Researchers develop automated technologies to analyze causes and effects that drive complicated systems
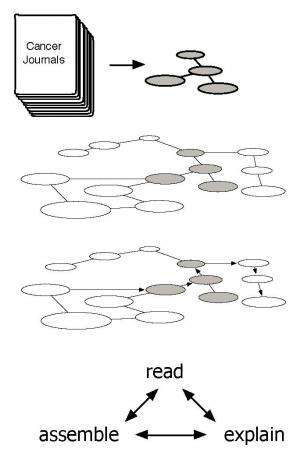
During the 1854 cholera epidemic in London, Dr. John Snow plotted cholera deaths on a map, and in the corner of a particularly hard-hit quadrangle of buildings was a water pump. Snow's maps, a 19th-century version of big data, suggested an association between cholera and the pump, but the germ theory of disease had not yet been invented and it took human ingenuity to realize that the pump was a causal mechanism of disease transmission.
Nearly two centuries on, big data is vastly bigger, but human ingenuity is still required to leap from associations to causal mechanisms. DARPA's new Big Mechanism program aims to change that.
"Having big data about complicated economic, biological, neural and climate systems isn't the same as understanding the dense webs of causes and effects—what we call the big mechanisms—in these systems," said Paul Cohen, DARPA program manager. "Unfortunately, what we know about big mechanisms is contained in enormous, fragmentary and sometimes contradictory literatures and databases, so no single human can understand a really complicated system in its entirety. Computers must help us."
The first challenge the Big Mechanism program intends to address is cancer pathways, the molecular interactions that cause cells to become and remain cancerous. The program has three primary technical areas: Computers should read abstracts and papers in cancer biology to extract fragments of cancer pathways. Next, they should assemble these fragments into complete pathways of unprecedented scale and accuracy, and should figure out how pathways interact. Finally, computers should determine the causes and effects that might be manipulated, perhaps even to prevent or control cancer.
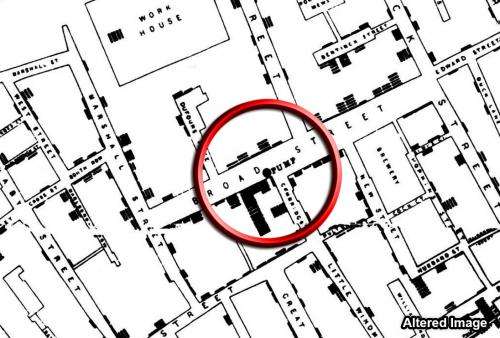
None of this is easy, but cancer biology is a logical place to start, and not only because of its obvious importance. "The language of molecular biology and the cancer literature emphasizes mechanisms," Cohen said. "Papers describe how proteins affect the expression of other proteins, and how these effects have biological consequences. Computers should be able to identify causes and effects in cancer biology papers more easily than in, say, the literatures of sociology or economics."
Assembling big mechanisms after reading about small fragments of pathways might be an even greater challenge. Inconsistent naming, experimental variability, the many kinds of cancer, and the changes cancer cells undergo as they progress through different stages make assembling a causal model of even one cancer, in one species, from fragmentary results extremely hard. But as a model emerges the Big Mechanism enterprise would, theoretically, get easier.
"The beautiful thing about causal models is that they make predictions, so we can return to our big data and see whether we're (retrospectively) right," Cohen said. "And we can propose new experiments, suggest interventions and advance our knowledge more rapidly."
Indeed, the Big Mechanism program might herald new ways to understand complicated systems. Today's researchers read deeply but struggle to keep up with relentless streams of relevant publications. To stay current, a researcher must specialize, becoming expert in a small part of something much bigger. The vision for the Big Mechanism program is fundamentally different: Every publication would immediately become part of a public, computer-maintained, causal model of a complicated system—a big mechanism—and every aspect of a big mechanism would be tied to the data that supports it or contradicts it.
"Causal models are needed to predict how systems will respond to interventions—how a patient or an economy will respond to a drug or a new tax—and to understand why systems behave as they do," Cohen said. "By emphasizing causal models and explanation, Big Mechanism may be the future of science."
Provided by DARPA