March 28, 2013 weblog
Scientists use AI cobweb analysis to determine spider species
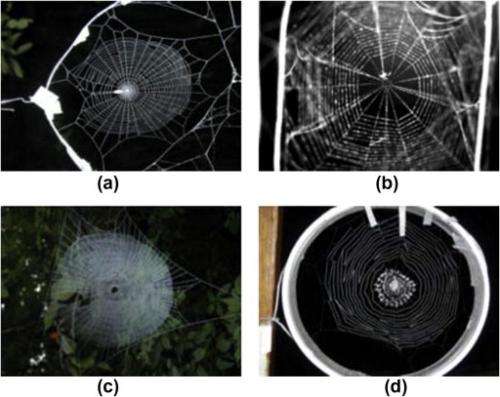
(Phys.org) —The identification of spider species based on pattern recognition of their cobwebs has been shown to be quite possible and successful. The paper, "Spider specie identification and verification based on pattern recognition of it cobweb," was published in Expert Systems and Applications and the paper's research was also recently discussed in New Scientist. A team came up with an AI cobweb recognition system through the use of special software for analyzing images supplied by a spider expert. More specifically the team used images from William Eberhard of the Smithsonian Tropical Research Institute in Costa Rica.
Carlos Travieso at the University of Las Palmas de Gran Canaria in Spain and colleagues applied their pattern recognition techniques on the pictures until they were confident they had a reliable means of identifying which species spun which type of web. According to New Scientist, they were able to achieve "astonishing" accuracy in species recognition, hitting 99.6 per cent, Their method involved principal component analysis (PCA), independent component analysis (ICA), Discrete Cosine Transform (DCT), Wavelet Transform (DWT) and discriminative common vectors such as features extractors, according to the study.
Interestingly, PCA provided the best performance, reaching a 99.65% ± 0.21 of success rate in identification mode and 99.98% ± 0.04 of the area under Receiver Operating Characteristic (ROC) curve in verification mode; the best combination of features extractors was PCA, DCT, DWT and ICA, which achieved a 99.96% ± 0.16 of success rate in identification mode and perfect verification.
The value of their research tool extends from a way of measuring spider species to understanding and measuring biodiversity. In a previous discussion on spider classification last year, Travieso and colleagues noted the great effort under way in the scientific community to study biodiversity on the planet, yet spiders were one of most unattended groups in biology.
They said the arachnids are not only plentiful but they are also ecologically crucial in almost every terrestrial and semi-terrestrial habitat. Spider webs, meanwhile, carry an incredible amount of information, they added, which can be used for the automatic classification of spider species. The researchers are now aiming to count other species of animals and insects.
More information: Spider specie identification and verification based on pattern recognition of it cobweb, Expert Systems with Applications, Volume 40, Issue 10, August 2013, Pages 4213–4225. dx.doi.org/10.1016/j.eswa.2013.01.024
Abstract
Biodiversity conservation is a global priority where the study of every type of living form is a fundamental task. Inside the huge number of the planet species, spiders play an important role in almost every habitat. This paper presents a comprehensive study on the reliability of the most used features extractors to face the problem of spider specie recognition by using their cobwebs, both in identification and verification modes. We have applied a preprocessing to the cobwebs images in order to obtain only the valid information and compute the optimal size to reach the highest performance. We have used the principal component analysis (PCA), independent component analysis (ICA), Discrete Cosine Transform (DCT), Wavelet Transform (DWT) and discriminative common vectors as features extractors, and proposed the fusion of several of them to improve the system's performance. Finally, we have used the Least Square Vector Support Machine with radial basis function as a classifier. We have implemented K-Fold and Hold-Out cross-validation techniques in order to obtain reliable results. PCA provided the best performance, reaching a 99.65% ± 0.21 of success rate in identification mode and 99.98% ± 0.04 of the area under de Reveicer Operating Characteristic (ROC) curve in verification mode. The best combination of features extractors was PCA, DCT, DWT and ICA, which achieved a 99.96% ± 0.16 of success rate in identification mode and perfect verification.
via Newscientist
© 2013 Phys.org