Leveraging machine learning to rapidly discover novel beneficial microbes
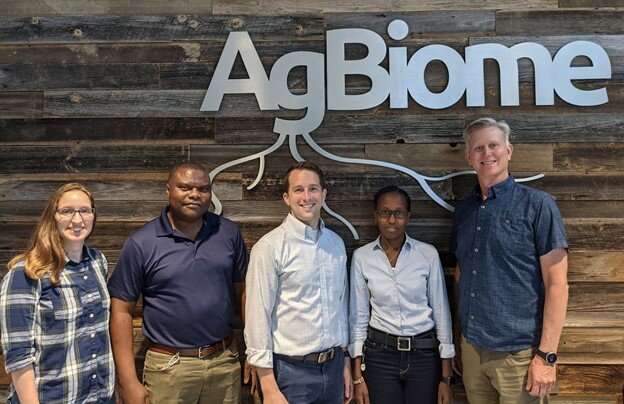
When you think about agriculture, what comes to mind? Tractors? Fields of corn? Big red barns? Often we don't think of computers. But computers and technology are playing a huge role in making our food system more sustainable and reliable. In the past few decades, high-tech machinery and robotics have changed the agroindustry. High-tech farming is making our crops more resilient against pathogens, harvest times more precise, and food yields more robust. Next-generation sequencing and machine learning now make high-tech advances possible at the genome level, particularly when untangling plant-microbe interactions. As technology advances, we can leverage these tools to promote sustainable agricultural practices.
Bacteria, fungi, and other microbes assist the growth, development, and protection of plants in a plethora of ways researchers are only beginning to understand. One of the better known microbe protection mechanisms is through microbe-microbe antagonism. Some microbes naturally produce compounds that kill other microbes. We call these compounds antibiotics or antifungals. Harnessing the compounds that harm plant pathogens can create a natural microbial product that can protect crops. However, understanding which of the thousands of microbes associated with a plant are providing such benefits to the host is challenging. How can you know which one(s) can provide beneficial qualities? This was the question addressed by Matthew Biggs in his recent Phytobiomes Journal publication. His article, "Genomics- and Machine Learning-Accelerated Discovery of Biocontrol Bacteria," uses machine learning to rapidly discover bacterial isolates with antifungal properties.
In the past, this task could take years. Researchers would take each microbe and test it for antifungal properties one at a time using chemical assays in the laboratory. This would consume a copious amount of resources and tie up a researcher for years. With the machine learning tactic proposed by Matthew Biggs and colleagues, this task is more attainable. DNA from thousands of microbes can be analyzed at once and interesting candidate microbes can be identified, quickly diminishing the pool of microbes from thousands to less than a hundred.
Machine learning uses previously known data to predict new data. It's an adaptive form of artificial intelligence that becomes more accurate over time. Dr. Biggs and his team tested and compared four different computational models. Each model was trained on a dataset before predicting bacterial function. In Biggs' case, they looked for bacteria that could prevent fungal diseases. Dr. Biggs said, "The exciting thing is that the model doesn't have to be perfect. As long as the model performs better than random, then it is useful. Our method allows us to be more efficient and effective."
A fundamental aspect of this work is the use of biosynthetic gene clusters or BGCs. BGCs are genes that are found next to each other in the genome and often work together to create a product, such as an antifungal compound. There are several known antifungal compounds such as fengycin, pyrrolnitrin, zwittermicin, or bacilysin. But there are also thousands of compounds produced by microbes that haven't been discovered yet. The computer's job is to draw connections between BGCs and pull out the BGCs that potentially have antifungal properties.
In the paper, the authors began with 1227 genomes. The machine learning algorithm flagged 72 bacteria to research further. Several of these taxa were not previously associated with antifungal activity. In addition, novel BGCs, not previously associated with antifungal activity, were discovered. In traditional screening tactics, researchers look for a known quality. They may have an assay that changes color if the microbe creates a known antifungal compound such as zwittermicin and an entirely different assay to see if the microbe produces fengycin (i.e. every microbe would have to be tested for every known antifungal compound). The novel taxa and genes, found in Bigg's research, may have been lost using these traditional tactics. With machine learning researchers can move past this barrier and find novel genes and taxa that have promising antifungal qualities. They are not limited to what is already known but with machine learning have the ability to discover new antifungal properties.
Machine learning tactics can improve the quality and development of crop protection solutions, which in turn can save time and resources. The machine learning tactic in this paper is also flexible and can be applied to other kinds of data such as finding novel microbes that can help break down plastics or new BGCs in beneficial microbes of the human gut. "Think of this as one possible application of machine learning to bacterial discovery. Let it tickle your imagination and inspire ideas for next steps in your own work," explained Matthew Biggs.
Matthew Biggs is a computational biologist at AgBiome and the lead author of this research paper. Co-authors Mathias Twizeyimana and Esther Gachango both serve as plant pathologists at Agbiome. Kelly Craig is the microbiologist on the project, and David Ingham is the product development manager. The research team will continue their work by testing the biological significance of the bacterial isolates found through their machine learning research. They will test these bacteria in field settings through a collaboration with researchers in Africa, Central America, and the U.S.
More information: Matthew B. Biggs et al, Genomics- and Machine Learning-Accelerated Discovery of Biocontrol Bacteria, Phytobiomes Journal (2021). DOI: 10.1094/PBIOMES-01-21-0003-R
Provided by American Phytopathological Society