New algorithm provides better predictions of individual preferences
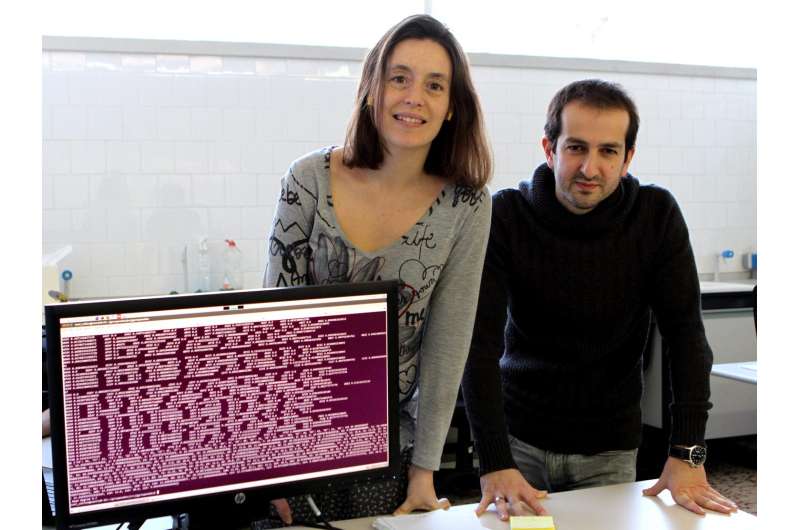
A collaborative of researchers has developed a filtering model with an associated scalable algorithm that makes accurate predictions of individuals' preferences. The new approach is based on the explicit assumption that there are groups of individuals and of items, and that the preferences of an individual for an item are determined only by their group memberships.
The new tool allows each individual and each item to belong simultaneously to mixtures of different groups, and unlike many popular approaches, it does not assume implicitly or explicitly that the individuals in each group prefer items in a single group of items. The algorithm can predict the resulting overlapping groups and preferences because it is able to predict individual preferences in large datasets, and is thus considerably more accurate than the algorithms currently used.
Algorithms are often based on unrealistic models. They mostly classify people into groups according to their preferences and make predictions on the basis of the group's behaviour. Consequently, the predictions reflect the overall preferences of the group but cannot predict the behaviour of individuals because they do not take individual differences into account. These models are therefore unable to reproduce behavioural models of the population.
The new approach is based on a more sophisticated model and better reflects how people really behave. As such, in contrast to existing models, it is more flexible and can reproduce the behavioural patterns of an entire population. It was already known that the model could provide better predictions but up to now it has always been too slow to apply to large datasets. In a scientific article published in the journal Proceedings of the National Academy of Sciences of the United States of America, the URV researchers state that they have achieved the best of both worlds—a model that is quick and scalable that also better reflects the decisions that people make.
More information: Antonia Godoy-Lorite et al, Accurate and scalable social recommendation using mixed-membership stochastic block models, Proceedings of the National Academy of Sciences (2016). DOI: 10.1073/pnas.1606316113
Journal information: Proceedings of the National Academy of Sciences
Provided by Universitat Rovira i Virgili