something important: a new way of solving problems in biomechanics that looks to virtual crowds for solutions.
Provided by Stanford University