March 14, 2016 report
Researchers develop a family tree for better prediction of influenza mutations
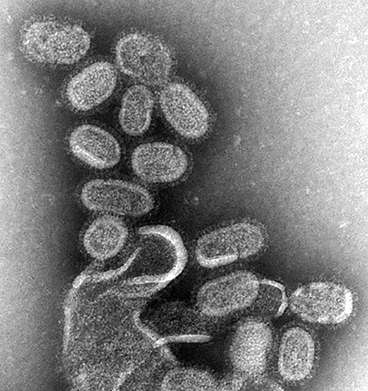
(Phys.org)—Once exposed to the influenza virus, humans mount an immune response that can be recalled by the adaptive immune system when they are exposed a second time. However, the virus mutates rapidly, and individuals can suffer from recurrent infections as a result. One of the problems health officials confront when trying to control influenza outbreaks is predicting the genetic mutations of existing strains from year to year.
By changing its surface glycoproteins, the flu virus can evade antibodies to its own genetic ancestors, and currently, the World Health Organization Global Influenza Surveillance and Response System (GISRS) directs vaccine production by assessing the antigenic phenotypes of circulating viruses. An international collaborative of researchers recently developed and tested a new technique for visualizing viral antigenic changes by mapping them along a path connecting the viruses in a phylogenetic tree.
This mapping allows allows the prediction of antigenicity by looking at a single surface glycoprotein, hemagglutinin (HA). The technique differs from the one used by the GISRS, which tracks genotypes and antigenic properties of circulating viruses. The researchers have published their results recently in the Proceedings of the National Academy of Sciences.
The new model, based on the phylogenetic tree structure of HA sequences, accurately describes the antigenic properties of seasonal influenza viruses. To develop the model, the researchers isolated A and B viruses from the Global Initiative on Sharing Avian Influenza Data (GISAID).
The model accounts for hemagglutination inhibition (HI) titers—the minimal antiserum concentration necessary to prevent the cross-linking of red blood cells by a standardized amount of virus—data that they collected from the WHO. The researchers then developed a data processing pipeline that subsampled the viruses, aligned the sequences, and built a phylogenetic tree. The model shows that HI titers have a symmetrical and treelike structure that is useful as a measure of antigenic distance between viruses.
Researchers have long preferred two-dimensional cartographic analyses of influenza mutations along the parameters of viruses and antisera. The addition of other dimensions did not improve predictive power. The new model, a tree structure, effectively has infinite dimensions, yet it predicts titers with similar or better accuracy than previous models, sharply contradicting the beliefs of medical researchers regarding the mapping of influenza mutations.
The authors note that using HI data should improve prediction accuracy, but is not a straightforward proposition; additionally, a high frequency of "false positives" tends to interfere with the us of HI measurements to detect emerging strains early enough for vaccine development. They write, "The main challenge is hence to find a way to reduce the false positive rate in HI-based prediction."
"In conclusion," they add, "our study demonstrates that HI data integrate naturally onto the sequence-derived phylogeny of the virus. Although, at present, HI-based prediction does not outperform sequence-based methods, better understanding of genetic context dependence of HI data may provide a path toward improved performance."
More information: Prediction, dynamics, and visualization of antigenic phenotypes of seasonal influenza viruses. PNAS 2016 ; published ahead of print March 7, 2016, DOI: 10.1073/pnas.1525578113
Abstract
Human seasonal influenza viruses evolve rapidly, enabling the virus population to evade immunity and reinfect previously infected individuals. Antigenic properties are largely determined by the surface glycoprotein hemagglutinin (HA), and amino acid substitutions at exposed epitope sites in HA mediate loss of recognition by antibodies. Here, we show that antigenic differences measured through serological assay data are well described by a sum of antigenic changes along the path connecting viruses in a phylogenetic tree. This mapping onto the tree allows prediction of antigenicity from HA sequence data alone. The mapping can further be used to make predictions about the makeup of the future A(H3N2) seasonal influenza virus population, and we compare predictions between models with serological and sequence data. To make timely model output readily available, we developed a web browser-based application that visualizes antigenic data on a continuously updated phylogeny.
Journal information: Proceedings of the National Academy of Sciences
© 2016 Phys.org